Dimitri Masin, CEO & Co-Founder at Gradient Labs – Interview Series
Dimitri Masin is the CEO and Co-Founder of Gradient Labs, an AI startup building autonomous customer support agents specifically designed for regulated industries such as financial services. Prior to founding Gradient Labs in 2023, Masin held senior leadership roles at Monzo Bank, including Vice President of Data Science, Financial Crime, and Fraud, and previously worked […] The post Dimitri Masin, CEO & Co-Founder at Gradient Labs – Interview Series appeared first on Unite.AI.
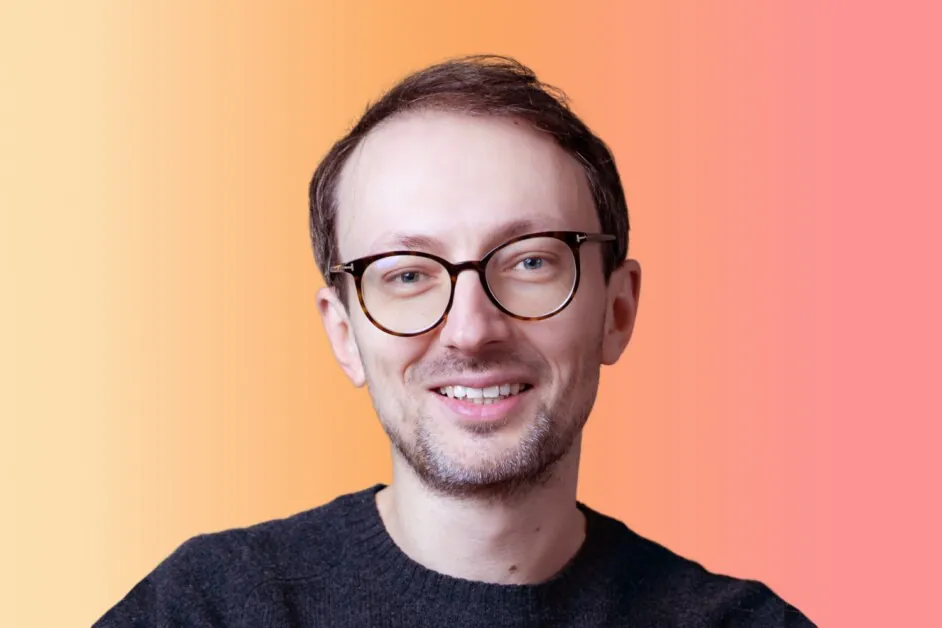

Dimitri Masin is the CEO and Co-Founder of Gradient Labs, an AI startup building autonomous customer support agents specifically designed for regulated industries such as financial services. Prior to founding Gradient Labs in 2023, Masin held senior leadership roles at Monzo Bank, including Vice President of Data Science, Financial Crime, and Fraud, and previously worked at Google. Under his leadership, Gradient Labs has quickly gained traction, reaching £1 million in annual recurring revenue within five months of launch. Masin’s focus is on developing AI systems that combine high performance with strict regulatory compliance, enabling safe and scalable automation for complex customer operations.
What inspired you to launch Gradient Labs after such a successful journey at Monzo?
At Monzo, we had spent years working on customer support automation, typically targeting modest 10% efficiency gains. But in early 2023, we witnessed a seismic technological shift with the release of GPT-4. Suddenly, it became possible to automate 70-80% of manual, repetitive work completely autonomously through AI.
This technological breakthrough we’re currently living through inspired us to start Gradient Labs. In my career, I've seen two such revolutionary waves: the mobile revolution (which happened early in my career), and now AI. When you recognize that you're in the middle of such a transformation that will completely change how the world works, you have to seize the moment. Our team knew – this is the time.
At Monzo, you helped lead the company through massive hypergrowth. What were some of the biggest lessons from that experience that you're now applying at Gradient Labs?
First, balance autonomy with direction. At Monzo, we initially assumed people simply thrive on autonomy – that it’s what motivates them most. However, that view now seems overly simplistic. I believe people also value guidance. True autonomy isn't telling people “do whatever you decide to do,” but rather providing clear direction while giving them freedom to solve well-defined problems their way.
Second, top talent requires top compensation. If you aim to hire the top 5% in your function, you must pay accordingly. Otherwise, major tech companies will hire them away once it becomes known you have top talent that's being underpaid.
Third, don't reinvent the wheel. At Monzo, we tried creating innovative approaches to work structures, compensation systems, and career ladders. The key takeaway: don't waste energy innovating on organizational fundamentals – thousands of companies have already established best practices. I still see LinkedIn posts about “getting rid of all titles and hierarchy” – I've watched this play out repeatedly, and nearly all companies eventually revert to traditional structures.
Gradient Labs is focused on regulated industries, which traditionally have complex needs. How did you approach building an AI agent (like Otto) that can operate effectively in this environment?
We took an unconventional approach, rejecting the typical advice to release quickly and iterate on a live product. Instead, we spent 14 months before releasing Otto, maintaining a very high-quality bar from the start. We needed to create something banks and financial institutions would trust to handle their support completely autonomously.
We weren't building co-pilots – we were building end-to-end automation of customer support. With our background in financial services, we had a precise internal benchmark for “what good looks like,” allowing us to assess quality without relying on customer feedback. This gave us the freedom to obsess over quality while iterating quickly. Without live customers, we could make larger leaps, break things freely, and pivot quickly – ultimately delivering a superior product at launch.
Otto goes beyond answering simple questions and handles complex workflows. Can you walk us through how Otto manages multi-step or high-risk tasks that typical AI agents might fail at?
We've built Otto around the concept of SOPs (Standard Operating Procedures) – essentially guidance documents written in plain English that detail how to handle specific issues, similar to what you'd give a human agent.
Two key architectural decisions make Otto particularly effective at managing complex workflows:
First, we limit tool exposure. A common failure mode for AI agents is choosing incorrectly from too many options. For each procedure, we expose only a small subset of relevant tools to Otto. For example, in a card replacement workflow, Otto might only see 1-2 tools instead of all 30 registered in the system. This dramatically improves accuracy by reducing the decision space.
Second, we've rebuilt much of the typical AI assistant infrastructure to enable extensive chain-of-thought reasoning. Rather than simply throwing procedures at an OpenAI or Anthropic assistant, our architecture allows for multiple processing steps between inputs and outputs. This enables deeper reasoning and more reliable outcomes.
Gradient Labs mentions achieving “superhuman quality” in customer support. What does “superhuman quality” mean to you, and how do you measure it internally?
Superhuman quality means delivering customer support measurably better than what humans can achieve. The following three examples illustrate this:
First, comprehensive knowledge. AI agents can process vast amounts of information and have detailed knowledge of a company. In contrast, humans typically only learn a small subset of information, and when they don’t know something, they must consult knowledge bases or escalate to colleagues. This leads to a frustrating experience where customers are passed between teams. An AI agent, by contrast, has a deep understanding of the company and its processes, delivering consistent, end-to-end answers – no escalation needed.
Second, non-lazy lookups – AI is quick to gather information. While humans try to save time by asking customers questions before investigating, AI proactively examines account information, flags, alerts, and error messages before the conversation begins. So, when a customer vaguely says “I have an issue with X,” the AI can immediately offer a solution instead of asking multiple clarifying questions.
Finally, patience and quality consistency. Unlike humans who face pressure to handle a certain number of replies per hour, our AI maintains consistently high quality, patience, and concise communication. It answers patiently as long as needed without rushing.
We measure this primarily through customer satisfaction scores. For all current customers, we achieve CSAT scores averaging 80%-90% – typically higher than their human teams.
You've deliberately avoided tying Gradient Labs to a single LLM provider. Why was this choice important, and how does it impact performance and reliability for your clients?
Over the past two years, we've observed that our biggest performance improvements came from our ability to switch to the next best model whenever OpenAI or Anthropic released something faster, better, or more accurate. Model agility has been key.
This flexibility allows us to continuously improve quality while managing costs. Some tasks require more powerful models, others less. Our architecture enables us to adapt and evolve over time, selecting the optimal model for each situation.
Eventually, we'll support private open-source LLMs hosted on customers' infrastructure. Because of our architecture, this will be a straightforward transition, which is especially important when serving banks that may have specific requirements about model deployment.
Gradient Labs isn't just building a chatbot — you're aiming to handle back-office processes too. What are the biggest technical or operational challenges in automating these kinds of tasks with AI?
There are two distinct categories of processes, each with its own challenges:
For simpler processes, the technology largely exists already. The main challenge is integration – connecting to the many bespoke backend systems and tools that financial institutions use, as most customer operations involve numerous internal systems.
For complex processes, significant technical challenges remain. These processes typically require humans to be hired and trained for 6-12 months to develop expertise, such as fraud investigations or money laundering assessments. The challenge here is knowledge transfer — how do we give AI agents the same domain expertise? That’s a hard problem everyone in this space is still trying to solve.
How does Gradient Labs balance the need for AI speed and efficiency with the rigorous compliance requirements of regulated industries?
It's certainly a balance, but at the conversation level, our agent simply takes more time to think. It evaluates multiple factors: Am I understanding what the customer is asking? Am I giving the correct answer? Is the customer showing vulnerability signs? Does the customer want to file a complaint?
This deliberate approach increases latency – our median response time might be 15-20 seconds. But for financial institutions, that’s a fair trade. A 15-second response is still much faster than a human reply, while the quality guarantees are vastly more important to the regulated companies we work with.
Do you foresee a future where AI agents are trusted not only for support but also for higher-stakes decision-making tasks inside financial institutions?
Financial institutions were already using more traditional AI techniques for high-stakes decisions before the current wave of generative AI. Where I see the real opportunity now is in orchestration – not making the decision, but coordinating the entire process.
For example, a customer uploads documents, an AI agent routes them to a validation system, receives confirmation of validity, and then triggers appropriate actions and customer communications. This orchestration function is where AI agents excel.
For the highest-stakes decisions themselves, I don't see much changing in the near term. These models require explainability, bias prevention, and approval through model risk committees. Large language models would face significant compliance challenges in these contexts.
In your view, how will AI reshape the customer experience for banks, fintech companies, and other regulated sectors over the next 3–5 years?
I see five major trends reshaping customer experience:
First, true omni-channel interaction. Imagine starting a chat in your banking app, then seamlessly switching to voice with the same AI agent. Voice, calls, and chat will blend into a single continuous experience.
Second, adaptive UIs that minimize navigation within the app. Rather than hunting through menus for specific functions, customers will simply voice their needs: “Please increase my limits” – and the action happens immediately through conversation.
Third, better unit economics. Support and ops are massive cost centers. Reducing these costs could let banks serve previously unprofitable customers or pass savings to users — especially in underbanked segments.
Fourth, exceptional support at scale. Currently, startups with few customers can provide personalized support, but quality typically degrades as companies grow. AI makes great support scalable, not just possible.
Finally, customer support will transform from a frustrating necessity to a genuinely helpful service. It will no longer be viewed as a labor-intensive infrastructure cost, but as a valuable, efficient customer touchpoint that enhances the overall experience.
Thank you for the great interview, readers who wish to learn more should visit Gradient Labs.
The post Dimitri Masin, CEO & Co-Founder at Gradient Labs – Interview Series appeared first on Unite.AI.