The Great AI Agent Protocol Race: Function Calling vs. MCP vs. A2A
If you’ve been keeping an eye on the AI dev world lately, you’ve probably noticed something: everyone is now talking about AI Agents — not just smart chatbots, but full-blown autonomous programs that can use tools, call APIs, and even collaborate with each other. LangChain and OpenAI even had a debate over the definition of “AI Agents.” But as soon as you start building serious AI Agent systems, one big headache hits you: there’s no clear, universal way for Agents to work with tools — or with each other. Right now, three major approaches are competing to define the future of AI agent architecture: Function Calling: OpenAI's pioneering approach — teaching LLMs to make API calls like junior developers MCP (Model Context Protocol): Anthropic’s attempt to create a standard toolkit interface across models and services. A2A (Agent-to-Agent Protocol): Google’s brand-new spec for letting different Agents talk to each other and work as a team. Every major AI player — OpenAI, Anthropic, Google — is quietly betting that whoever defines these standards will shape the future agent ecosystem. For developers building beyond basic chatbots, understanding these protocols isn't just about keeping up — it's about avoiding painful rewrites down the road. Here's what we'll cover in his post: What is Function Calling Why it made tool use possible — but why it’s not enough. How MCP tries to fix the mess by creating a real protocol for tools and models. What A2A adds by making Agents work together like teams, not loners. How you should actually think about using them (without wasting time chasing hype). Function Calling: The Pioneer with Growing Pains Function Calling, popularized by OpenAI and now adopted by Meta, Google, and others, was the first mainstream approach to connecting LLMs with external tools. Think of it as teaching your LLM to write API calls based on natural language requests. Figure 1: Function calling workflow (Credit @Google Cloud) The workflow is straightforward: User asks a question ("What's the weather in Seattle?") LLM recognizes it needs external data It selects the appropriate function from your predefined list It formats parameters following JSON Schema: 5 { "location": "Seattle", "unit": "celsius" } Your application executes the actual API call The LLM incorporates the returned data into its response For developers, Function Calling feels like giving your AI a cookbook of API recipes it can follow. For simple applications with a single model, it's nearly plug-and-play. To learn more about how to use function calling for building applications, check out the following articles: How to Use Function Calling with Ollama, Llama3 and Milvus - Zilliz blog Understanding Function Calling in LLMs - Zilliz blog But there's a significant drawback when scaling: no cross-model consistency. Each LLM provider implements function calling differently. Want to support both Claude and GPT? You'll need to maintain separate function definitions and handle different response formats. It's like having to rewrite your restaurant order in a different language for each chef in the kitchen. This M×N problem becomes unwieldy fast as you add more models and tools. Function Calling also lacks native support for multi-step function chains. If the output from one function needs to feed into another, you're handling that orchestration yourself. MCP (Model Context Protocol): The Universal Translator for AI and Tools MCP (Model Context Protocol) addresses precisely these scaling issues. Backed by Anthropic and gaining support across models like Claude, GPT, Llama, and others, MCP introduces a standardized way for LLMs to interact with external tools and data sources. How MCP Works Think of MCP as the "USB standard for AI tools" — a universal interface that ensures compatibility: Tools advertise their capabilities using a standardized format, describing available actions, required inputs, and expected outputs AI models read these descriptions and can automatically understand how to use the tools Applications integrate once and gain compatibility across the AI ecosystem MCP transforms the messy M×N integration problem into a more manageable M+N problem. The MCP Architecture MCP uses a client-server model with four key components: Figure 2: The MCP architecture (Credit @Anthropic) MCP Hosts: The applications where users interact with AI (like Claude Desktop or AI-enhanced code editors) MCP Clients: The connectors that manage communication between hosts and servers MCP Servers: Tool implementations that expose functionality through the MCP standard Data Sources: The underlying files, databases, APIs and services that provide information If Function Calling is like having to speak multiple languages to different chefs, MCP is like having a universal translator in the kitchen. Define your tools once, and any MCP-compatible model can use them wi
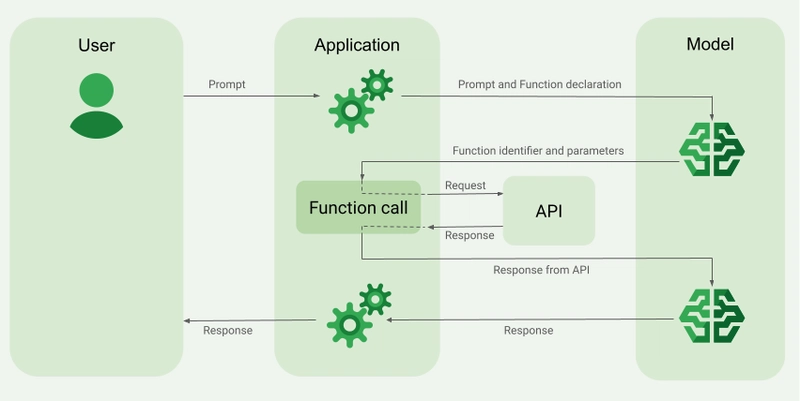
If you’ve been keeping an eye on the AI dev world lately, you’ve probably noticed something: everyone is now talking about AI Agents — not just smart chatbots, but full-blown autonomous programs that can use tools, call APIs, and even collaborate with each other. LangChain and OpenAI even had a debate over the definition of “AI Agents.”
But as soon as you start building serious AI Agent systems, one big headache hits you: there’s no clear, universal way for Agents to work with tools — or with each other.
Right now, three major approaches are competing to define the future of AI agent architecture:
Function Calling: OpenAI's pioneering approach — teaching LLMs to make API calls like junior developers
MCP (Model Context Protocol): Anthropic’s attempt to create a standard toolkit interface across models and services.
A2A (Agent-to-Agent Protocol): Google’s brand-new spec for letting different Agents talk to each other and work as a team.
Every major AI player — OpenAI, Anthropic, Google — is quietly betting that whoever defines these standards will shape the future agent ecosystem.
For developers building beyond basic chatbots, understanding these protocols isn't just about keeping up — it's about avoiding painful rewrites down the road.
Here's what we'll cover in his post:
What is Function Calling Why it made tool use possible — but why it’s not enough.
How MCP tries to fix the mess by creating a real protocol for tools and models.
What A2A adds by making Agents work together like teams, not loners.
How you should actually think about using them (without wasting time chasing hype).
Function Calling: The Pioneer with Growing Pains
Function Calling, popularized by OpenAI and now adopted by Meta, Google, and others, was the first mainstream approach to connecting LLMs with external tools. Think of it as teaching your LLM to write API calls based on natural language requests.
Figure 1: Function calling workflow (Credit @Google Cloud)
The workflow is straightforward:
User asks a question ("What's the weather in Seattle?")
LLM recognizes it needs external data
It selects the appropriate function from your predefined list
It formats parameters following JSON Schema:
5
{
"location": "Seattle",
"unit": "celsius"
}
Your application executes the actual API call
The LLM incorporates the returned data into its response
For developers, Function Calling feels like giving your AI a cookbook of API recipes it can follow. For simple applications with a single model, it's nearly plug-and-play. To learn more about how to use function calling for building applications, check out the following articles:
But there's a significant drawback when scaling: no cross-model consistency. Each LLM provider implements function calling differently. Want to support both Claude and GPT? You'll need to maintain separate function definitions and handle different response formats.
It's like having to rewrite your restaurant order in a different language for each chef in the kitchen. This M×N problem becomes unwieldy fast as you add more models and tools.
Function Calling also lacks native support for multi-step function chains. If the output from one function needs to feed into another, you're handling that orchestration yourself.
MCP (Model Context Protocol): The Universal Translator for AI and Tools
MCP (Model Context Protocol) addresses precisely these scaling issues. Backed by Anthropic and gaining support across models like Claude, GPT, Llama, and others, MCP introduces a standardized way for LLMs to interact with external tools and data sources.
How MCP Works
Think of MCP as the "USB standard for AI tools" — a universal interface that ensures compatibility:
Tools advertise their capabilities using a standardized format, describing available actions, required inputs, and expected outputs
AI models read these descriptions and can automatically understand how to use the tools
Applications integrate once and gain compatibility across the AI ecosystem
MCP transforms the messy M×N integration problem into a more manageable M+N problem.
The MCP Architecture
MCP uses a client-server model with four key components:
Figure 2: The MCP architecture (Credit @Anthropic)
MCP Hosts: The applications where users interact with AI (like Claude Desktop or AI-enhanced code editors)
MCP Clients: The connectors that manage communication between hosts and servers
MCP Servers: Tool implementations that expose functionality through the MCP standard
Data Sources: The underlying files, databases, APIs and services that provide information
If Function Calling is like having to speak multiple languages to different chefs, MCP is like having a universal translator in the kitchen. Define your tools once, and any MCP-compatible model can use them without custom code. This dramatically reduces the marginal cost of adding new models or tools to your application. As someone who's dealt with integration headaches, that's music to my ears.
A2A (Agent-to-Agent Protocol): The Team Coordinator for AI Agents
While Function Calling and MCP focus on model-to-tool interaction, A2A (Agent-to-Agent Protocol), introduced by Google, tackles a different challenge: How do we get multiple specialized agents to collaborate effectively?
As AI agent architectures grow more complex, it quickly becomes clear that no single agent should handle everything. You might have one agent specialized in document summarization, another in database queries, and another in user interaction.
A2A defines a lightweight, open protocol that lets different Agents:
Discover each other and advertise their capabilities,
Delegate tasks dynamically to the best-suited Agent,
Coordinate progress and share real-time updates securely.
Figure 3: How A2A works (credit @Google)
A2A facilitates communication between a "client" agent that manages tasks and a "remote" agent that executes them. If Function Calling gives an agent access to tools, A2A lets agents form effective teams.
Consider hiring a software engineer: A hiring manager could task their agent to find candidates matching specific criteria. This agent then collaborates with specialized agents to source candidates, schedule interviews, and facilitate background checks — all through a unified interface.
Quick Comparison: Function Calling vs MCP vs A2A
It's tempting to see these protocols as competitors, but they actually solve different pieces of the agent ecosystem puzzle:
Function Calling connects models to individual tools (limited but simple)
MCP standardizes tool access across different models (more scalable)
A2A enables collaboration between independent agents (higher-level orchestration)
Function Calling | MCP | A2A | |
---|---|---|---|
What it solves | Model → API calls | Model → Tools access, standardized | Agent → Agent collaboration |
Good for | Simple real-time queries | Scalable tool ecosystems | Distributed multi-agent workflows |
Pain points | No standard, messy multi-model support | Need to set up servers | Still early days, limited support |
Real-world analogy | Teaching your AI to make phone calls | Having any smart app access any database/API easily | Having teams of bots working together like coworkers |
In architectural terms, MCP answers "what tools can my agent use?" while A2A handles "how can my agents work together?"
This resembles how we structure complex software: individual components with well-defined interfaces, composed into larger systems. An effective agent ecosystem needs both tool interfaces (Function Calling/MCP) and inter-agent communication (A2A).
What This Means for Developers
So, what should you, as a developer building with AI, do with these competing standards?
For simple applications: Function Calling remains the quickest path to adding tool use to your LLM application, especially if you're only using one model provider.
For cross-model compatibility: Consider adopting MCP, which gives you broader model support without duplicating integration work.
For complex multi-agent systems: Keep an eye on A2A, which could become crucial as agent ecosystems mature.
The smart play might be to layer these approaches: use Function Calling for quick prototyping, but implement MCP adapters for better scalability, with A2A orchestration for multi-agent workflows.
The Road Ahead
The conversation around what makes an "AI Agent" is still evolving — sometimes even debated between companies like OpenAI, Anthropic, and LangChain.
But regardless of definitions, one thing is clear: Standards like Function Calling, MCP, and A2A are laying the foundation for the next generation of AI applications.
For developers, understanding these patterns early is an investment in future-proofing your work. It's how we move from toy demos to production-ready systems — the kind that solve real problems at scale. The agent ecosystem is developing rapidly, and building on these protocols now means positioning your applications for what's coming next.
What do you think? Which protocols are you using in your AI projects? Are you betting on one standard winning out, or preparing for a multi-protocol future?