AI-Driven Data Analytics for Enhanced Creditworthiness Assessment
Creditworthiness assessment plays a crucial role in the financial ecosystem, as it helps lenders determine the likelihood that a borrower will repay a loan. Traditionally, this process has been based on static indicators like credit scores, financial statements, and a history of loan repayments. While these methods have their merits, they also have limitations. For instance, they often miss valuable insights that could lead to more accurate and fair evaluations. Enter Artificial Intelligence (AI) , a game-changing tool in modern finance that can provide a more comprehensive, precise, and efficient way of assessing creditworthiness. In this blog, we will explore how AI and data analytics are transforming creditworthiness assessments, offering a deeper look into the technologies involved and their potential benefits, challenges, and ethical considerations. The Traditional Approach to Creditworthiness Assessment Before diving into AI’s role, let’s quickly review the traditional methods of evaluating creditworthiness: Credit Scores: The FICO score is perhaps the most widely recognized method for assessing an individual’s creditworthiness. It is based on a number of factors, including payment history, credit utilization, the length of the credit history, new credit inquiries, and the types of credit accounts. Credit Reports: Lenders frequently review a borrower’s credit report, which contains information about an individual's credit accounts, including loans, credit card balances, payment history, and even bankruptcies. Income and Employment History: This is typically assessed through pay stubs, tax returns, and employer verification. Lenders want to ensure that the borrower has a stable income to support loan repayments. Debt-to-Income Ratio (DTI): This ratio measures the proportion of a borrower’s monthly debt payments to their monthly income. A high DTI indicates that a large portion of the borrower’s income is already committed to existing debts, which may make it more difficult for them to repay additional debt. While these methods are effective in many cases, they often fail to give a complete picture of a borrower’s financial behavior. For instance, someone who has consistently paid rent and utility bills but has no credit cards may be considered a poor credit risk using traditional methods, despite their strong financial discipline. The Role of AI in Creditworthiness Assessment Artificial Intelligence and machine learning are taking the traditional creditworthiness assessment to new heights. By leveraging massive datasets and advanced algorithms, AI can offer a much more nuanced understanding of a borrower’s financial health. Here are some ways AI is changing the game: Big Data Integration Traditional creditworthiness assessments rely on a limited set of data, such as credit reports and financial statements. AI, however, can analyze a broader array of data points, including social media activity, online spending behavior, and even mobile phone usage. By tapping into these alternative data sources, AI provides lenders with a richer understanding of a borrower’s financial habits, which can lead to more informed and fairer credit decisions. Predictive Analytics and Machine Learning AI can process and analyze large quantities of data to uncover hidden patterns and predict future behavior. Machine learning algorithms can identify trends in a borrower’s spending, saving, and borrowing behaviors, which can offer valuable insights into their future repayment likelihood. These models continuously improve as they analyze new data, leading to increasingly accurate predictions over time. Real-Time Decision Making One of the most significant advantages of AI is its ability to process data in real time. This allows lenders to evaluate creditworthiness instantly, reducing the time it takes to approve or deny a loan. Faster processing times also mean that customers receive quicker responses, which can improve their experience and lead to greater customer satisfaction. Risk Mitigation AI can help reduce the risk of loan defaults by identifying potential risks before they become problematic. By analyzing a wide range of data points, AI can flag borrowers who may be at risk of missing payments. This proactive approach to risk management enables lenders to take preventive actions, such as adjusting loan terms or requiring additional documentation, reducing the chances of default. Personalization of Credit Offers AI also allows lenders to tailor their offers to individual borrowers. Instead of using a one-size-fits-all approach, AI-driven analytics can assess a borrower’s unique financial situation and create a more personalized credit offer. This approach not only benefits borrowers but also enables lenders to optimize their portfolios by offering loans with terms that best match the borrower’s risk profile. Key AI Technologies Dr
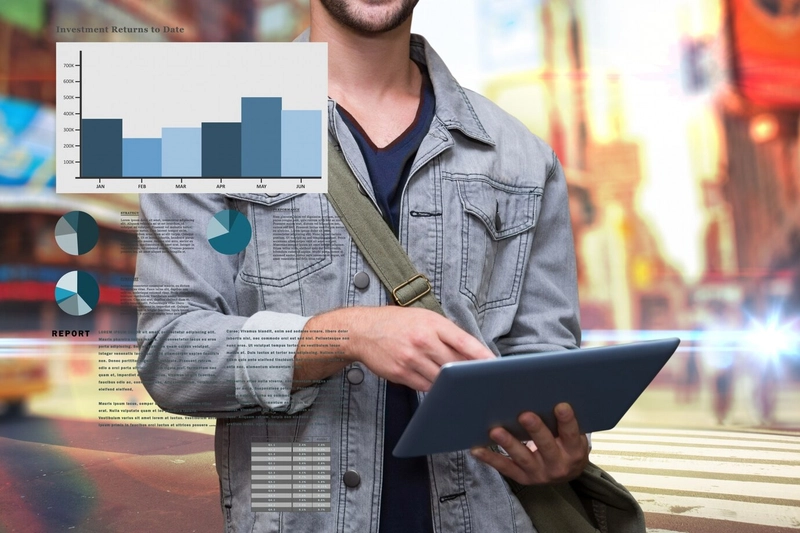
Creditworthiness assessment plays a crucial role in the financial ecosystem, as it helps lenders determine the likelihood that a borrower will repay a loan. Traditionally, this process has been based on static indicators like credit scores, financial statements, and a history of loan repayments. While these methods have their merits, they also have limitations.
For instance, they often miss valuable insights that could lead to more accurate and fair evaluations. Enter Artificial Intelligence (AI) , a game-changing tool in modern finance that can provide a more comprehensive, precise, and efficient way of assessing creditworthiness.
In this blog, we will explore how AI and data analytics are transforming creditworthiness assessments, offering a deeper look into the technologies involved and their potential benefits, challenges, and ethical considerations.
The Traditional Approach to Creditworthiness Assessment
Before diving into AI’s role, let’s quickly review the traditional methods of evaluating creditworthiness:
Credit Scores: The FICO score is perhaps the most widely recognized method for assessing an individual’s creditworthiness. It is based on a number of factors, including payment history, credit utilization, the length of the credit history, new credit inquiries, and the types of credit accounts.
Credit Reports: Lenders frequently review a borrower’s credit report, which contains information about an individual's credit accounts, including loans, credit card balances, payment history, and even bankruptcies.
Income and Employment History: This is typically assessed through pay stubs, tax returns, and employer verification. Lenders want to ensure that the borrower has a stable income to support loan repayments.
Debt-to-Income Ratio (DTI): This ratio measures the proportion of a borrower’s monthly debt payments to their monthly income. A high DTI indicates that a large portion of the borrower’s income is already committed to existing debts, which may make it more difficult for them to repay additional debt.
While these methods are effective in many cases, they often fail to give a complete picture of a borrower’s financial behavior. For instance, someone who has consistently paid rent and utility bills but has no credit cards may be considered a poor credit risk using traditional methods, despite their strong financial discipline.
The Role of AI in Creditworthiness Assessment
Artificial Intelligence and machine learning are taking the traditional creditworthiness assessment to new heights. By leveraging massive datasets and advanced algorithms, AI can offer a much more nuanced understanding of a borrower’s financial health. Here are some ways AI is changing the game:
Big Data Integration
Traditional creditworthiness assessments rely on a limited set of data, such as credit reports and financial statements. AI, however, can analyze a broader array of data points, including social media activity, online spending behavior, and even mobile phone usage. By tapping into these alternative data sources, AI provides lenders with a richer understanding of a borrower’s financial habits, which can lead to more informed and fairer credit decisions.
Predictive Analytics and Machine Learning
AI can process and analyze large quantities of data to uncover hidden patterns and predict future behavior. Machine learning algorithms can identify trends in a borrower’s spending, saving, and borrowing behaviors, which can offer valuable insights into their future repayment likelihood. These models continuously improve as they analyze new data, leading to increasingly accurate predictions over time.
Real-Time Decision Making
One of the most significant advantages of AI is its ability to process data in real time. This allows lenders to evaluate creditworthiness instantly, reducing the time it takes to approve or deny a loan. Faster processing times also mean that customers receive quicker responses, which can improve their experience and lead to greater customer satisfaction.
Risk Mitigation
AI can help reduce the risk of loan defaults by identifying potential risks before they become problematic. By analyzing a wide range of data points, AI can flag borrowers who may be at risk of missing payments. This proactive approach to risk management enables lenders to take preventive actions, such as adjusting loan terms or requiring additional documentation, reducing the chances of default.
Personalization of Credit Offers
AI also allows lenders to tailor their offers to individual borrowers. Instead of using a one-size-fits-all approach, AI-driven analytics can assess a borrower’s unique financial situation and create a more personalized credit offer. This approach not only benefits borrowers but also enables lenders to optimize their portfolios by offering loans with terms that best match the borrower’s risk profile.
Key AI Technologies Driving Creditworthiness Assessment
Several AI technologies are at the forefront of transforming creditworthiness assessments, including machine learning (ML), natural language processing (NLP), and deep learning. Each of these technologies contributes to a more sophisticated and holistic understanding of a borrower’s financial profile.
1. Machine Learning (ML)
Machine learning algorithms learn from data and improve their predictions over time. In the context of creditworthiness assessment, ML models can process historical credit data, loan repayment histories, and other relevant financial indicators to predict future borrowing behavior. Common ML techniques include:
Supervised Learning: This technique uses labeled data (e.g., past loan outcomes) to train a model to predict future outcomes.
Unsupervised Learning: Unlike supervised learning, this technique analyzes unlabeled data to find patterns or clusters that may indicate creditworthiness.
2. Natural Language Processing (NLP)
NLP is a branch of AI that enables machines to understand and interpret human language. In creditworthiness assessment, NLP can be used to analyze unstructured data sources, such as customer service interactions, emails, or social media posts. By understanding the sentiment and context behind the words, NLP can provide additional insights into a borrower’s trustworthiness and financial behavior.
3. Deep Learning
Deep learning is a subset of machine learning that uses neural networks to process complex datasets. It is particularly useful for analyzing unstructured data, such as transaction histories, customer communications, and social media activity. Deep learning can detect patterns that may not be obvious using traditional credit scoring models, providing lenders with a deeper understanding of a borrower’s financial situation.
4. Alternative Data Sources
AI-powered systems can incorporate alternative data sources, which are not typically included in traditional credit reports. These may include:
Utility and Rent Payments: Many people make regular utility or rent payments but have no credit history. AI can use this data to assess a borrower’s payment behavior.
Mobile Phone Usage: Data from mobile phones, such as payment behavior or app usage patterns, can also help determine creditworthiness.
Social Media and Digital Footprints: AI can analyze social media activity to gauge a borrower’s behavior, sentiment, and even their lifestyle choices, providing deeper insights into their financial habits.
The Benefits of AI-Driven Creditworthiness Assessment
The integration of AI into creditworthiness assessment offers several key benefits:
1. Increased Accuracy
AI can process vast amounts of data, leading to more accurate assessments. By analyzing a wider array of data points, AI can identify financial behaviors and patterns that traditional models might miss. This leads to better credit decisions and helps reduce the risk of default.
2. Faster Loan Processing
AI-driven creditworthiness assessments can be completed in real time, allowing lenders to approve or reject loans almost instantly. This speed not only improves operational efficiency but also enhances the customer experience, as borrowers get faster responses.
3. Expanded Access to Credit
By using alternative data, AI can help underserved populations, such as those with no credit history or those who have been traditionally excluded from the financial system gain access to credit. AI opens up opportunities for individuals who may otherwise be denied loans, providing more equitable access to financial services.
4. Enhanced Risk Management
AI’s ability to analyze large datasets enables it to identify high-risk borrowers more accurately. By flagging potential risks early on, lenders can take preventive measures to reduce the likelihood of defaults, ensuring healthier loan portfolios.
5. Cost Efficiency
By automating the creditworthiness assessment process, AI can reduce the costs associated with manual evaluations. This makes the process more cost-effective for financial institutions, allowing them to allocate resources more efficiently.
Challenges and Ethical Considerations
Despite its many benefits, the use of AI in creditworthiness assessment also raises several challenges and ethical concerns:
1. Data Privacy and Security
AI systems rely on large amounts of personal data, raising concerns about privacy and data security. Financial institutions must ensure that customer data is protected and comply with privacy regulations such as GDPR or CCPA.
2. Bias and Fairness
AI systems can inherit biases present in the data they are trained on. If the data reflects historical discrimination or exclusion, the AI model may perpetuate those biases. Financial institutions must take steps to ensure that AI models are fair and do not inadvertently discriminate against certain groups.
3. Transparency and Accountability
AI models are often criticized for being “black boxes” because it is difficult to understand how they arrive at their conclusions. This lack of transparency can make it harder for borrowers to understand why they were denied credit. Lenders need to ensure that AI systems are explainable and that borrowers have the opportunity to challenge decisions.
4. Regulatory Compliance
The rapid adoption of AI in creditworthiness assessment has outpaced the development of regulatory frameworks. Governments and regulatory bodies need to create clear guidelines to ensure that AI is used ethically and in compliance with existing financial regulations.
The Final Takeaway
AI-driven data analytics is transforming the creditworthiness assessment process, offering more accurate, efficient, and inclusive solutions. By leveraging big data, machine learning, NLP, and alternative data sources, financial institutions can make better-informed decisions and provide more personalized, fair credit offers. However, the adoption of AI must be accompanied by responsible practices, such as ensuring data privacy, minimizing biases, and adhering to regulatory requirements. As AI continues to evolve, its potential to revolutionize the financial industry remains immense, paving the way for a more efficient and equitable credit system.