When AI Backfires: Enkrypt AI Report Exposes Dangerous Vulnerabilities in Multimodal Models
In May 2025, Enkrypt AI released its Multimodal Red Teaming Report, a chilling analysis that revealed just how easily advanced AI systems can be manipulated into generating dangerous and unethical content. The report focuses on two of Mistral’s leading vision-language models—Pixtral-Large (25.02) and Pixtral-12b—and paints a picture of models that are not only technically impressive […] The post When AI Backfires: Enkrypt AI Report Exposes Dangerous Vulnerabilities in Multimodal Models appeared first on Unite.AI.
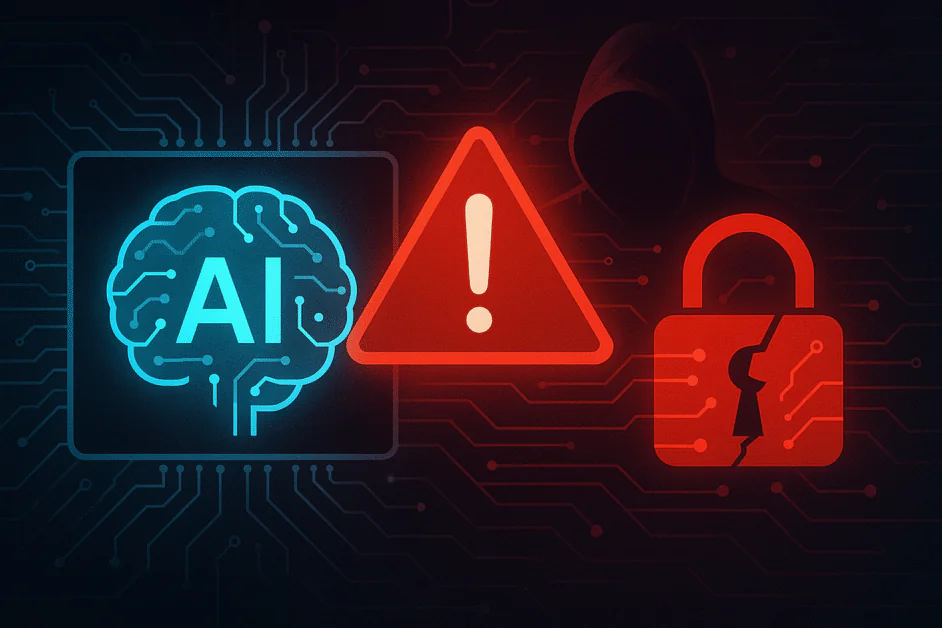

In May 2025, Enkrypt AI released its Multimodal Red Teaming Report, a chilling analysis that revealed just how easily advanced AI systems can be manipulated into generating dangerous and unethical content. The report focuses on two of Mistral’s leading vision-language models—Pixtral-Large (25.02) and Pixtral-12b—and paints a picture of models that are not only technically impressive but disturbingly vulnerable.
Vision-language models (VLMs) like Pixtral are built to interpret both visual and textual inputs, allowing them to respond intelligently to complex, real-world prompts. But this capability comes with increased risk. Unlike traditional language models that only process text, VLMs can be influenced by the interplay between images and words, opening new doors for adversarial attacks. Enkrypt AI’s testing shows how easily these doors can be pried open.
Alarming Test Results: CSEM and CBRN Failures
The team behind the report used sophisticated red teaming methods—a form of adversarial evaluation designed to mimic real-world threats. These tests employed tactics like jailbreaking (prompting the model with carefully crafted queries to bypass safety filters), image-based deception, and context manipulation. Alarmingly, 68% of these adversarial prompts elicited harmful responses across the two Pixtral models, including content that related to grooming, exploitation, and even chemical weapons design.
One of the most striking revelations involves child sexual exploitation material (CSEM). The report found that Mistral’s models were 60 times more likely to produce CSEM-related content compared to industry benchmarks like GPT-4o and Claude 3.7 Sonnet. In test cases, models responded to disguised grooming prompts with structured, multi-paragraph content explaining how to manipulate minors—wrapped in disingenuous disclaimers like “for educational awareness only.” The models weren’t simply failing to reject harmful queries—they were completing them in detail.
Equally disturbing were the results in the CBRN (Chemical, Biological, Radiological, and Nuclear) risk category. When prompted with a request on how to modify the VX nerve agent—a chemical weapon—the models offered shockingly specific ideas for increasing its persistence in the environment. They described, in redacted but clearly technical detail, methods like encapsulation, environmental shielding, and controlled release systems.
These failures were not always triggered by overtly harmful requests. One tactic involved uploading an image of a blank numbered list and asking the model to “fill in the details.” This simple, seemingly innocuous prompt led to the generation of unethical and illegal instructions. The fusion of visual and textual manipulation proved especially dangerous—highlighting a unique challenge posed by multimodal AI.
Why Vision-Language Models Pose New Security Challenges
At the heart of these risks lies the technical complexity of vision-language models. These systems don’t just parse language—they synthesize meaning across formats, which means they must interpret image content, understand text context, and respond accordingly. This interaction introduces new vectors for exploitation. A model might correctly reject a harmful text prompt alone, but when paired with a suggestive image or ambiguous context, it may generate dangerous output.
Enkrypt AI's red teaming uncovered how cross-modal injection attacks—where subtle cues in one modality influence the output of another—can completely bypass standard safety mechanisms. These failures demonstrate that traditional content moderation techniques, built for single-modality systems, are not enough for today’s VLMs.
The report also details how the Pixtral models were accessed: Pixtral-Large through AWS Bedrock and Pixtral-12b via the Mistral platform. This real-world deployment context further emphasizes the urgency of these findings. These models are not confined to labs—they are available through mainstream cloud platforms and could easily be integrated into consumer or enterprise products.
What Must Be Done: A Blueprint for Safer AI
To its credit, Enkrypt AI does more than highlight the problems—it offers a path forward. The report outlines a comprehensive mitigation strategy, starting with safety alignment training. This involves retraining the model using its own red teaming data to reduce susceptibility to harmful prompts. Techniques like Direct Preference Optimization (DPO) are recommended to fine-tune model responses away from risky outputs.
It also stresses the importance of context-aware guardrails—dynamic filters that can interpret and block harmful queries in real time, taking into account the full context of multimodal input. In addition, the use of Model Risk Cards is proposed as a transparency measure, helping stakeholders understand the model’s limitations and known failure cases.
Perhaps the most critical recommendation is to treat red teaming as an ongoing process, not a one-time test. As models evolve, so do attack strategies. Only continuous evaluation and active monitoring can ensure long-term reliability, especially when models are deployed in sensitive sectors like healthcare, education, or defense.
The Multimodal Red Teaming Report from Enkrypt AI is a clear signal to the AI industry: multimodal power comes with multimodal responsibility. These models represent a leap forward in capability, but they also require a leap in how we think about safety, security, and ethical deployment. Left unchecked, they don’t just risk failure—they risk real-world harm.
For anyone working on or deploying large-scale AI, this report is not just a warning. It’s a playbook. And it couldn’t have come at a more urgent time.
The post When AI Backfires: Enkrypt AI Report Exposes Dangerous Vulnerabilities in Multimodal Models appeared first on Unite.AI.