MCP vs API: Revolutionizing AI Integration & Development
Large language models(LLMs) have completely changed the way we interact with information and technology, with models like ChatGPT, LlaMA, and Claude. These models have enough capabilities to conduct thorough research, resolve more tough tasks, and write effectively. On the other hand, the traditional models are limited to real-world data and functions, though they are very good at responding to generic language. Anthropic MCP (Model Context Protocol) helps in overcoming this difficulty by providing standardized methods for LLMs to interact with multiple data sources and tools. It acts as a ‘universal remote’ for AI applications. Anthropic released MCP as an open-source protocol, which helps in improving function calling by eliminating the need for special integration between LLMs and other applications. You don’t have to start from scratch for every combination of external systems and AI models; developers can create more strong, up-to-the-point context apps. This sets the stage for the mcp vs api debate, where MCP offers a new way to handle AI interactions more efficiently. Testing tools for AI-powered APIs might not work well with legacy infrastructures and older APIs. Additional customization and labor are frequently needed to adapt SOAP-based, solid, or undocumented APIs to AI-driven workflows. Traditional APIs, which were created for human-driven interactions, are unsuitable for AI-powered apps due to their static nature, limited adaptability, and difficulty managing massive AI workloads. Let’s understand more about MCP in AI development and how it provides a simpler way to integrate AI as compared to APIs, highlighting the mcp vs api contrast. ** What Do You Mean By Model Context Protocol (MCP)? ** MCP Hosts: Applications such as Claude Desktop or AI-powered IDEs that require interaction with external tools or data. MCP Clients: Components that establish direct, one-to-one links with MCP servers to facilitate communication. MCP Servers: Lightweight service layers that offer specific capabilities through MCP, bridging connections to local or remote resources. Local Data Sources: Securely accessed assets like files, databases, or local services connected via MCP servers. Remote Services: Online APIs or cloud-based platforms that MCP servers interact with to retrieve or send data. MCP works as a two-way communication link between external tools and AI assistants, enabling them to act in addition to providing access to information. It is an open-source protocol made to safely and securely link AI tools to data sources such as the development server, Slack workplace, or CRM used by your business. This implies that your AI assistant may retrieve pertinent information and initiate activities in those tools, such as sending a message, amending a record, or initiating a deployment. More practical, context-aware, and proactive AI experiences are made possible by MCP, which empowers AI assistants to both understand and act. ** Key features of MCP: ** Stateful AI interactions: AI models and external tools may interact flexibly and dynamically because of MCP's client-server design. MCP uses JSON-RPC to standardize the process of establishing these connections through a single protocol, eliminating the need to hardcode unique integrations for each service. (remembers context across sessions). Lower latency: A lightweight protocol guarantees low latency and quick, real-time communication and reduces back-and-forth requests. Self-optimizing: Works with a variety of platforms (such as AWS, Slack, and GitHub) and uses a modular design to adapt to new technologies & model behavior dynamically. Why Use MCP Over Traditional APIs? Conventional APIs are often stateless, rigid, and lack the ability to provide models with the rich, persistent context necessary for advanced reasoning and decision-making. However, MCP can supercharge AI as it is designed to support dynamic context propagation. It provides a standardized mechanism for maintaining, updating, and retrieving contextual information across interactions. Let’s understand why MCP is better than traditional APIs: Always Get The Most Recent Information MCP works with real-time data retrieval rather than pre-cached or indexed datasets that are rapidly out-of-date. This implies that AI systems are continually working with new data, which lowers the possibility of inaccurate or out-of-date answers. Increased Compliance And Security The danger of breaches and noncompliance increases when intermediary data is stored. This problem is resolved by MCP, which only retrieves data when required and does not retain extra copies. Businesses that handle sensitive data, like healthcare and banking, where regulatory compliance is crucial, would find this especially helpful. Reduced computational burden Many AI systems use vector databases and embeddings to preprocess data. This works well, but it takes a lot of resources. By allowing models to
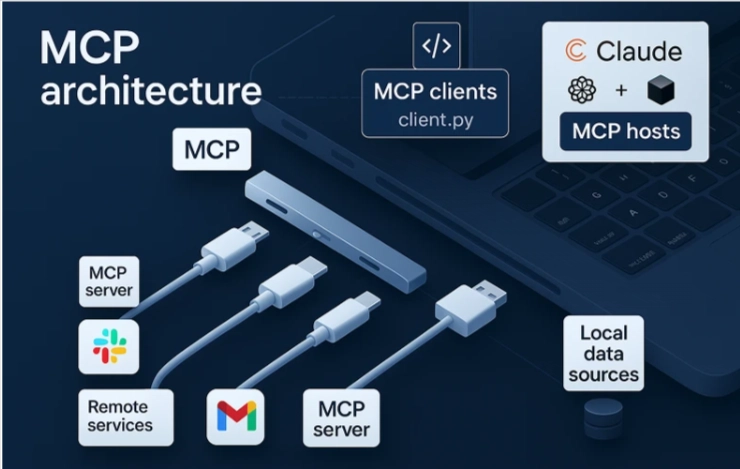
Large language models(LLMs) have completely changed the way we interact with information and technology, with models like ChatGPT, LlaMA, and Claude. These models have enough capabilities to conduct thorough research, resolve more tough tasks, and write effectively. On the other hand, the traditional models are limited to real-world data and functions, though they are very good at responding to generic language.
Anthropic MCP (Model Context Protocol) helps in overcoming this difficulty by providing standardized methods for LLMs to interact with multiple data sources and tools. It acts as a ‘universal remote’ for AI applications. Anthropic released MCP as an open-source protocol, which helps in improving function calling by eliminating the need for special integration between LLMs and other applications. You don’t have to start from scratch for every combination of external systems and AI models; developers can create more strong, up-to-the-point context apps. This sets the stage for the mcp vs api debate, where MCP offers a new way to handle AI interactions more efficiently.
Testing tools for AI-powered APIs might not work well with legacy infrastructures and older APIs. Additional customization and labor are frequently needed to adapt SOAP-based, solid, or undocumented APIs to AI-driven workflows. Traditional APIs, which were created for human-driven interactions, are unsuitable for AI-powered apps due to their static nature, limited adaptability, and difficulty managing massive AI workloads.
Let’s understand more about MCP in AI development and how it provides a simpler way to integrate AI as compared to APIs, highlighting the mcp vs api contrast.
**
What Do You Mean By Model Context Protocol (MCP)?
**
- MCP Hosts: Applications such as Claude Desktop or AI-powered IDEs that require interaction with external tools or data.
- MCP Clients: Components that establish direct, one-to-one links with MCP servers to facilitate communication.
- MCP Servers: Lightweight service layers that offer specific capabilities through MCP, bridging connections to local or remote resources.
- Local Data Sources: Securely accessed assets like files, databases, or local services connected via MCP servers.
- Remote Services: Online APIs or cloud-based platforms that MCP servers interact with to retrieve or send data.
MCP works as a two-way communication link between external tools and AI assistants, enabling them to act in addition to providing access to information.
It is an open-source protocol made to safely and securely link AI tools to data sources such as the development server, Slack workplace, or CRM used by your business. This implies that your AI assistant may retrieve pertinent information and initiate activities in those tools, such as sending a message, amending a record, or initiating a deployment. More practical, context-aware, and proactive AI experiences are made possible by MCP, which empowers AI assistants to both understand and act.
**
Key features of MCP:
**
- Stateful AI interactions: AI models and external tools may interact flexibly and dynamically because of MCP's client-server design. MCP uses JSON-RPC to standardize the process of establishing these connections through a single protocol, eliminating the need to hardcode unique integrations for each service. (remembers context across sessions).
- Lower latency: A lightweight protocol guarantees low latency and quick, real-time communication and reduces back-and-forth requests.
- Self-optimizing: Works with a variety of platforms (such as AWS, Slack, and GitHub) and uses a modular design to adapt to new technologies & model behavior dynamically.
Why Use MCP Over Traditional APIs?
Conventional APIs are often stateless, rigid, and lack the ability to provide models with the rich, persistent context necessary for advanced reasoning and decision-making. However, MCP can supercharge AI as it is designed to support dynamic context propagation. It provides a standardized mechanism for maintaining, updating, and retrieving contextual information across interactions. Let’s understand why MCP is better than traditional APIs:
Always Get The Most Recent Information
MCP works with real-time data retrieval rather than pre-cached or indexed datasets that are rapidly out-of-date. This implies that AI systems are continually working with new data, which lowers the possibility of inaccurate or out-of-date answers.
Increased Compliance And SecurityThe danger of breaches and noncompliance increases when intermediary data is stored. This problem is resolved by MCP, which only retrieves data when required and does not retain extra copies. Businesses that handle sensitive data, like healthcare and banking, where regulatory compliance is crucial, would find this especially helpful.
Reduced computational burdenMany AI systems use vector databases and embeddings to preprocess data. This works well, but it takes a lot of resources. By allowing models to request only the data they need in real time, MCP reduces this load while enhancing performance and lowering computation costs.
**Scales without further effort
- Traditional methods increase complexity by requiring specially designed connectors for various platforms. Without requiring additional development work, MCP's standard protocol enables AI models to interface with a variety of applications. Scaling across various AI workflows is made simpler as a result.
Makes development and maintenance easier
- Developers can eliminate the requirement to maintain distinct API connectors for each external system by using MCP. This expedites development and lessens maintenance hassles because API upgrades or modifications won't interfere with integrations. More contextually aware and flexible AI
- MCP facilitates the dynamic discovery of new data sources and the environmental adaptation of AI models. As a result, AI systems can continue to adapt to changing requirements without requiring frequent reconfiguration.
Read The Full Blog:- [https://www.bitontree.com/blog/model-context-protocol-vs-api](https://www.bitontree.com/blog/model-context-protocol-vs-api
)