Mega Models Aren’t the Crux of the Compute Crisis
Every time a new AI model drops—GPT updates, DeepSeek, Gemini—people gawk at the sheer size, the complexity, and increasingly, the compute hunger of these mega-models. The assumption is that these models are defining the resourcing needs of the AI revolution. That assumption is wrong. Yes, large models are compute-hungry. But the biggest strain on AI […] The post Mega Models Aren’t the Crux of the Compute Crisis appeared first on Unite.AI.
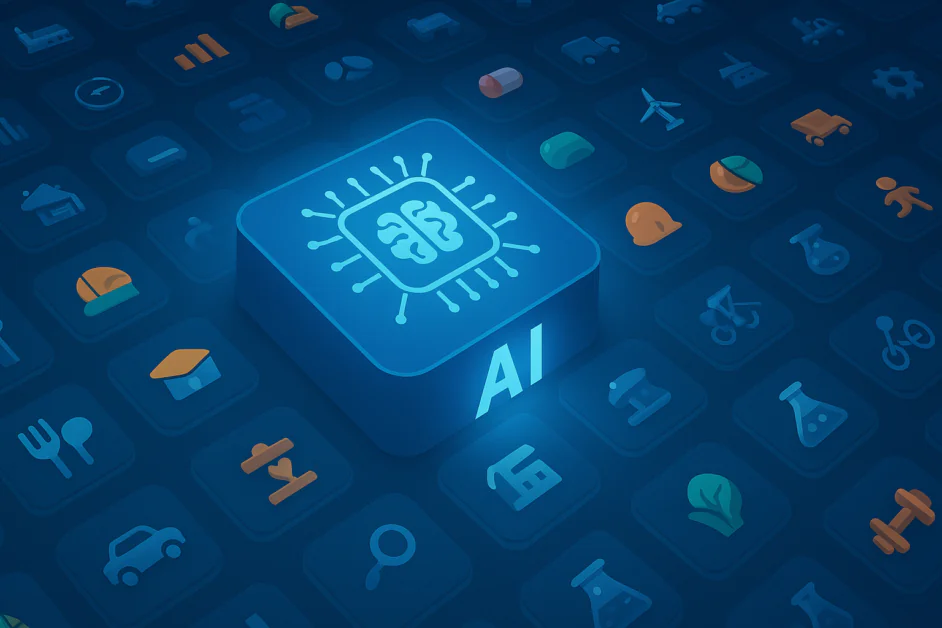
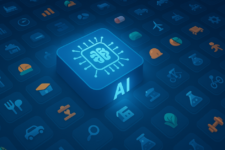
Every time a new AI model drops—GPT updates, DeepSeek, Gemini—people gawk at the sheer size, the complexity, and increasingly, the compute hunger of these mega-models. The assumption is that these models are defining the resourcing needs of the AI revolution.
That assumption is wrong.
Yes, large models are compute-hungry. But the biggest strain on AI infrastructure isn’t coming from a handful of mega-models—it’s coming from the silent proliferation of AI models across industries, each fine-tuned for specific applications, each consuming compute at an unprecedented scale.
Despite the potential winner-takes-all competition developing among the LLMs, the AI landscape at large isn’t centralizing—it’s fragmenting. Every business isn’t just using AI—they’re training, customizing, and deploying private models tailored to their needs. It's the latter situation that will create an infrastructure demand curve that cloud providers, enterprises, and governments aren’t ready for.
We’ve seen this pattern before. Cloud didn’t consolidate IT workloads; it created a sprawling hybrid ecosystem. First, it was server sprawl. Then VM sprawl. Now? AI sprawl. Each wave of computing led to proliferation, not simplification. AI is no different.
AI Sprawl: Why the Future of AI Is a Million Models, Not One
Finance, logistics, cybersecurity, customer service, R&D—each has its own AI model optimized for its own function. Organizations aren’t training one AI model to rule their entire operation. They’re training thousands. That means more training cycles, more compute, more storage demand, and more infrastructure sprawl.
This isn’t theoretical. Even in industries that are traditionally cautious about tech adoption, AI investment is accelerating. A 2024 McKinsey report found that organizations now use AI in an average of three business functions, with manufacturing, supply chain, and product development leading the charge (McKinsey).
Healthcare is a prime example. Navina, a startup that integrates AI into electronic health records to surface clinical insights, just raised $55 million in Series C funding from Goldman Sachs (Business Insider). Energy is no different—industry leaders have launched the Open Power AI Consortium to bring AI optimization to grid and plant operations (Axios).
The Compute Strain No One Is Talking About
AI is already breaking traditional infrastructure models. The assumption that cloud can scale infinitely to support AI growth is dead wrong. AI doesn’t scale like traditional workloads. The demand curve isn’t gradual—it’s exponential, and hyperscalers aren’t keeping up.
- Power Constraints: AI-specific data centers are now being built around power availability, not just network backbones.
- Network Bottlenecks: Hybrid IT environments are becoming unmanageable without automation, which AI workloads will only exacerbate.
- Economic Pressure: AI workloads can consume millions in a single month, creating financial unpredictability.
Data centers already account for 1% of global electricity consumption. In Ireland, they now consume 20% of the national grid, a share expected to rise significantly by 2030 (IEA).
Add to that the looming pressure on GPUs. Bain & Company recently warned that AI growth is setting the stage for a semiconductor shortage, driven by explosive demand for data center-grade chips (Bain).
Meanwhile, AI’s sustainability problem grows. A 2024 analysis in Sustainable Cities and Society warns that widespread adoption of AI in healthcare could substantially increase the sector’s energy consumption and carbon emissions, unless offset by targeted efficiencies (ScienceDirect).
AI Sprawl Is Bigger Than the Market—It’s a Matter of State Power
If you think AI sprawl is a corporate problem, think again. The most significant driver of AI fragmentation isn’t the private sector—it’s governments and military defense agencies, deploying AI at a scale that no hyperscaler or enterprise can match.
The U.S. government alone has deployed AI in over 700 applications across 27 agencies, covering intelligence analysis, logistics, and more (FedTech Magazine).
Canada is investing up to $700 million to expand domestic AI compute capacity, launching a national challenge to bolster sovereign data center infrastructure (Innovation, Science and Economic Development Canada).
And there are rising calls for an “Apollo program” for AI infrastructure—highlighting AI’s elevation from commercial advantage to national imperative (MIT Technology Review).
Military AI will not be efficient, coordinated, or optimized for cost—it will be driven by national security mandates, geopolitical urgency, and the need for closed, sovereign AI systems. Even if enterprises rein in AI sprawl, who’s going to tell governments to slow down?
Because when national security is on the line, no one’s stopping to ask whether the power grid can handle it.
The post Mega Models Aren’t the Crux of the Compute Crisis appeared first on Unite.AI.