Interview with Gillian Hadfield: Normative infrastructure for AI alignment
During the 33rd International Joint Conference on Artificial Intelligence (IJCAI), held in Jeju, I had the opportunity to meet with one of the keynote speakers, Gillian Hadfield. We spoke about her interdisciplinary research, career trajectory, path into AI alignment, law, and general thoughts on AI systems. Transcript: Note: the transcript has been lightly edited for […]
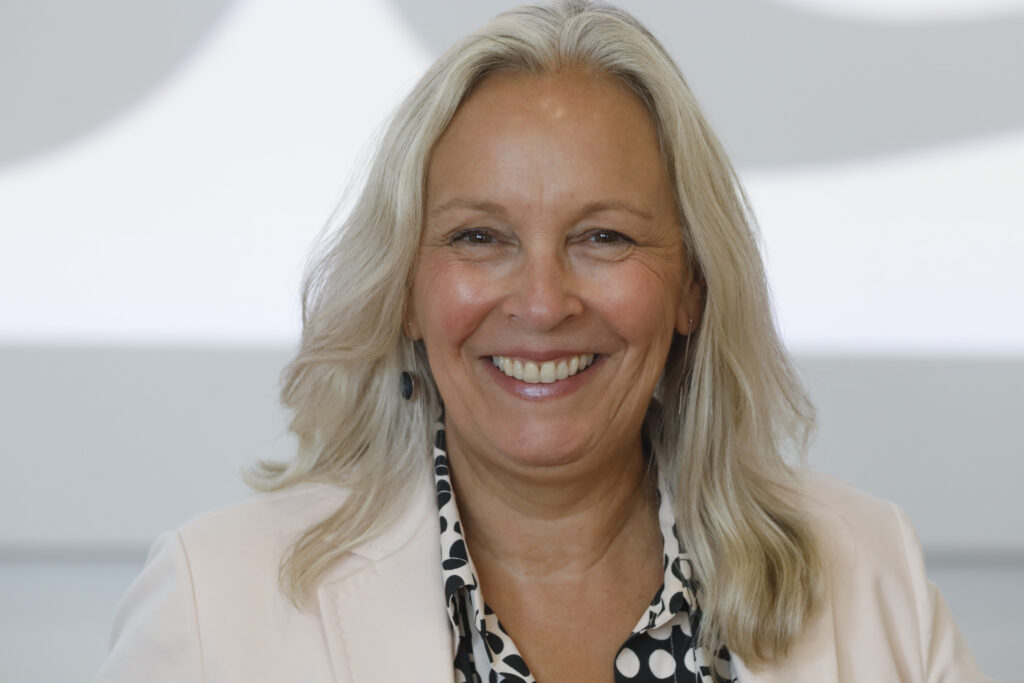
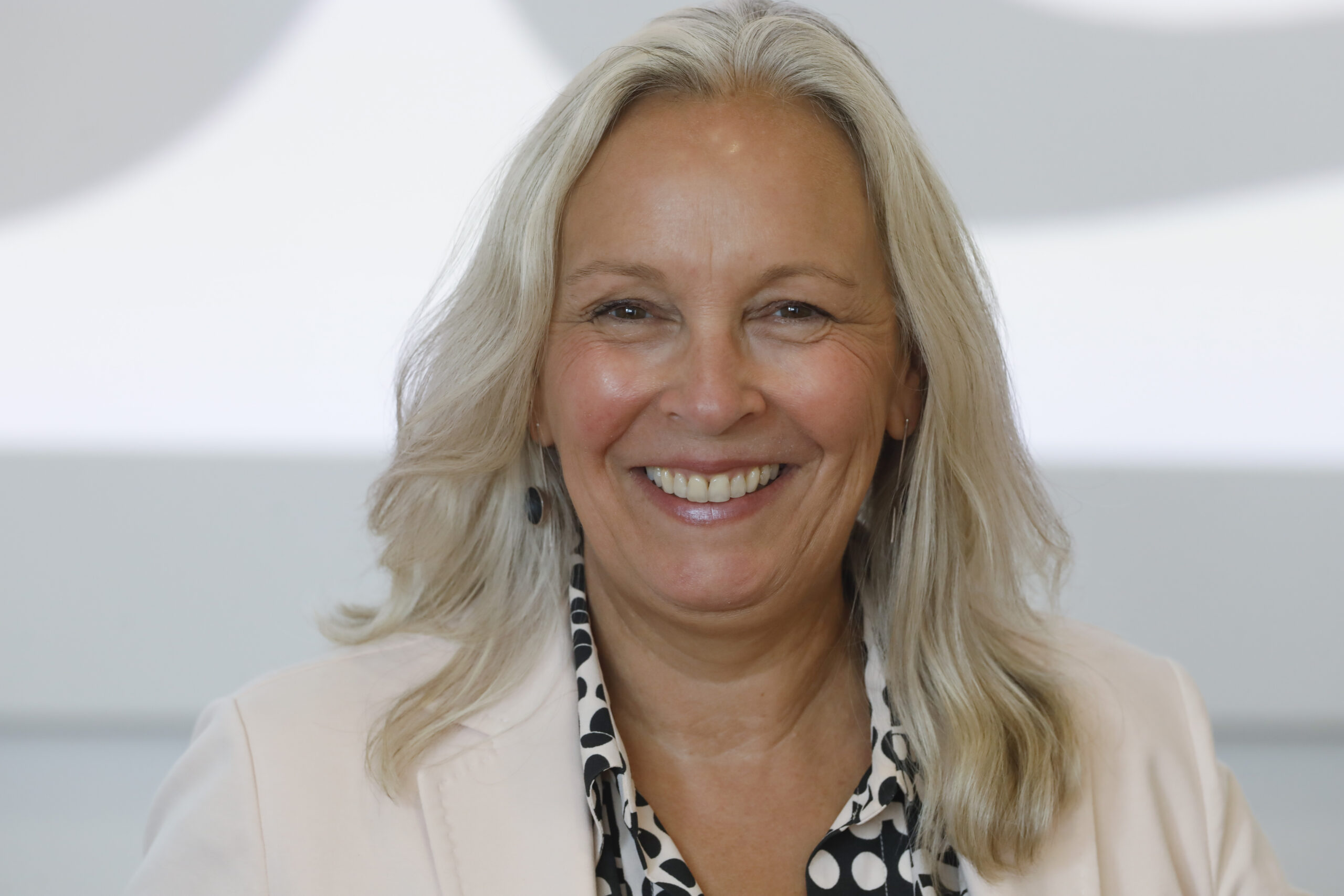
During the 33rd International Joint Conference on Artificial Intelligence (IJCAI), held in Jeju, I had the opportunity to meet with one of the keynote speakers, Gillian Hadfield. We spoke about her interdisciplinary research, career trajectory, path into AI alignment, law, and general thoughts on AI systems.
Transcript:
Note: the transcript has been lightly edited for clarity.
This is an interview with Professor Gillian Hadfield who was a keynote speaker at IJCAI 2024. She gave a very insightful talk about normative infrastructures and how they can guide our search for AI alignment.
Kumar Kshitij Patel (KKP): Could you talk a bit about your background and career trajectory? I want our readers to understand how much interdisciplinary work you’ve done over the years.
Gillian Hadfield (GH): I did a PhD in economics and a law degree, a JD, at Stanford, originally motivated by wanting to think about the big questions about the world. So I read John Rawls’ theory of justice when I was an undergraduate, and those are the big questions: how do we organize the world and just institutions, but I was very interested in using more formal methods and social scientific approaches. That’s why I decided to do that joint degree. So, this is in the 1980s, and in the early days of starting to use a lot of game theory. I studied information theory, a student of Canaro and Paul Milgram at the economics department at Stanford. I did work on contract theory, bargaining theory, but I was still very interested in going to law school, not to practice law, but to learn about legal institutions and how those work. I was a member of this emerging area of law and economics early in my career, which of course, was interdisciplinary, using economics to think about law and legal institutions. Over time, I got very interested in stepping back from our legal institutions and asking, “how well do they work”? I started thinking theoretically about the function of law, and one of the things I learned is that we don’t really have much theory of what law is. So, I started working on developing that theory, which I mentioned during the talk. That theory evolved into providing this framework for normative infrastructure. Now, you asked me about my career trajectory. I got to my PhD. My first position was at the University of California, Berkeley. I had a choice of whether to go into an economics department or go into a law school, and I ended up choosing to go into law school because I was concerned that if I went to an economics department, I was just at the beginning of this wave of more formal theory and I like doing math and all that work, but I thought that wasn’t really capturing the issues and ideas I was interested in. I thought I’d have more flexibility in a law school, which was true. My first position was at Berkeley. I then went back to Toronto, which is where I’m originally from, for about 5 years, and then moved to the University of Southern California. I was there for about 17 years, appointed both in the law school and the economics department. I moved back to Toronto in 2018 because I was starting to work on AI and had an opportunity to affiliate with the Vector Institute there and build my collaborations with computer scientists. And so this next move is to Johns Hopkins. It’s quite exciting. They’re starting a new school of government and policy right in DC. And the goal there is to start building how we think about governance and policy for the technological world. That also comes with a part-time appointment in the Computer Science department, which is my first appointment, so I’m very excited about that.
KKP: So the way you talk about your career trajectory and even when you were talking about how you got into AI alignment by talking to your son and you almost make it sound like it was very easy, but it’s very hard, and I can imagine requires a high capability to adapt to new people, new literature, new norms when you move between fields. How have you maintained such a high adaptivity over the years across your careers? Do you have some tips for people who want to do interdisciplinary work as well?
GH: One thing that is important to recognize is that, since my PhD is in economics, it is a technical, formal training. And there’s a lot of overlap in some of the frameworks that are used in AI. Even though I can’t program, I’m not a coder, but I think I’ll need to learn to do it at some point. But if you think about the notion of agents pursuing goals, there’s a lot of game theory. So I had a lot of the technical tools, and I did have this secret weapon: both of my sons were in computer science. And so I could ask every stupid question that I wanted to ask. And I was just very committed to really understanding what’s going on under the hood, both in order to be able to contribute and to have the confidence to get up and give a talk in front of computer scientists. I really do emphasize this to the colleagues that I’ve been trying to draw in from economics, law, and political science. Make an investment in really understanding what’s under the hood. And I really emphasize that most people who have PhDs in the social sciences have the sufficient technical capability to understand. I might not be able to build a multi-agent reinforcement learning system myself, but I have students and postdocs who can and I can talk to them about what they’re building and how we should build it. I can evaluate it. I can assess it. So that’s my advice: you really do need to invest. And you need to find a way to ask all your stupid questions. And if you don’t happen to have children who can answer those questions for you, find another source. I run boot camps for example.
KKP: At some point in your talk, you were talking about law and how law has evolved over time. It almost came off as you were talking about law as a technology which humans have developed. And after the talk I looked up and found your 2013 book which talks about why humans invented law in the first place and how it’s evolved over time. And of course I can’t ask you to summarize your entire book in this question, but if you could help computer scientists understand, broadly, how over the last two millennia or so, the legal systems have evolved, and in particular emphasizing how AI can help take this evolution to the next step. Are there any gaps here which you feel AI is a really good tool to be able to fill?
GH: So for an economist, a technology is just the transformation of inputs into outputs, right? If you start thinking about the challenge of organizing human societies as accomplishing this objective, getting to this outcome of everybody reasonably understands what they’re allowed to do, what they’re not allowed to do, and those behavioural requirements are actually being enforced, so you convert that into a challenge of how do you build a structure for that, a mechanism for that? So you could think of that as a technology. Once I started thinking about this theory of law, I spent a long time looking into the history and the evolution of, more generally, normative social order. That there’s a set of shared behavioral standards, and they’re basically enforced. And I really emphasized to my colleagues in economics and law and social science, for example, that this did evolve, that the institutions that we’ve used, the technology we’ve used for accomplishing that objective of obtaining that normative social order has evolved as the complexity of our human societies has evolved. In fact, in my book I argue that you can think of the dynamics as stable if a community is successful at maintaining stable normative social order, it will be more productive, it will generate more surplus. Now economists have a saying that the division of Labor is limited by the extent of the market. Basically saying that as we get growth, we can sustain the same greater levels of specialization in the division of labor. Well, that implies that human societies that are developing in that economic sense are also inventing new behaviors, inventing new goods, new technologies, and that’s going to present a challenge to their existing normative social order. They’re going to have to adapt. They’re going to have to develop new institutions that can say oh, you know, it used to be that it was considered wrong to sit under the tree and not contribute to productivity in the group. But now we have somebody who used to be a bow maker but who now has developed new techniques and spends their time supervising apprentices who make bows. So is that being lazy, is that sitting under the tree or is that a new activity that we approve of? I started thinking about how we evolved like that. And the way I look at human history, we’ve always had norms and rules. You can’t have a human group that doesn’t have rules. And what’s evolved is increasingly sophisticated and adaptive mechanisms, classification institutions for making these coordinating assessments of what’s acceptable and what’s not. So elders, chieftains, councils, rulers, kings, queens, parliaments, legislatures, juries, legal lawyers. We just moved in that direction. And one of the reasons that I think this is important for thinking about AI is because I think about AI, as you know, we really are on the cusp of something that’s just fundamentally transformative for human societies. And so the way I think about that is to say, oh, so we’re going to need to make the next leap on how we solve this problem of developing shared classifications, enforcing those classifications and adapting them. So AI will go faster, we’ll have totally new types of relationships, we’ll have totally new agents in the system. I think that building the theory of legal order and normative social order is critical for helping us both predict and design those new institutions with AI.
KKP: I was attending a tutorial at the conference two days ago; I think it was machine ethics. The presenters raised a very interesting point that AI systems, we want them to be moral agents in the sense that they can think about moral questions while choosing to do things. But we don’t necessarily need to think of them as moral patients in the sense that if you want to blow up someplace with a bomb, then having a robot as a casualty is a very different moral question compared to having humans as a causality. I wonder if that allows AI agents a certain sense of being able to look at legal questions while keeping themselves apart because and again I’m saying all of this as if they have consciousness, which we don’t necessarily have any reason to believe they do, but, even thinking of them as a black box, there’s a certain, I don’t want to say they are devoid of any biases because they are biased in ways humans are biased or humans quote them to be biased, but there are at least possibilities of making things unbiased in universal provable ways which potentially humans cannot do. And is that something you’ve thought about when you think about using AI agents in either law or governance?
GH: Yeah, so there’s a lot in there. I actually want to offer a counterpoint to the idea that we want our AI agents to be moral agents. I’m trying to offer a theory of normativity that doesn’t say that there’s just some attribute that humans have that’s moral. I say, well, actually, morality is a classification scheme and we coordinate on that. And so, absolutely AI systems, when I say they need to be normatively competent and be able to integrate into that normative system, and that they should be able to, like we do, predict, given my community uses this system of reasoning to decide what’s acceptable or not and resolve hard questions about what’s acceptable or not. Yeah, we should be building AI systems that have that capacity as well. That’s my concept of normative competence. The question of moral patienthood, gets into would AI agents be entitled to the protections of some of that moral reasoning? I think of that as a positive predictive question, not a normative one of should they be. I think people who are talking about moral patienthood in terms of all of their consciousness, they can experience suffering, I don’t think that’s an account other than that a reasoning system we’ve developed for humans. I think if we end up giving robots rights it will be because that more effectively implements our normative system.
The last part of your question was about if we could imagine that AI may be able to help us with our classification process. I think that’s an empirical question. It’s a hopeful thing that, maybe with this computational system, we will do a better job at, say, reconciling. A lot of our normative systems consist of exchanging reasons, arguments, evidence, and we know that some of that is flawed in human institutions. So maybe if we have entities that are capable of doing that reasoning in ways that are less likely to make errors associated with self-interest, that could be valuable. But at the end of the day, the stability of your normative system, your normative social order, depends on the voluntary decisions by all the humans involved. So we can’t just in a technocratic way say, the AI is getting closer to the correct answer. It doesn’t really matter what the correct answer is. What matters is that it’s one that most of the age and most of the people in your system look at it and say, oh, I think that’s fair. Obviously, we have people capable of doing that reasoning to different degrees in the world today. But you still have to defend, even if you’re saying, well, this is one of the smartest people there on our Supreme Court, or they’re one of our top philosophers, ordinary people need to be able to relate to it and say I trust that it’s fair reasoning.
KKP: So if I understood your last bit correctly, when we have axiomatic systems and we want to derive theorems or conclusions or propositions out of them, AI in particular can really help us scale these issues without potentially logical fallacies which humans could have conducted, but it still remains challenging to make things interpretable so that us humans can understand the scale at which these systems are operating.
GH: Exactly. If we think about explainable AI, which I think is better called justifiable AI, there might be limits to what we would allow an AI system to do that we might say, oh, we could in some sense prove that the world would be better off if we let the system make the decision. But if you couldn’t present justifications that were reasonably understood by a large enough share of the population, then, that’s just going to be a constraint. It’s like, you know, our courts have to be able to explain what they’re doing in ways that the population will accept. When you start thinking about what you’re trying to do to maintain or improve that equilibrium, rather than trying to achieve some ground truth, or think that there’s a correct way of reasoning about harm, or there’s a correct way of doing utilitarian calculus or cost benefit analysis. At the end of the day, human societies depend on most people feeling confident that the world they’re living in is meeting certain criteria.
KKP: So the next question may be a bit of a cynical one. I’ve been the opposite of an interdisciplinary person. I did my undergrad in computer science and started my PhD right after in computer science, and the only thing I’ve worked on is machine learning. My career started at the point when machine learning was rising and I just don’t know how conscious that decision was. One thing I often get frustrated by is the tendency of this community to reinvent things over time and the cynical bit here is I don’t understand why AI alignment is relevant today more than it was yesterday and I don’t understand in particular why a lot of the examples which are used to cite a need for AI alignment are just not examples of a failed learning procedure. Like I was thinking about the boat racing example that you mentioned and I could very well have cast it as an issue in improper loss design or the feedback for the reward was not correct or there was some spurious reward pathway which led it to go around in circles. How do you look at this? Because you also have an outsider’s view, partly by looking at this issue. Why is AI alignment all the hype right now?
GH: So by the way, I want to agree that one of the things that I coming in as somebody from another disciplinary background, and one of things that’s kept me initially kept me engaged, was to say, “holy cow, these folks are all reinventing everything”, right? Like I would be in discussions, “I know how we’ll align with one agent, how we can align with multiple agents, how we can aggregate?”. OK, economists have been thinking about the aggregation of utility and welfare for a very long time. That’s a political process; that’s your theory of the market. And so I think you asked me earlier how did I become competent enough to have conversations and collaboration with computer scientists. So that’s the flip side is people who want to work in machine learning, if you don’t have that background, that’s what I was also trying to do in my talk was to say “let me introduce you to some other ways of thinking about normativity and normative structure”. So I think that AI alignment has become a bigger… I was drawn into this small niche area of AGI safety back in the early days. And I had no idea whether we could build anything called AGI, but I found it intellectually challenging and I felt I had something to contribute to be able to think about “this sounds like a problem of the rewards on the boat racing game”. I’ve heard that from economists. This is what economists were saying; “if you’ve got the contract designed incorrectly, you should have just designed it better” and then taking on board the idea that you can never design it perfectly. So yes, you could look at the boat thing and say it’s actually very obvious – your reward signal was the score in the game and you didn’t give it any reward for winning so you could fix that, right? But you can’t do that with everything. So you have to think about how you align. I suggested in the talk the reason I think this has become much more mainstream is because foundation models basically. So the idea that we’re increasingly on a path of building powerful general-purpose systems that will then be the whole idea of a foundation model. The idea that you can build all kinds of things on top of it, and now we’re going to build agents. Mark Zuckerberg when they release Llama 3 was saying, oh, you know, any small business will be able to build an agent to do these functions for it. So then the question of ”OK, how do these systems behave and is that aligned with the welfare of groups, is it aligned with social welfare, is it creating externalities for other agents?”. I think that’s why that’s become a bigger issue. I agree with you that if we’re thinking about the alignment question, so there’s… In some ways the definition of alignment I feel like has really narrowed recently. I just heard Dario Amodei at a conference recently say, alignment means doing what the designer intended, or what the owner intended. Well, that’s a very narrow idea. In some ways, that is just an engineering problem. The control mechanism for that is going to be “I’m not going to use it. It doesn’t do what I want it to”. You’re not going to be able to sell it to me if it’s not winning the boat race, I’m not buying that agent. Right? It’s when you start thinking about what are the implications on everything else. If you’re going to put it up there and it’s going to start contracting, OK, well, we’ve got lots of legal rules that preserve the efficiency, productivity, efficacy of our markets, like fraud rules or contracting, contract breach rules or property rules. The behaviors you could get out of that foundation model or LLM-based agent now have lots of implications for other people, but then also just for the stability of our system, right? Will our antitrust rules work if we’re starting to delegate pricing decisions to LLM-based agents or AI agents more generally? So I think the alignment question, especially if you start looking at it through this lens of is it aligned with all the rules that make our society work? That’s why that’s become a bigger question.
KKP: So essentially, the fact that these systems seem much more capable than they did before has pushed us into thinking more aggressively?
GH: Yeah, so the increased autonomy and increased generality. I’ve seen lots of talks here at IJCAI, Thomas Dietterich’s talk this morning, and Bo An’s talk yesterday, talking about the evolution in AI from very task-specific domain-specific build a system to chase and pursuit, classify bugs, and you could say, “well then you’re kind of in a closed box of who cares about how well it performs”. But once you’re saying we’re going to integrate this into fairness and machine learning, if we’re going to start delegating decision making in banks or governments or housing authorities, actually the way this is built matters more than just the purchaser or the buyer/ designer of that system, and I think the foundation models, language models, again, increasing autonomy and increased generality.
KKP: I agree with what you said about the normative infrastructure and the need to build it for alignment issues in AI, but one question that comes to mind is it almost sounds like a naturalistic fallacy, like in the sense of it being a humanistic fallacy here. Because, why should it be the case that AI models be based on how human societies work, or the best way to get a particular outcome out of them be based on how human societies get that particular outcome? Because in the process of doing that, one could potentially lose what these algorithms are good at. How do you avoid scenarios where potentially you can build in things like lying or being dishonest because of some human structure that you’re trying to build into an AI system? And it’s a very broad question, but what is your perspective? Maybe to summarize at all, perhaps the solution you suggested is a sufficient solution for getting the kind of behavior that we want from the AI model, but is it a necessary thing to incorporate in these systems? Is there a theoretical way to show that without a normative infrastructure one could never hope for getting alignment out of incomplete contracts or some theoretical statement about that?
GH: So my methodology approaches these questions from what an economist would say is a purely positive standpoint. So I’m not starting from a normative premise. And I’m not saying we should align our systems because that’s the good thing to do. That would be normative. My claim is that normative infrastructure is serving this instrumental goal of achieving social order in a group of interacting agents. I’m modeling humans like that. The original model here is a very conventional economic model in the sense that humans are modeled as having a utility function, they are trying to achieve objectives. They need to coordinate on enforcement if they’re going to deter other actors, other agents from taking actions that will reduce their own welfare, so I have my goals, you have your goals, there’s a third party over there who might be causing problems for either of us. And the only way we can deter that third person is if we both join together in boycotting them. So that’s very instrumental. And that says, for all of the agents in this group to collectively achieve their goals, and in fact, to produce more so they can both be productive because that’ll produce more for us collectively as well. So if you think about introducing AI agents into human societies, humans would want them to be aligned with our normative infrastructure because we don’t want to admit somebody to our club who doesn’t know how to follow our rules, right? They are just going to create chaos and havoc. So it’s a very instrumental story and it’s about humans saying we need this because instrumentally we don’t want to wreck our system. So that’s one answer. Now the other is the thought experiment of: suppose you had a community, exclusively composed of artificial agents and Peter Railton, who is a philosopher at Michigan, I just came across his work and met him and had some great conversations this summer, he’s been thinking about that thought experiment as well. If AI agents need other AI agents to accomplish their own goals, if there are benefits to them, in the same way there are benefits to humans of a specialization and division of labor, then the theory predicts they too will need rules of behavior, because they’re gonna want to engage in exchange and specialization. I have data different from the data you have. And when you think about normative systems as functional, instrumental, performing that role of increasing the capacity of all the members of the group to improve their outcomes for themselves, then it’s really no reason to think that AI agents wouldn’t invent the same. Now they may invent different kinds of institutions, but it would perform the same function of classification and enforcement. And I remember when I first started talking with my son Dylan and then members of the AI safety community about these issues like 2016/2017 and at the time the existential worries were around a super intelligence, and so the vision was like the paperclip maximizer, like the AI that just takes over the world. And I remember saying, I don’t have a clue of what direction this technology goes and so on, and I’m not the one to say, but, if we’re on a path to a single superintelligence, we’re cooked because there’s no reason why it would be responsive to humans. But if AI develops on a path where AI needs humans or needs other AIs, then I think you’re OK, because then you have the raw materials for the development of normative social order. There’s an incentive for all those agents to build institutions of classification and enforcement.
KKP: That makes a lot of sense. The next question is on a slightly different theme. I work in collaborative learning, and this is something I’ve been thinking about recently. As AI systems become better, it’s also becoming clearer that there are ceilings on training data procurement or getting good valuable quality data which we can train these systems on to further improve their capabilities. It seems almost inevitable that at some point we’d need to start compensating people to give us data or generate data. What are your views on questions around compensation for data and how should we build laws around it? In particular, the issue of artists’ rights to their own creations and how certain models, like diffusion models, can often memorize a big chunk of their training data has brought a lot of these questions to the surface and when I talk to computer scientists about this, the technological optimists always tell me that who’s to say that humans don’t copy the content that we actually believe that we create? The legal perspective is of course different because there is a law on plagiarism and there are laws about ownership. But again, I was reading a book titled Mine by NYU lawyers recently, and I just realized that the laws around ownership are as messy as they can get. Essentially it’s a game about who has the most power and can actually exploit the ambiguities surrounding ownership who gets the most out of the system. So long story short, how do we navigate our way around this mess of data compensation, privacy rights, and ownership of the data on which these things are trained and there are emerging rights and laws – every year new laws are coming in and it’s even unclear if we can implement those laws. So, how do you provably show that you’ve deleted someone’s data from an AI model?
GH: So In my career, I’ve thought a lot about why the law doesn’t innovate fast enough to keep up with changes in technology and economies. I remember, my historical saying, is as societies grow, they invent new things, they have new classification tasks and their existing institutions will deal with it very well. And one of the things I leave my book with is to say I want to shake the presumption that law, the way it is today, is what it means to have law. It’s written by government, it’s expressed in text. And say, well, no, it’s performing this function, maybe it needs to look quite different. So when we think about things like creative work. So our laws on copyright were invented in the wake of the printing press, and one of the things that I think is quite misunderstood by the conversation about creative work in the context of machine learning is the idea that the creator owns what they created. And then therefore, they have the capacity to prevent anybody from using it if they don’t get paid. That’s actually not accurate, and that would be a bad solution from an economic point of view. And this was actually a paper I wrote in my last year of law school that I won a national award for, about the copyright laws that we have (this is in the US) are fundamentally economic. They were put in place in order to protect the incentive to create new works. And from an economic point of view, like from a welfare point of view, because the marginal cost of additional people using a creative work, an intangible work, is zero, we’d start off with the idea that the optimal distribution of that good is that everybody has it. If we did that, if we made it zero, then we would lose incentives to create, if you think there’s only a financial incentive to create work. So copyright law, and even more clearly, patent laws deliberately trade off how much protection we need to give to the creator to secure their incentive to produce creative works. We want to give protection as enough to secure that, but we then want as many people as possible to benefit from the value of that work, the information of that work. It’s a public good. We learn from works all the time. I can go to the museum and I can look at all the paintings and if I was an artist, I could learn from studying the paintings, I could even sit there and try and reproduce it, to learn about techniques and brush strokes and all that stuff. So when we’re training machines. to learn about producing creative works, art, music, literature, in a sense, we’re saying, OK, they can learn just like humans can learn from it. What we want to think about is what kinds of institutions do we need or what kinds of laws would we need in order to protect the incentive of people to continue to produce that work. Now we could also say we want fair compensation because I think it doesn’t make any sense at all that creators are basically not gaining from their creative work in this way, because OpenAI or Stable Diffusion or whatever scooped it all up and created this thing they can earn massive profits from. That doesn’t seem fair. Just like we invented copyright law for the printing press, we need to invent something that accomplishes this objective. I don’t think we get there by saying, “oh, they have copyright in their work”. Obviously, if the model produces a duplicate of their artwork, then that is clearly copyright violation. But if it is, just learning to draw pictures by having consumed a whole bunch of pictures that artists have created, we may want a new mechanism for sharing that wealth. We may want to preserve their incentive. You started off your question with would we just see compensation? And I think we would, if the builders of the model said nobody’s willing to do artwork anymore. I don’t think that actually would happen, but if nobody’s willing to put it on the Internet, or won’t distribute it on the Internet and make it available to us anymore, then yeah, there’s going to be an economic incentive for them to say, “oh, we are going to start to pay”, or “we’re going to start art fellowships” or “we’re going to hire a lot of artists”. Then we’ll have compensation mechanisms that will arise.
KKP: Would it be appropriate to say that the new incentive structures we need to develop would essentially be conditioned on the fact that AI can already generate a lot of art, and perhaps the human artistic expression we need now is the one that supersedes it, so we want to incentivize that more?
GH: Yeah. So I was just in Vienna, and spent a lot of time in wonderful art museums and I was saying to my husband as we were walking around, there’s no chance that we’re not going to have… I mean human artists, one of the things that drives them is everybody else is doing it this way, can we invent a new way of doing art? And. in order to produce all we want in art, and we need to compensate people for that, then I think that’ll emerge. When you get the radio and people playing music on the radio like they invented a new system for compensating songwriters for their compositions, with an organization, ASCAP, that said “you’re going to share revenues, you’re not going to have individual contracts between the radio station and every songwriter”.
KKP: What’s your biggest fear about large scale deployment of AI systems? Maybe to contextualize it a bit more, we actually have those fears when you see that a lot of the powers being concentrated in hands of a few technology companies, largely speaking, do not have open models and there’s little to no way to look at these models beyond how much these companies want us to look at them. There was this famous incident at the UK AI Institute. They had some contract with Anthropic that they wanted to look at their pre-release models, but Anthropic denied doing that. Do you fear anything about the way AI is being deployed in 2024?
GH: So yes. My concerns about big models are around the potential to cause catastrophic disruption to human societies. I don’t really worry about rogue AI. I worry about misuse, but in the same way that we should just say, “oh, if there are terrorists or criminals, and they have a new tool and we need new systems to respond to that”. But I do worry about companies saying, “we’re going to release a whole bunch of … we’re going to let people build agents”. I mean, we already have that with the capacity for plug-ins with GPT4 and so on. As I understand it, what’s happening in the industry is that billions are going into this agentic development. And what I worry about there is that we are pushing forward very fast in the complete absence of basic standard legal infrastructure to manage that. I think for the first time in history, we have massively consequential technology that is being built pretty much exclusively within private technology companies. And so it’s protected by an artificial legal fictional wall that we invented, you know, a few 100 years ago. Very productive, the idea that you had something called a corporation and then we protected the information in that corporation, we created incentives that way. But now that fictional wall is getting treated like it’s a real thing. And we’re saying, “oh, well, the companies own, like they’re allowed to keep completely to themselves what it is they’re building, how it works, what they know about its capabilities and so on”. And I think because that has massive implications for how we even build regulatory infrastructure around that. We should absolutely not be dependent on what these companies voluntarily want to share. So I and Tim O’Reilly and Tino Cuéllar have a proposal saying we should be requiring at a minimum to get us started registration of frontier models that would involve disclosing to governments confidentially, not putting it out on the Internet in the public, but disclosing to governments basic things about models, the size, what we know about capabilities, what kinds of evaluations have been done, what data was it trained on. And to do that as laying the groundwork for… we need to have visibility, governments need to have this visibility. Maybe we don’t need to do anything. Maybe it’s not going to develop the way that the companies expect that it will. But at a minimum you have to have government visibility into that.
With respect to agents, I think we need legal infrastructure that says “OK, if you want to work in this economy, you have to be authorized to participate in the labor market, and you have to have an identification”. When you get a job, you have to show your Social Security number, you’ve got to prove that you’re a citizen or that you have a valid work visa, and you have a name, an address. So, if you want to start a business in a state, you need to register that corporation with the Secretary of State. You have to disclose who are your directors, what’s your ownership structure, what’s your legal address. The legal address is important because that is the address at which anybody wants to sue you, another private party or the government, as long as they send the legal documents to that address, they have properly started the lawsuit. And if you ignore it and don’t show up, the court will be authorized to enter default judgement without you and seize your assets or whatever. So then I say, well, we’ve got these new AI agents that are going to start participating in the economy. Well, we don’t have any structure around that. We should, at a minimum, require a registration of those agents and then a capacity to integrate them into our legal system. Maybe that has to go as far as something like legal personhood for AI agents or minimum asset. So these are the things I worry about, I worry that we’re going to blunder our way into a whole bunch of actors, autonomous agents acting in the economy without any of the basic legal infrastructure that we have in place for humans and corporations, which are also artificial entities.
KKP: It’s been really interesting. Let me end because I think we’re already close to our end time. Final question: what advice would you give to an early career researcher, something maybe you would have told yourself when you started your academic career?
GH: So part of what I wanted to do in my talk today and my proselytizing with the AI community is, there are so many important, rich questions and sometimes you have to step back to see what those questions are. So I’m trying to provide that step back, and say let’s talk about building normatively competent agents. Let’s talk about building normative infrastructure. Even though I was presenting legal structures like if you were going to create identification systems for AI agents, I mean, there’s a lot of technical questions in that. It’d be like, OK, if you had to verify the registration or verify the identification of systems, like somebody in the talk asked the question about agents that could just duplicate themselves and say, OK, so that would be a problem to solve. So I think there’s a lot of questions, and when I go through a conference like this and I see a phenomenon I saw in economics, which is also why I didn’t go into an economics department is, a lot of people who are attracted to these fields, they’re excited by technical like, oh gosh, look at that beautiful proof. Look at this technical challenge, right? They’re excited about accomplishing it. And you can see a lot “well, I’ve got a hammer that must be a nail” phenomena of, “oh, they’re interesting technical, theoretical, I could show this game theoretic result or whatever”. And I see a fair bit of that happening and it feels a little disconnected from saying, “oh, wait a second, this stuff’s really happening and there are real consequences for the world”. I think there’s a lot of open questions, a lot of rich research agendas that there’s nobody working in. So one of the bits of advice for early career is to find the problem people aren’t working on. The other is, a lot of PhDs in AI have these real choices between pursuing an academic path or pursuing an industry path, or pursuing work with the AI Safety Institute. If you’re interested in the academic path, I think it’s really important to remember that the only reason to be an academic is because of the freedom you have to work on what you decide is an important and interesting question. So I see sometimes too many people in academia who are saying, “oh, what does everybody else working? How can I prove that I’ve got a smarter answer?”, and they’re driven by that. And I think well, you’re wasting. Unless you’re working on something you’re really motivated by that you feel is important, then you’re squandering what academia has to offer. And it also means that we’re not getting enough creativity out of our academic world.
KKP: I mean, of course, the incentive structures also have some role to play in how people end up making a lot of choices.
GH: They do. I just think that our universities have become a bit of a machine in that way, and I think we’ve lost some of what universities should be doing. I have felt very motivated in my life, in my academic life, by I think this is an important question to work on and I feel like I have something important to add to the world stock of knowledge on this important question. And that’s why, can I even admit it, 40 years later here I am, heading off in new directions and very energized. I figure I got another 30 years to go. That’s the great joy of, and fulfilling way, to have an academic career. I feel sad when I see too many of my students who are saying “oh, but I need to get this publication and that publication”, and saying step back, you have a wonderful brain. Figure out how to use it to add value to the world.
KKP: Thanks. That was a wonderful end to the interview, I hope our readers will also find it as interesting, insightful and lovely takeaways to take away from as I did.
About Gillian
Gillian K. Hadfield is an economist and legal scholar turned AI researcher thinking about how humans build the normative world and how to make sure AI plays by rules that make us all better off. She is Professor of Government and Policy and Research Professor in Computer Science at the Whiting School of Engineering at Johns Hopkins University. She is on leave from the University of Toronto as Professor of Law and Professor of Strategic Management. Hadfield holds a Canada CIFAR AI Chair at the Vector Institute for Artificial Intelligence and is a Schmidt Sciences AI2050 Senior Fellow. Hadfield’s research is focused on computational models of human normative systems; working with machine learning researchers to build ML systems that understand and respond to human norms; and innovative design for legal and regulatory systems for AI and other complex global technologies. She is a faculty affiliate at the Center for Human-Compatible AI at the University of California Berkeley and she was previously the inaugural Director (2019-2023) and Chair (2019-2024) of the Schwartz Reisman Institute for Technology and Society at the University of Toronto, and a senior policy advisor at OpenAI. Her book Rules for a Flat World: Why Humans Invented Law and How to Reinvent It for a Complex Global Economy was published by Oxford University Press in 2017; a paperback edition with a new prologue on AI was published in 2020 and an audiobook version released in 2021.