“Death by 1,000 Pilots”
Most companies find that the biggest challenge to AI is taking a promising experiment, demo, or proof-of-concept and bringing it to market. McKinsey Digital Analyst Rodney Zemmel sums this up: It’s “so easy to fire up a pilot that you can get stuck in this ‘death by 1,000 pilots’ approach.” It’s easy to see AI’s […]
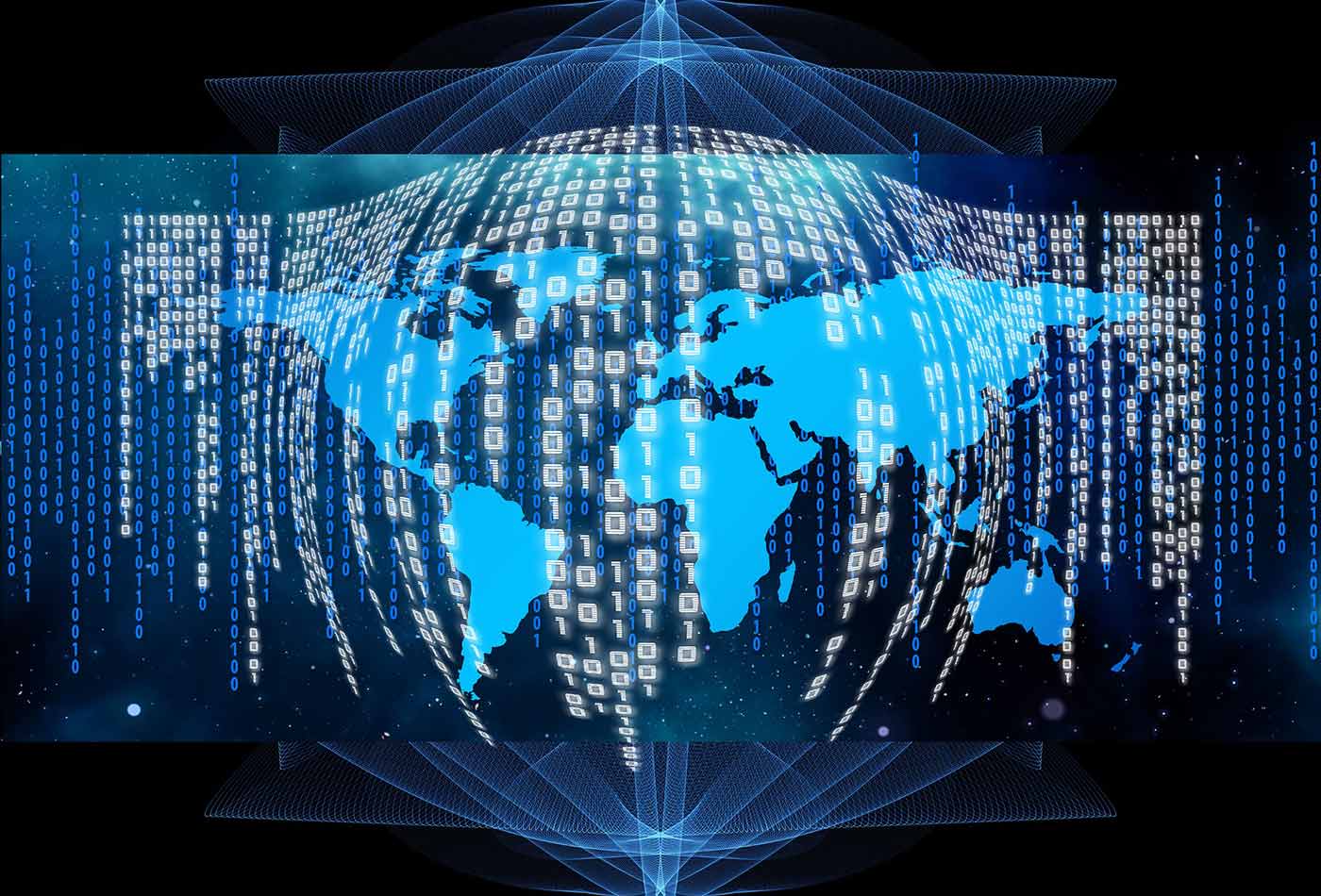
Most companies find that the biggest challenge to AI is taking a promising experiment, demo, or proof-of-concept and bringing it to market. McKinsey Digital Analyst Rodney Zemmel sums this up: It’s “so easy to fire up a pilot that you can get stuck in this ‘death by 1,000 pilots’ approach.” It’s easy to see AI’s potential, come up with some ideas, and spin up dozens (if not thousands) of pilot projects. However, the issue isn’t just the number of pilots; it’s also the difficulty of getting a pilot into production, something called “proof of concept purgatory” by Hugo Bowne-Anderson, and also discussed by Chip Huyen, Hamel Husain, and many other O’Reilly authors. Our work focuses on the challenges that come with bringing PoCs to production, such as scaling AI infrastructure, improving AI system reliability, and producing business value.
Bringing products to production includes keeping them up to date with the newest technologies for building agentic AI systems, RAG, GraphRAG, and MCP. We’re also following the development of reasoning models such as DeepSeek R1, Alibaba’s QwQ, Open AI’s 4o1 and 4o3, Google’s Gemini 2, and a growing number of other models. These models increase their accuracy by planning how to solve problems in advance.
Developers also have to consider whether to use APIs from the major providers like Open AI, Anthropic, and Google or rely on open models, including Google’s Gemma, Meta’s Llama, DeepSeek’s R1, and the many small language models that are derived (or “distilled”) from larger models. Many of these smaller models can run locally, without GPUs; some can run on limited hardware, like cell phones. The ability to run models locally gives AI developers options that didn’t exist a year or two ago. We are helping developers understand how to put those options to use.
A final development is a change in the way software developers write code. Programmers increasingly rely on AI assistants to write code, and are also using AI for testing and debugging. Far from being the “end of programming,” this development means that software developers will become more efficient, able to develop more software for tasks that we haven’t yet automated and tasks we haven’t yet even imagined. The term “vibe coding” has captured the popular imagination, but using AI assistants appropriately requires discipline–and we’re only now understanding what that “discipline” means. As Steve Yegge says, you have to demand that the AI writes code that meets your quality standards as an engineer.
AI assisted coding is only the tip of the iceberg, though. O’Reilly author Phillip Carter points out that LLMs and traditional software are good at different things. Understanding how to meld the two into an effective application requires a new approach to software architecture, debugging and ‘evals’, downstream monitoring and observability, and operations at scale. The internet’s dominant services have built using systems that provide rich feedback loops and accumulating data; these systems of control and optimization will necessarily be different as AI takes center stage.
The challenge of achieving AI’s full potential is not just true for programming. AI is changing content creation, design, marketing, sales, corporate learning, and even internal management processes; the challenge will be building effective tools with AI, and both employees and customers will need to learn to use those new tools effectively.
Helping our customers keep up with this avalanche of innovation, all the while turning exciting pilots into effective implementation: That’s our work in one sentence.