Hussein Osman, Segment Marketing Director at Lattice Semiconductor – Interview Series
Hussein Osman is a semiconductor industry veteran with over two decades of experience bringing to market silicon and software products that integrate sensing, processing and connectivity solutions, focusing on innovative experiences that deliver value to the end user. Over the past five years he has led the sensAI solution strategy and go-to-market efforts at Lattice […] The post Hussein Osman, Segment Marketing Director at Lattice Semiconductor – Interview Series appeared first on Unite.AI.
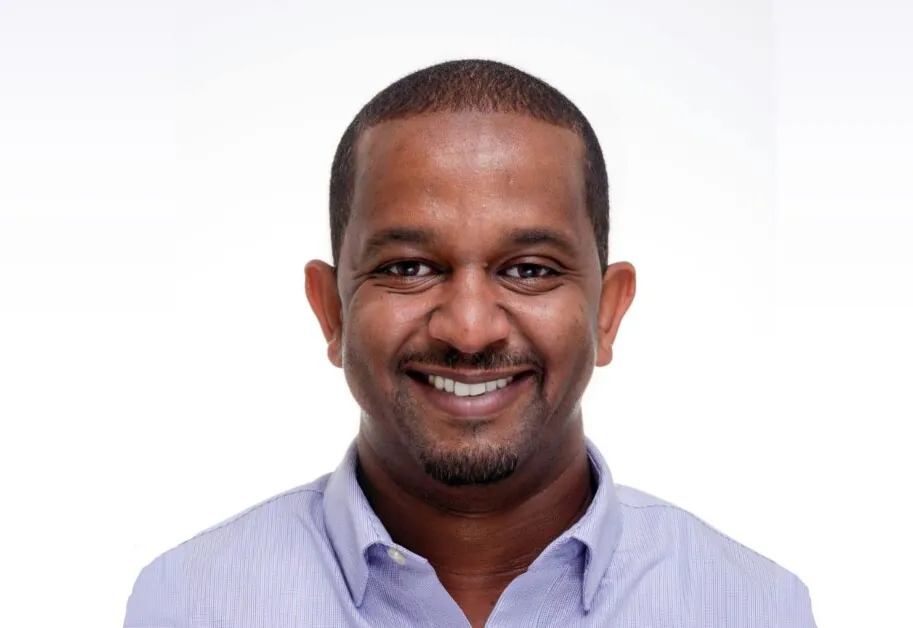
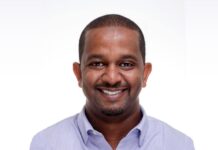
Hussein Osman is a semiconductor industry veteran with over two decades of experience bringing to market silicon and software products that integrate sensing, processing and connectivity solutions, focusing on innovative experiences that deliver value to the end user. Over the past five years he has led the sensAI solution strategy and go-to-market efforts at Lattice Semiconductor, creating high-performance AI/ML applications. Mr. Osman received his bachelor’s degree in Electrical Engineering from California Polytechnic State University in San Luis Obispo.
Lattice Semiconductor (LSCC -12.16%) is a provider of low-power programmable solutions used across communications, computing, industrial, automotive, and consumer markets. The company's low-power FPGAs and software tools are designed to help accelerate development and support innovation across applications from the Edge to the Cloud.
Edge AI is gaining traction as companies seek alternatives to cloud-based AI processing. How do you see this shift impacting the semiconductor industry, and what role does Lattice Semiconductor play in this transformation?
Edge AI is absolutely gaining traction, and it’s because of its potential to truly revolutionize entire markets. Organizations across a wide range of sectors are leaning into Edge AI because it’s helping them achieve faster, more efficient, and more secure operations — especially in real-time applications — than are possible with cloud computing alone. That’s the piece most people tend to focus on: how Edge AI is changing business operations when implemented. But there’s this other journey that’s happening in tandem, and it starts far before implementation.
Innovation in Edge AI is pushing original equipment manufacturers to design system components that can run AI models despite footprint constraints. That means lightweight, optimized algorithms, specialized hardware, and other advancements that complement and/or amplify performance. This is where Lattice Semiconductor comes into play.
Our Field Programmable Gate Arrays (FPGAs) provide the highly adaptable hardware necessary for designers to meet strict system requirements related to latency, power, security, connectivity, size, and more. They provide a foundation on which engineers can build devices capable of keeping mission-critical Automotive, Industrial, and Medical applications functional. This is a big focus area for our current innovation, and we’re excited to help customers overcome challenges and greet the era of Edge AI with confidence.
What are the key challenges that businesses face when implementing Edge AI, and how do you see FPGAs addressing these issues more effectively than traditional processors or GPUs?
You know, some challenges seem to be truly universal as any technology advances. For example, developers and businesses hoping to harness the power of Edge AI will likely grapple with common challenges, such as:
- Resource management. Edge AI devices have to perform complex processes reliably while working within increasingly limited computational and battery capacities.
- Although Edge AI offers the privacy benefits of local data processing, it raises other security concerns, such as the possibility of physical tampering or the vulnerabilities that come with smaller-scale models.
- Edge AI ecosystems can be extremely diverse in hardware architectures and computing requirements, making it difficult to streamline aspects like data management and model updates at scale.
FPGAs offer businesses a leg up in addressing these key issues through their combination of efficient parallel processing, low power consumption, hardware-level security capabilities, and reconfigurability. While these may sound like marketing buzzwords, they are essential features for solving top Edge AI pain points.
FPGAs have traditionally been used for functions like bridging and I/O expansion. What makes them particularly well-suited for Edge AI applications?
Yes, you’re exactly right that FPGAs excel in the realm of connectivity — and that’s part of what makes them so powerful in Edge AI applications. As you mentioned, they have customizable I/O ports that allow them to interface with a wide array of devices and communication protocols. On top of this, they can perform functions like bridging and sensor fusion to ensure seamless data exchange, aggregation, and synchronization between different system components, including legacy and emerging standards. These functions are particularly important as today’s Edge AI ecosystems grow more complex and the need for interoperability and scalability increases.
However, as we’ve been discussing, FPGAs’ connectivity benefits are only the tip of the iceberg; it’s also about how their adaptability, processing power, energy efficiency, and security features are driving outcomes. For example, FPGAs can be configured and reconfigured to perform specific AI tasks, enabling developers to tailor applications to their unique needs and meet evolving requirements.
Can you explain how low-power FPGAs compare to GPUs and ASICs in terms of efficiency, scalability, and real-time processing capabilities for Edge AI?
I won’t pretend that hardware like GPUs and ASICs don’t have the compute power to support Edge AI applications. They do. But FPGAs truly have an “edge” on these other components in other areas like latency and flexibility. For example, both GPUs and FPGAs can perform parallel processing, but GPU hardware is designed for broad appeal and is not as well suited to supporting specific Edge applications as that of FPGAs. On the other hand, ASICs are targeted for specific applications, but their fixed functionality means they require full redesigns to accommodate any significant change in use. FPGAs are purpose-built to provide the best of both worlds; they offer the low latency that comes with custom hardware pipelines and room for post-deployment modifications whenever Edge models need updating.
Of course, no single option is the only right one. It’s up to each developer to decide what makes sense for their system. They should carefully consider the primary functions of the application, the specific outcomes they are trying to meet, and how agile the design needs to be from a future-proofing perspective. This will allow them to choose the right set of hardware and software components to meet their requirements — we just happen to think that FPGAs are usually the right choice.
How do Lattice’s FPGAs enhance AI-driven decision-making at the edge, particularly in industries like automotive, industrial automation, and IoT?
FPGAs’ parallel processing capabilities are a good place to begin. Unlike sequential processors, the architecture of FPGAs allows them to perform many tasks in parallel, including AI computations, with all the configurable logic blocks executing different operations simultaneously. This allows for the high throughput, low latency processing needed to support real-time applications in the key verticals you named — whether we’re talking about autonomous vehicles, smart industrial robots, or even smart home devices or healthcare wearables. Moreover, they can be customized for specific AI workloads and easily reprogrammed in the field as models and requirements evolve over time. Last, but not least, they offer hardware-level security features to ensure AI-powered systems remain secure, from boot-up to data processing and beyond.
What are some real-world use cases where Lattice’s FPGAs have significantly improved Edge AI performance, security, or efficiency?
Great question! One application that I find really intriguing is the ways engineers are using Lattice FPGAs to power the next generation of smart, AI-powered robots. Intelligent robots require real-time, on-device processing capabilities to ensure safe automation, and that’s something Edge AI is designed to deliver. Not only is the demand for these assistants rising, but so is the complexity and sophistication of their functions. At a recent conference, the Lattice team demonstrated how the use of FPGAs allowed a smart robot to track the trajectory of a ball and catch it in midair, showing just how fast and precise these machines can be when built with the right technologies.
What makes this so interesting to me, from a hardware perspective, is how design tactics are changing to accommodate these applications. For example, instead of relying solely on CPUs or other traditional processors, developers are beginning to integrate FPGAs into the mix. The main benefit is that FPGAs can interface with more sensors and actuators (and a more diverse range of these components), while also performing low-level processing tasks near these sensors to free up the main compute engine for more advanced computations.
With the growing demand for AI inference at the edge, how does Lattice ensure its FPGAs remain competitive against specialized AI chips developed by larger semiconductor companies?
There’s no doubt that the pursuit of AI chips is driving much of the semiconductor industry — just look at how companies like Nvidia pivoted from creating video game graphics cards to becoming AI industry giants. Still, Lattice brings unique strengths to the table that make us stand out even as the market becomes more saturated.
FPGAs are not just a component we’re choosing to invest in because demand is rising; they’re a critical piece of our core product line. The strengths of our FPGA offerings — from latency and programmability to power consumption and scalability — are the result of years of technical development and refinement. We also provide a full range of industry-leading software and solution stacks, built to optimize the usage of FPGAs in AI designs and beyond.
We’ve refined our FPGAs through years of continuous improvement driven by iteration on our hardware and software solutions and relationships with partners across the semiconductor industry. We’ll continue to be competitive because we’ll keep true to that path, working with design, development, and implementation partners to ensure that we’re providing our customers with the most relevant and reliable technical capabilities.
What role does programmability play in FPGAs’ ability to adapt to evolving AI models and workloads?
Unlike fixed-function hardware, FPGAs can be retooled and reprogrammed post-deployment. This inherent adaptability is arguably their biggest differentiator, especially in supporting evolving AI models and workloads. Considering how dynamic the AI landscape is, developers need to be able to support algorithm updates, growing datasets, and other significant changes as they occur without worrying about constant hardware upgrades.
For example, FPGAs are already playing a pivotal role in the ongoing shift to post-quantum cryptography (PQC). As businesses brace against looming quantum threats and work to replace vulnerable encryption schemes with next-generation algorithms, they are using FPGAs to facilitate a seamless transition and ensure compliance with new PQC standards.
How do Lattice's FPGAs help businesses balance the trade-off between performance, power consumption, and cost in Edge AI deployments?
Ultimately, developers shouldn’t have to choose between performance and possibility. Yes, Edge applications are often hindered by computational limitations, power constraints, and increased latency. But with Lattice FPGAs, developers are empowered with flexible, energy efficient, and scalable hardware that’s more than capable of mitigating these challenges. Customizable I/O interfaces, for example, enable connectivity to various Edge applications while reducing complexity.
Post-deployment modification also makes it easier to adjust to support the needs of evolving models. Beyond this, preprocessing and data aggregation can occur on FPGAs, lowering the power and computational strain on Edge processors, reducing latency, and in turn lowering costs and increasing system efficiency.
How do you envision the future of AI hardware evolving in the next 5-10 years, particularly in relation to Edge AI and power-efficient processing?
Edge devices will need to be faster and more powerful to handle the computing and energy demands of the ever-more-complex AI and ML algorithms businesses need to thrive — especially as these applications become more commonplace. The capabilities of the dynamic hardware components that support Edge applications will need to adapt in tandem, becoming smaller, smarter and more integrated. FPGAs will need to expand on their existing flexibility, offering low latency and low power capabilities for higher levels of demand. With these capabilities, FPGAs will continue to help developers reprogram and reconfigure with ease to meet the needs of evolving models — be they for more sophisticated autonomous vehicles, industrial automation, smart cities, or beyond.
Thank you for the great interview, readers who wish to learn more should visit Lattice Semiconductor.
The post Hussein Osman, Segment Marketing Director at Lattice Semiconductor – Interview Series appeared first on Unite.AI.