SOTA Models for Web Devs: Practical Use Cases
You've likely encountered discussions about state-of-the-art (SOTA) AI models. If you're curious about what SOTA is and how it works, check out this article. The examples I’m sharing below are just starting points to give you an idea of how SOTA models can fit into your web development workflow. They’re simple directions to help you get started, but if you really want to get the most out of these tools, I’d suggest exploring more advanced use cases on your own. 1. OpenAI's GPT-3.5 & GPT-4 (Think of it as a Quick Code Helper): If you need to create the basic structure for a website contact form, instead of writing all the HTML for the labels, input fields (like name, email, message), and the submit button from scratch, you can tell GPT-3.5 or GPT-4 something like: "Create the HTML for a simple contact form with fields for name, email, and a text area for the message, include labels for each, and a submit button." It can then generate that basic HTML structure for you in seconds, saving you the initial typing. Similarly, if you encounter a JavaScript error in your browser's console, instead of spending a lot of time trying to figure out the cause, you can paste the error message and the line of code where it occurred into the AI and ask, "What does this JavaScript error mean and what could be causing it?" It might give you a few common reasons and suggest things to check in your code. 2. Anthropic's Claude (For Understanding and Explaining Code): Imagine you've inherited a project with a complex JavaScript function that handles user authentication, and it's not well-documented. Instead of spending hours tracing through the code, you could give Claude the entire function and ask, "Explain what this JavaScript function does in simple terms." Claude can then break down the logic step by step, explaining the different parts of the authentication process. Another example: if you need to explain to a client how the website's new image optimization feature works, you could provide Claude with the technical details and ask it to "Explain this image optimization process in a few short sentences that a non-technical person can understand." 3. Google DeepMind's AlphaDev (Behind the Scenes Performance): You don't directly use AlphaDev, but think of it this way: when the JavaScript engine in your browser or the Node.js runtime you use on the backend gets updated, it might include more efficient ways of sorting lists or handling data structures – improvements that could have originated from research like AlphaDev's. So, by simply keeping your browser updated or using the latest stable version of Node.js, your web applications can benefit from these under-the-hood performance enhancements without you needing to write any different code. 4. Open-source Models like AutoGPT (Your Automation Assistant): Let's say you're starting a new React project and need to look up the best practices for folder structure and component organization in 2025. You could instruct AutoGPT with a goal like: "Research the recommended folder structure and component organization patterns for a medium-sized React application in 2025. Provide a summary of the top 3 approaches and links to relevant articles." AutoGPT would then autonomously browse the web, gather information, and present you with a summary, saving you the time of manually searching and compiling this information yourself. 5. Google's Gemini Models (Especially 2.5 Flash) (For Tough Problems): Suppose you're experiencing significant performance issues on a complex single-page application built with a modern framework like Vue.js, and you're not sure where the bottleneck is. You could provide Gemini with performance profiling data (like output from your browser's developer tools) and relevant sections of your Vue.js component code, then ask, "Based on this performance data and component logic, what are the most likely causes of the performance issues and what are some potential optimization strategies I could try in Vue.js?" Gemini's advanced reasoning could help you pinpoint areas in your code or architecture that need attention and suggest specific ways to improve performance. So, are you already using some of these in your dev workflow? I'd love to hear how you're using them or what tasks you think they could help with. Drop your thoughts or examples in the comments.
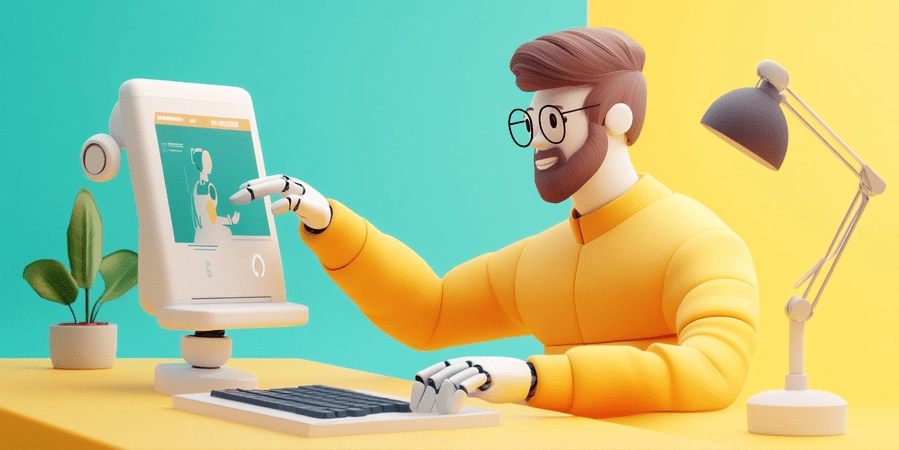
You've likely encountered discussions about state-of-the-art (SOTA) AI models. If you're curious about what SOTA is and how it works, check out this article.
The examples I’m sharing below are just starting points to give you an idea of how SOTA models can fit into your web development workflow. They’re simple directions to help you get started, but if you really want to get the most out of these tools, I’d suggest exploring more advanced use cases on your own.
1. OpenAI's GPT-3.5 & GPT-4 (Think of it as a Quick Code Helper):
If you need to create the basic structure for a website contact form, instead of writing all the HTML for the labels, input fields (like name, email, message), and the submit button from scratch, you can tell GPT-3.5 or GPT-4 something like: "Create the HTML for a simple contact form with fields for name, email, and a text area for the message, include labels for each, and a submit button." It can then generate that basic HTML structure for you in seconds, saving you the initial typing. Similarly, if you encounter a JavaScript error in your browser's console, instead of spending a lot of time trying to figure out the cause, you can paste the error message and the line of code where it occurred into the AI and ask, "What does this JavaScript error mean and what could be causing it?" It might give you a few common reasons and suggest things to check in your code.
2. Anthropic's Claude (For Understanding and Explaining Code):
Imagine you've inherited a project with a complex JavaScript function that handles user authentication, and it's not well-documented. Instead of spending hours tracing through the code, you could give Claude the entire function and ask, "Explain what this JavaScript function does in simple terms." Claude can then break down the logic step by step, explaining the different parts of the authentication process. Another example: if you need to explain to a client how the website's new image optimization feature works, you could provide Claude with the technical details and ask it to "Explain this image optimization process in a few short sentences that a non-technical person can understand."
3. Google DeepMind's AlphaDev (Behind the Scenes Performance):
You don't directly use AlphaDev, but think of it this way: when the JavaScript engine in your browser or the Node.js runtime you use on the backend gets updated, it might include more efficient ways of sorting lists or handling data structures – improvements that could have originated from research like AlphaDev's. So, by simply keeping your browser updated or using the latest stable version of Node.js, your web applications can benefit from these under-the-hood performance enhancements without you needing to write any different code.
4. Open-source Models like AutoGPT (Your Automation Assistant):
Let's say you're starting a new React project and need to look up the best practices for folder structure and component organization in 2025. You could instruct AutoGPT with a goal like: "Research the recommended folder structure and component organization patterns for a medium-sized React application in 2025. Provide a summary of the top 3 approaches and links to relevant articles." AutoGPT would then autonomously browse the web, gather information, and present you with a summary, saving you the time of manually searching and compiling this information yourself.
5. Google's Gemini Models (Especially 2.5 Flash) (For Tough Problems):
Suppose you're experiencing significant performance issues on a complex single-page application built with a modern framework like Vue.js, and you're not sure where the bottleneck is. You could provide Gemini with performance profiling data (like output from your browser's developer tools) and relevant sections of your Vue.js component code, then ask, "Based on this performance data and component logic, what are the most likely causes of the performance issues and what are some potential optimization strategies I could try in Vue.js?" Gemini's advanced reasoning could help you pinpoint areas in your code or architecture that need attention and suggest specific ways to improve performance.
So, are you already using some of these in your dev workflow? I'd love to hear how you're using them or what tasks you think they could help with.
Drop your thoughts or examples in the comments.