Why AI’s Future Rests on Human Partnership
For decades, the promise – and the anxiety – surrounding artificial intelligence has centered on automation. A future where machines handle the mundane, freeing humanity for higher pursuits. But a quiet revolution is underway. The most insightful minds in AI are now arguing that how we build and deploy AI is far more critical than what it can do. The next wave isn’t about replacing us; it’s about amplifying the uniquely human qualities of reasoning, ethical judgment, and contextual understanding – a conscious partnership designed for symbiotic outcomes. This isn’t simply a technical challenge; it’s a fundamental re-evaluation of our relationship with intelligence itself. The Plateau of Automation The early narrative of AI was dominated by efficiency. Faster data processing, more accurate predictions, lower costs. These advancements have undeniably reshaped industries, opening doors to new possibilities. Yet, we’re increasingly encountering an ‘automation plateau’ – a point where simply adding more processing power and sophisticated algorithms yields diminishing returns. The limitations aren’t technical, but systemic. AI models, however complex, are only as reliable as the data they’re trained on. Biased datasets, incomplete information, or a lack of real-world context can lead to skewed results, reinforcing inequalities or generating solutions that miss the mark. The early struggles of facial recognition software, demonstrably less accurate with darker skin tones, serve as a stark example of this inherent flaw. Similarly, risk assessment algorithms in criminal justice can perpetuate societal biases through flawed predictive models. The relentless pursuit of automation often overlooks the crucial role of human judgment, intuition, and ethical considerations. An algorithm can optimize for a specific outcome, but it lacks the nuanced understanding to anticipate unintended consequences or adapt to the unpredictable nature of the real world. It’s akin to a brilliant chess player, masterful in tactical calculation, yet oblivious to the human stakes of the game. The result is a system that is efficient, perhaps, but ultimately brittle and prone to error. Beyond Assistance, Towards Collaboration The emerging alternative is ‘augmented intelligence’ – a framework that prioritizes human-AI collaboration as the primary engine of progress. This isn’t about relegating AI to a supporting role, but reframing it as a powerful tool for amplifying human capabilities. The focus shifts from replacing human decision-making to enhancing it, providing individuals with access to insights and tools previously beyond reach. This shift is already yielding tangible benefits. At the Royal Marsden Hospital, AI systems are assisting radiologists in detecting breast cancer in mammograms. NHS reports indicate these systems reduce both false positives and false negatives, allowing radiologists to concentrate on complex cases and improve diagnostic accuracy. The AI doesn’t deliver the final diagnosis; it flags areas of concern, prompting a more thorough review by a skilled professional. The same principle applies to creative fields. AI tools like Midjourney and DALL-E 2 can generate a multitude of visual concepts and explore diverse aesthetic possibilities. However, it’s the human designer who provides the overarching vision, selects the most compelling ideas, and imbues the final product with meaning and emotional resonance. The AI acts as a tireless brainstorming partner, a generator of variations, but the human remains the curator and storyteller. The most compelling outcomes arise from a synergistic partnership, where human creativity and AI-powered exploration complement each other. The Foundation of Trust The effectiveness of any AI system is inextricably linked to the quality of its training data. The age-old computing adage – “garbage in, garbage out” – is more relevant than ever. But ensuring data quality isn’t simply about collecting more data; it demands a fundamentally different approach to data management, one that prioritizes human oversight and contextual understanding. Current reliance on automated data collection and labeling is often prone to errors and biases. While crowdsourcing offers scalability, it can introduce inconsistencies and inaccuracies. Algorithms designed to automatically cleanse and pre-process data can inadvertently strip away valuable nuances or introduce unintended distortions. OpenStreetMap (OSM) offers a compelling alternative. A collaborative mapping project built by a global community of volunteers, OSM demonstrates the power of human curation and validation. Automated tools initially extract data from satellite imagery, but accuracy and completeness are maintained through the collective efforts of contributors who meticulously verify and update information. This highlights the importance of integrating human expertise into the data pipeline – validating, annot
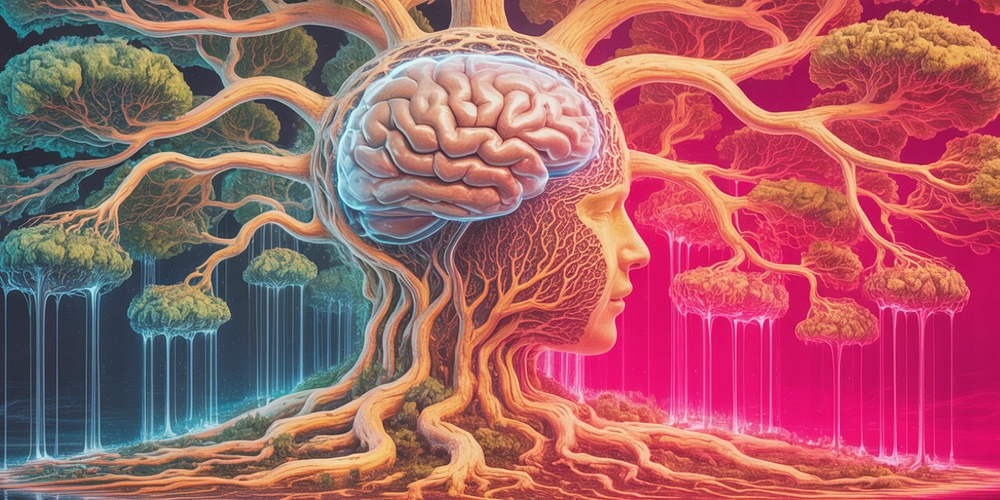
For decades, the promise – and the anxiety – surrounding artificial intelligence has centered on automation. A future where machines handle the mundane, freeing humanity for higher pursuits. But a quiet revolution is underway. The most insightful minds in AI are now arguing that how we build and deploy AI is far more critical than what it can do. The next wave isn’t about replacing us; it’s about amplifying the uniquely human qualities of reasoning, ethical judgment, and contextual understanding – a conscious partnership designed for symbiotic outcomes. This isn’t simply a technical challenge; it’s a fundamental re-evaluation of our relationship with intelligence itself.
The Plateau of Automation
The early narrative of AI was dominated by efficiency. Faster data processing, more accurate predictions, lower costs. These advancements have undeniably reshaped industries, opening doors to new possibilities. Yet, we’re increasingly encountering an ‘automation plateau’ – a point where simply adding more processing power and sophisticated algorithms yields diminishing returns.
The limitations aren’t technical, but systemic. AI models, however complex, are only as reliable as the data they’re trained on. Biased datasets, incomplete information, or a lack of real-world context can lead to skewed results, reinforcing inequalities or generating solutions that miss the mark. The early struggles of facial recognition software, demonstrably less accurate with darker skin tones, serve as a stark example of this inherent flaw. Similarly, risk assessment algorithms in criminal justice can perpetuate societal biases through flawed predictive models.
The relentless pursuit of automation often overlooks the crucial role of human judgment, intuition, and ethical considerations. An algorithm can optimize for a specific outcome, but it lacks the nuanced understanding to anticipate unintended consequences or adapt to the unpredictable nature of the real world. It’s akin to a brilliant chess player, masterful in tactical calculation, yet oblivious to the human stakes of the game. The result is a system that is efficient, perhaps, but ultimately brittle and prone to error.
Beyond Assistance, Towards Collaboration
The emerging alternative is ‘augmented intelligence’ – a framework that prioritizes human-AI collaboration as the primary engine of progress. This isn’t about relegating AI to a supporting role, but reframing it as a powerful tool for amplifying human capabilities. The focus shifts from replacing human decision-making to enhancing it, providing individuals with access to insights and tools previously beyond reach.
This shift is already yielding tangible benefits. At the Royal Marsden Hospital, AI systems are assisting radiologists in detecting breast cancer in mammograms. NHS reports indicate these systems reduce both false positives and false negatives, allowing radiologists to concentrate on complex cases and improve diagnostic accuracy. The AI doesn’t deliver the final diagnosis; it flags areas of concern, prompting a more thorough review by a skilled professional.
The same principle applies to creative fields. AI tools like Midjourney and DALL-E 2 can generate a multitude of visual concepts and explore diverse aesthetic possibilities. However, it’s the human designer who provides the overarching vision, selects the most compelling ideas, and imbues the final product with meaning and emotional resonance. The AI acts as a tireless brainstorming partner, a generator of variations, but the human remains the curator and storyteller. The most compelling outcomes arise from a synergistic partnership, where human creativity and AI-powered exploration complement each other.
The Foundation of Trust
The effectiveness of any AI system is inextricably linked to the quality of its training data. The age-old computing adage – “garbage in, garbage out” – is more relevant than ever. But ensuring data quality isn’t simply about collecting more data; it demands a fundamentally different approach to data management, one that prioritizes human oversight and contextual understanding.
Current reliance on automated data collection and labeling is often prone to errors and biases. While crowdsourcing offers scalability, it can introduce inconsistencies and inaccuracies. Algorithms designed to automatically cleanse and pre-process data can inadvertently strip away valuable nuances or introduce unintended distortions.
OpenStreetMap (OSM) offers a compelling alternative. A collaborative mapping project built by a global community of volunteers, OSM demonstrates the power of human curation and validation. Automated tools initially extract data from satellite imagery, but accuracy and completeness are maintained through the collective efforts of contributors who meticulously verify and update information. This highlights the importance of integrating human expertise into the data pipeline – validating, annotating, and enriching the data with contextual understanding.
Unlocking the Black Box
A significant barrier to widespread AI adoption is a lack of trust. Many are understandably hesitant to entrust important decisions to algorithms they don’t understand. This is particularly problematic with ‘black box’ AI systems, where the decision-making process is opaque and inscrutable.
Addressing this requires a shift towards ‘explainable AI’ (XAI) – developing algorithms that can provide clear, understandable explanations for their decisions. This isn’t about revealing the algorithm’s inner workings in minute detail, but rather providing a high-level overview of the factors that influenced the outcome.
Transparency is equally crucial. Organizations deploying AI systems should be open about their use of the technology, the data they’re collecting, and the potential biases that may be present. This is gaining legislative momentum. The proposed Algorithmic Accountability Act in the United States, while not yet law, signals a growing demand for greater transparency and accountability in AI deployment.
Addressing Systemic Risks and Unintended Consequences
Mitigating bias in AI is a crucial step, but it’s not enough. We must also address the broader systemic risks and social impacts of this technology. AI has the potential to exacerbate existing inequalities, automate jobs, and erode privacy. Addressing these challenges requires a holistic and proactive approach.
Consider the growing use of automated hiring tools. While promising to streamline recruitment and reduce bias, these tools have often been shown to perpetuate existing inequalities. Amazon famously scrapped an AI recruitment tool in 2018 after it was discovered to be biased against female candidates. The algorithm, trained on historical hiring data, had learned to associate male applicants with success, effectively discriminating against women. This underscores the importance of critically examining the societal context in which AI systems are deployed, not just addressing bias in the data itself.
Automation, even with the best intentions, can entrench existing power structures and exacerbate societal inequalities if not carefully considered.
Continuous Learning and Human-in-the-Loop Systems
The ideal human-AI collaboration isn’t a static relationship, but a dynamic, iterative process of continuous learning and adaptation. AI systems should be designed to learn from human feedback, incorporating new information and refining their models over time.
This requires establishing ‘symbiotic loops’ – feedback mechanisms that allow humans and AI to continuously improve each other’s performance.
GitHub Copilot, a coding assistant powered by OpenAI’s Codex model, exemplifies this principle. Copilot learns from the code its users write, suggesting lines of code, completing functions, and even generating entire code blocks based on natural language prompts. Crucially, the system doesn’t simply output code blindly; it presents multiple suggestions, allowing developers to choose the best option and provide feedback on the quality of the suggestions. This ongoing interaction between human and AI creates a virtuous cycle of learning and improvement, resulting in a more powerful and effective coding assistant.
A Deliberate Path Forward
The future of AI isn’t about creating intelligent machines that surpass human capabilities. It’s about building a symbiotic relationship where humans and AI work together to achieve outcomes that neither could accomplish alone. It’s about harnessing the power of AI to amplify human creativity, enhance our understanding of the world, and solve some of the most pressing challenges facing humanity.
This requires a fundamental shift in mindset – from a focus on automation to a focus on augmentation, from a pursuit of efficiency to a commitment to ethical and responsible innovation.
A counter-movement to the relentless pursuit of faster, more complex AI is gaining traction. This “Slow AI” philosophy advocates for a more deliberate and thoughtful approach to AI development, prioritizing transparency, accountability, and human control. It echoes the “Slow Food” movement, championing quality and sustainability over speed and efficiency. Researchers like Kate Crawford, author of Atlas of AI, advocate for critically examining the planetary costs of artificial intelligence.
Ultimately, the goal isn’t simply to create more intelligent machines, but to create a more intelligent world – a world where human potential is unleashed, and where technology serves humanity’s highest aspirations. This symbiotic algorithm, crafted with intention and guided by human values, represents not a threat, but an opportunity for profound and positive change.
References & Further Information:
- NHS. (n.d.). AI in diagnosis, imaging and pathology. Retrieved from https://www.nhs.uk/innovation-research/artificial-intelligence/ai-in-diagnosis-imaging-and-pathology
- OpenStreetMap. (n.d.). About. Retrieved from https://www.openstreetmap.org/about
- GitHub Copilot. (n.d.). AI pair programmer. Retrieved from https://github.com/features/copilot
- O’Neil, C. (2016). Weapons of math destruction: How big data increases inequality and threatens democracy. Crown.
- Crawford, K. (2021). Atlas of AI: Power, Politics, and the Planetary Costs of Artificial Intelligence. Yale University Press.
- Algorithmic Accountability Act. (n.d.). Retrieved from https://www.govtrack.us/congress/bills/117/s3953 (example bill information – specific legislation may vary).