Transforming Service Operations with AI-Driven Observability and ITSM Integration
In today’s hyper-connected, technology-driven world, enterprises face unprecedented demands to deliver seamless digital experiences while maintaining robust IT operations. From financial institutions processing millions of transactions daily to global retailers managing e-commerce platforms and telecommunications giants ensuring uninterrupted connectivity, the stakes have never been higher. These organizations operate in hybrid and multi-cloud environments, where complexity, scale, and speed define the operational landscape. Downtime, latency, or security breaches can result in significant revenue losses, eroded customer trust, and regulatory penalties. To meet these challenges, enterprises are increasingly adopting AI-powered observability platforms integrated with IT Service Management (ITSM) tools. These solutions harness advanced automation, predictive analytics, and Generative AI to revolutionize how IT teams monitor, manage, and optimize services. By transitioning from siloed, reactive approaches to unified, proactive strategies, organizations can minimize disruptions, accelerate incident resolution, and elevate customer satisfaction. This article explores how AI-driven observability and ITSM integration are transforming service operations, offering actionable insights, real-world applications, and a glimpse into the future of IT. The Shift Toward AI-Driven Observability The Limitations of Traditional Monitoring Traditional IT monitoring tools, designed for static, on-premises infrastructures, are ill-equipped to handle the dynamic nature of modern cloud-native environments. These legacy systems rely heavily on predefined thresholds and manual intervention, struggling to process the vast volumes of telemetry data-logs, metrics, and traces-generated by microservices, APIs, and distributed applications. For example, a global retailer with thousands of microservices powering its online platform might generate terabytes of data daily, making it impossible for IT teams to manually sift through alerts and pinpoint issues in real time. This reactive approach often leads to prolonged downtime, frustrated customers, and overburdened staff. The Power of AI-Driven Observability AI-driven observability platforms address these shortcomings by leveraging machine learning (ML), real-time data processing, and intelligent anomaly detection. Unlike traditional tools that merely report what’s happening, observability platforms provide a holistic view of system health, answering the critical “why” behind performance issues. For instance, if a telecommunications provider experiences a spike in latency, an AI-driven platform can analyze patterns across network logs, application metrics, and user behavior to detect anomalies-such as a failing API endpoint—and suggest root causes without human guesswork. These platforms also incorporate predictive insights, enabling IT teams to anticipate problems before they escalate. By automating root cause analysis (RCA), they reduce mean time to detection (MTTD) and mean time to resolution (MTTR), ensuring higher uptime and reliability. A financial services firm, for example, could use AI-driven observability to identify a misconfigured payment gateway before it disrupts transactions, saving millions in potential losses. Traditional monitoring is ineffective for modern cloud architectures: It lacks the scalability and intelligence needed for dynamic environments. AI-driven observability enables real-time anomaly detection and predictive insights: It shifts IT from firefighting to prevention. Automated root cause analysis accelerates incident resolution and minimizes downtime: It empowers teams with actionable intelligence. Integrating Observability with ITSM for Seamless Operations Bridging the Gap Between Insight and Action AI-driven observability alone provides valuable insights, but its full potential is realized when integrated with ITSM platforms like ServiceNow, BMC Helix, or Jira Service Management. This integration creates a closed-loop system where monitoring data feeds directly into incident response workflows. For example, when an observability platform detects a server overload in a retailer’s e-commerce stack, it can automatically generate a ticket in ServiceNow, assign it to the appropriate team, and attach relevant diagnostic data-all within seconds. AI-Powered Automation in ITSM The integration leverages AI to automate repetitive tasks, such as ticket categorization, prioritization, and escalation. By analyzing business impact e.g., whether an issue affects a critical customer-facing application versus an internal tool-AI ensures resources are allocated efficiently. In a real-world scenario, a telecom provider might use this capability to prioritize a network outage affecting thousands of users over a minor internal dashboard glitch, minimizing customer impact. Moreover, AI-driven ITSM workflows can trigger predefined remediation scripts
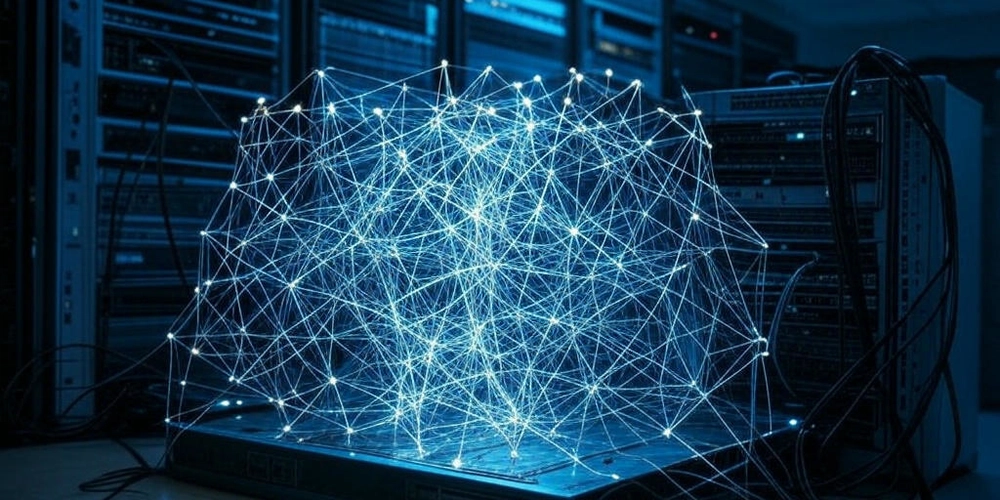
In today’s hyper-connected, technology-driven world, enterprises face unprecedented demands to deliver seamless digital experiences while maintaining robust IT operations. From financial institutions processing millions of transactions daily to global retailers managing e-commerce platforms and telecommunications giants ensuring uninterrupted connectivity, the stakes have never been higher. These organizations operate in hybrid and multi-cloud environments, where complexity, scale, and speed define the operational landscape. Downtime, latency, or security breaches can result in significant revenue losses, eroded customer trust, and regulatory penalties.
To meet these challenges, enterprises are increasingly adopting AI-powered observability platforms integrated with IT Service Management (ITSM) tools. These solutions harness advanced automation, predictive analytics, and Generative AI to revolutionize how IT teams monitor, manage, and optimize services. By transitioning from siloed, reactive approaches to unified, proactive strategies, organizations can minimize disruptions, accelerate incident resolution, and elevate customer satisfaction. This article explores how AI-driven observability and ITSM integration are transforming service operations, offering actionable insights, real-world applications, and a glimpse into the future of IT.
The Shift Toward AI-Driven Observability
The Limitations of Traditional Monitoring
Traditional IT monitoring tools, designed for static, on-premises infrastructures, are ill-equipped to handle the dynamic nature of modern cloud-native environments. These legacy systems rely heavily on predefined thresholds and manual intervention, struggling to process the vast volumes of telemetry data-logs, metrics, and traces-generated by microservices, APIs, and distributed applications. For example, a global retailer with thousands of microservices powering its online platform might generate terabytes of data daily, making it impossible for IT teams to manually sift through alerts and pinpoint issues in real time. This reactive approach often leads to prolonged downtime, frustrated customers, and overburdened staff.
The Power of AI-Driven Observability
AI-driven observability platforms address these shortcomings by leveraging machine learning (ML), real-time data processing, and intelligent anomaly detection. Unlike traditional tools that merely report what’s happening, observability platforms provide a holistic view of system health, answering the critical “why” behind performance issues. For instance, if a telecommunications provider experiences a spike in latency, an AI-driven platform can analyze patterns across network logs, application metrics, and user behavior to detect anomalies-such as a failing API endpoint—and suggest root causes without human guesswork.
These platforms also incorporate predictive insights, enabling IT teams to anticipate problems before they escalate. By automating root cause analysis (RCA), they reduce mean time to detection (MTTD) and mean time to resolution (MTTR), ensuring higher uptime and reliability. A financial services firm, for example, could use AI-driven observability to identify a misconfigured payment gateway before it disrupts transactions, saving millions in potential losses.
- Traditional monitoring is ineffective for modern cloud architectures: It lacks the scalability and intelligence needed for dynamic environments.
- AI-driven observability enables real-time anomaly detection and predictive insights: It shifts IT from firefighting to prevention.
- Automated root cause analysis accelerates incident resolution and minimizes downtime: It empowers teams with actionable intelligence.
Integrating Observability with ITSM for Seamless Operations
Bridging the Gap Between Insight and Action
AI-driven observability alone provides valuable insights, but its full potential is realized when integrated with ITSM platforms like ServiceNow, BMC Helix, or Jira Service Management. This integration creates a closed-loop system where monitoring data feeds directly into incident response workflows. For example, when an observability platform detects a server overload in a retailer’s e-commerce stack, it can automatically generate a ticket in ServiceNow, assign it to the appropriate team, and attach relevant diagnostic data-all within seconds.
AI-Powered Automation in ITSM
The integration leverages AI to automate repetitive tasks, such as ticket categorization, prioritization, and escalation. By analyzing business impact e.g., whether an issue affects a critical customer-facing application versus an internal tool-AI ensures resources are allocated efficiently. In a real-world scenario, a telecom provider might use this capability to prioritize a network outage affecting thousands of users over a minor internal dashboard glitch, minimizing customer impact.
Moreover, AI-driven ITSM workflows can trigger predefined remediation scripts. If a database performance issue is detected, the system might automatically restart the service or allocate additional resources, reducing manual intervention and human error. This seamless collaboration between observability and ITSM transforms IT operations into a proactive, business-aligned function.
Challenges and Considerations
While integration offers significant benefits, it’s not without challenges. Enterprises must ensure data consistency between observability and ITSM systems, address potential latency in real-time workflows, and train staff to trust AI-driven decisions. Overcoming these hurdles requires robust APIs, standardized data formats, and a cultural shift toward automation.
- ITSM integration bridges observability insights with incident response: It creates a unified operational ecosystem.
- AI-powered automation reduces manual intervention and enhances efficiency: It streamlines processes and minimizes errors.
- Incident prioritization ensures optimal resource allocation and service continuity: It aligns IT efforts with business priorities.
Generative AI: Transforming Incident Management and Resolution
The Rise of Intelligent Assistance
Generative AI, exemplified by models like those powering chatbots or virtual assistants, is redefining incident management. These tools analyze historical incident data, system logs, and contextual information to provide dynamic, tailored remediation recommendations. For instance, if a financial institution’s trading platform experiences a connectivity failure, a Generative AI assistant might suggest specific network configurations or rollback procedures based on past resolutions, accelerating recovery.
Automating Fixes in Real Time
Beyond recommendations, Generative AI can execute automated fixes. Imagine a scenario where a retailer’s checkout system crashes during a peak sales event like Black Friday. An AI-powered assistant could detect the issue, identify it as a memory leak, and trigger a container restart-all without human input. This capability slashes MTTR and ensures service continuity during critical moments.
Enhancing Knowledge Management
Generative AI also transforms knowledge management by creating real-time documentation. As incidents are resolved, the AI can generate detailed reports, update FAQs, and produce troubleshooting guides, ensuring institutional knowledge is preserved and accessible. For example, a telecom IT team resolving a recurring billing issue could rely on AI-generated guides to prevent future occurrences, reducing ticket volume over time.
Limitations and Ethical Considerations
While powerful, Generative AI isn’t infallible. It requires high-quality training data to avoid biased or inaccurate recommendations. Enterprises must also address ethical concerns, such as over-reliance on automation, ensuring human oversight remains in place for critical decisions.
- Generative AI enhances incident resolution with intelligent recommendations: It leverages historical data for contextual solutions.
- AI-powered assistants reduce MTTR and improve service reliability: They enable rapid, automated responses.
- Dynamic knowledge management ensures up-to-date remediation insights: It builds a self-improving knowledge base.
Predictive Analytics: Preventing Issues Before They Impact Customers
From Reactive to Proactive
Predictive analytics, a cornerstone of AI-driven observability, empowers organizations to foresee and prevent disruptions. By analyzing historical trends, system performance metrics, and anomaly patterns, AI models can predict failures with remarkable accuracy. For instance, a retailer might use predictive analytics to forecast server overload during a holiday sale, preemptively scaling resources to avoid crashes.
Real-World Applications
In telecommunications, predictive analytics can identify degrading network equipment before it fails, triggering maintenance workflows to replace hardware. Similarly, a financial services firm could detect unusual transaction patterns indicative of a looming system bottleneck, addressing it before customers notice delays. These proactive measures enhance service resilience and customer trust.
Measuring Success
The impact of predictive analytics is quantifiable: reduced incident frequency, lower operational costs, and higher Net Promoter Scores (NPS). Enterprises adopting this approach report up to 30% fewer critical incidents, according to industry studies, underscoring its value in a competitive market.
- Predictive analytics prevents issues before they impact end users: It shifts IT to a preventive mindset.
- AI-driven forecasting enhances service resilience and operational efficiency: It mitigates risks proactively.
- Preventive measures minimize downtime and improve customer satisfaction: They ensure uninterrupted experiences.
Automated Remediation: The Future of Self-Healing IT Operations
The Self-Healing Vision
Automated remediation takes AI-driven observability and ITSM integration to the next level, enabling self-healing IT operations. When an issue is detected—say, a memory spike in a cloud application-the system can automatically execute a predefined script to resolve it, such as reallocating resources or applying a patch. This hands-free approach minimizes downtime and frees IT teams for strategic tasks.
Real-World Examples
Consider a global retailer using Kubernetes for its e-commerce platform. If a pod fails, an AI-driven workflow could detect the anomaly, redeploy the pod, and update the ITSM ticket-all in under a minute. Similarly, a telecom provider might automate patch deployment for vulnerable network devices, ensuring security without manual effort.
Benefits and Scalability
Self-healing operations reduce the operational burden, enhance system reliability, and scale effortlessly with growing infrastructure. However, they require careful design to avoid unintended consequences, such as over-correcting minor issues or conflicting with manual processes.
- Self-healing IT operations reduce manual intervention and enhance resilience: They automate routine fixes.
- Automated remediation minimizes downtime and operational disruptions: It ensures continuous service delivery.
- AI-driven workflows ensure seamless issue detection and resolution: They scale with enterprise needs.
Enhancing Customer Experience with AI-Driven IT Operations
The Customer-Centric Imperative
In a digital-first world, customer experience (CX) hinges on IT performance. AI-driven observability and ITSM integration ensure applications run smoothly, incidents resolve quickly, and services remain proactive. For example, a streaming service using AI to monitor video delivery can optimize bitrate in real time, preventing buffering and retaining viewers.
Tangible Outcomes
Faster resolution times translate to fewer abandoned carts for retailers, higher transaction success rates for banks, and better call quality for telecoms. By reducing latency and ensuring availability, enterprises boost customer loyalty and revenue. A 2024 Gartner report notes that companies prioritizing IT-driven CX see a 20% increase in customer retention.
- AI-driven IT operations ensure seamless digital experiences for customers: They optimize touchpoints.
- Faster incident resolution and proactive optimization enhance service reliability: They build trust.
- Improved application performance translates to higher customer satisfaction: It drives business growth.
The Role of AIOps in Modern IT Service Management
Defining AIOps
AIOps combines AI, ML, and big data to enhance ITSM. It filters out noise from thousands of alerts, correlates events across systems, and classifies incidents automatically. For instance, an AIOps platform might link a database slowdown to a recent deployment, sparing IT teams hours of manual investigation.
Hyper-Automation in Action
Integrated with observability and ITSM, AIOps enables hyper-automation—end-to-end process optimization. A retailer could use AIOps to automate inventory system checks, ensuring stock levels sync with online orders without human oversight.
- AIOps enhances ITSM with intelligent automation and noise reduction: It focuses teams on critical issues.
- Automated event correlation streamlines incident classification and resolution: It cuts through complexity.
- Hyper-automation drives efficiency and operational excellence: It scales IT capabilities.
The Future of IT Operations: AI, Automation, and Observability
Emerging Trends
The convergence of AI, automation, and observability is poised to redefine IT. Edge computing, 5G, and IoT will generate even more data, necessitating smarter platforms. Quantum computing could further accelerate predictive models, while augmented reality might enhance troubleshooting for field teams.
Strategic Investments
Enterprises must adopt scalable, vendor-agnostic solutions that integrate seamlessly with existing stacks. Training programs to upskill staff in AI and automation will also be critical to maximizing ROI.
- AI, automation, and observability are reshaping IT operations: They drive the next wave of innovation.
- Enterprises must invest in scalable AI-driven platforms for long-term success: They ensure competitiveness.
- Future-proofing IT environments enhances agility and service excellence: It prepares organizations for tomorrow.
AI-powered observability and ITSM integration are no longer optional-they’re imperatives for enterprises seeking to thrive in a digital-first era. By harnessing Generative AI, predictive analytics, and automated remediation, organizations can transform IT from a cost center into a strategic enabler. This shift ensures seamless service delivery, delighted customers, and resilient operations, positioning businesses for sustained success. As technology evolves, AI-driven IT operations will continue to innovate, paving the way for autonomous, intelligent enterprises.
In Summary
AI-driven IT operations offer a range of transformative benefits that empower enterprises to optimize service delivery and enhance customer experiences. AI-driven observability provides real-time insights and anomaly detection, enabling IT teams to monitor complex systems effectively. ITSM integration streamlines operations through automated workflows and improved incident management, ensuring seamless coordination between monitoring and resolution processes. Generative AI introduces intelligent recommendations and self-healing capabilities, allowing for faster, automated fixes and dynamic knowledge management. Predictive analytics plays a crucial role in issue prevention and proactive remediation by forecasting potential disruptions before they impact users. Automated remediation reduces mean time to resolution (MTTR) and enhances service reliability by enabling self-healing IT operations with minimal human intervention. Finally, AIOps integration cuts through operational complexity with noise reduction and intelligent incident handling, driving efficiency and precision. By embracing these technologies, enterprises can elevate service operations, foster innovation, and deliver exceptional customer experiences in an increasingly competitive landscape.