Continuous Vulnerability Monitoring with AI: Real-Time Risk Visibility and Adaptive Security Posture Management
The Future of Vulnerability Management is Continuous and AI-Driven Cybersecurity is no longer just about setting up firewalls and running occasional vulnerability scans. In today’s rapidly evolving threat landscape, traditional vulnerability management falls short because it relies on static, point-in-time assessments. Attackers, however, don’t wait for quarterly security audits—they exploit vulnerabilities the moment they surface. This is where AI-driven continuous vulnerability monitoring comes into play. Instead of reacting to threats after the damage is done, AI enables security teams to proactively identify, prioritize, and mitigate vulnerabilities in real time. By integrating AI with cybersecurity workflows, organizations gain adaptive security posture management that evolves dynamically with the threat landscape. Let’s explore how AI is transforming continuous vulnerability monitoring and what security leaders need to consider to stay ahead of adversaries. Why Traditional Vulnerability Management Falls Short For years, cybersecurity teams have relied on periodic vulnerability scans, often conducted monthly, quarterly, or even annually. These scans identify weaknesses but fail to provide ongoing visibility into an organization’s risk posture. Meanwhile, attackers move quickly, exploiting newly discovered vulnerabilities before they even make it onto a security team’s radar. Static vulnerability management has three major shortcomings: Delayed Risk Visibility: By the time a vulnerability is detected, attackers may have already weaponized it. Lack of Context: Traditional scans don’t consider an organization’s specific risk profile, leaving security teams overwhelmed with generic severity scores. Reactive Approach: Organizations often scramble to patch vulnerabilities only after they become a known exploit. Real-World Example of a Missed Exploit: Consider the Log4Shell vulnerability (CVE-2021-44228). Many organizations ran routine scans and identified affected systems. However, attackers had already been exploiting this vulnerability in the wild before many security teams even received an alert. Organizations relying on periodic scanning were left exposed, while those with AI-driven continuous monitoring detected exploit attempts in real time and applied defenses proactively. How AI Enables Real-Time Vulnerability Monitoring AI doesn’t just automate existing processes, it fundamentally changes how vulnerabilities are identified and managed. Here’s how: 1. Automated Data Collection & Analysis AI-powered security platforms continuously scan networks, endpoints, and applications for emerging threats. Unlike traditional tools, AI integrates multiple data sources, including: Threat intelligence feeds (dark web, exploit databases, MITRE ATT&CK framework) Behavioral analytics (monitoring traffic patterns for anomaly detection) Asset context (understanding the criticality of affected systems) Instead of relying solely on CVE databases, AI dynamically updates its threat model based on real-time attack patterns. This ensures that security teams are alerted about high-risk vulnerabilities the moment they emerge. 2. Contextual Risk Analysis AI enhances vulnerability assessment by analyzing risk within the context of an organization’s unique environment. A vulnerability affecting an internet-facing web server is far more dangerous than the same flaw on an isolated internal system. AI prioritizes vulnerabilities by considering factors such as: Exploitability in the wild: AI checks active exploit attempts. Business impact: AI assesses whether a vulnerability affects critical business operations. Network exposure: AI determines whether an affected system is externally accessible. 3. Behavioral Analysis & Anomaly Detection Traditional vulnerability management tools can identify known threats but struggle with novel attack techniques. AI-driven behavioral analysis detects suspicious activity even when no specific CVE exists. For instance: AI might detect repeated failed login attempts on a newly patched system, signaling an attacker testing for vulnerabilities. AI can recognize unusual data exfiltration patterns that indicate an exploited zero-day vulnerability. This proactive approach helps organizations detect and mitigate threats before attackers can take advantage of them. AI-Driven Risk Prioritization: Moving Beyond CVSS Scores Why CVSS Alone Isn’t Enough Most vulnerability management programs rely heavily on the Common Vulnerability Scoring System (CVSS). While CVSS provides a standardized severity rating, it lacks context. Not all “critical” vulnerabilities pose an immediate risk, and some “low” severity vulnerabilities can be devastating in specific environments. For example, CVE-2022-30190 (the Follina vulnerability) had a low initial CVSS score but was widely exploited in ransomware campaigns. AI-driven prioritization ensures that vulnerabilities with high exploitability receiv
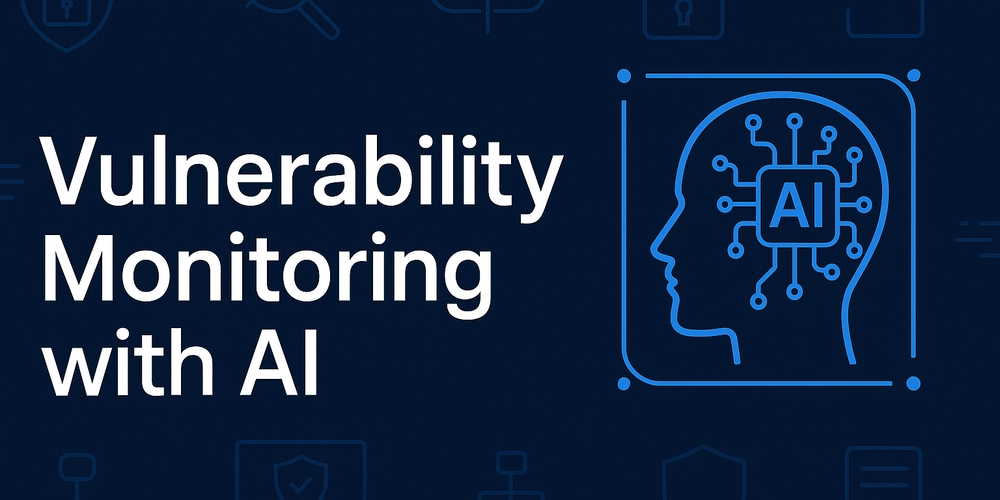
The Future of Vulnerability Management is Continuous and AI-Driven
Cybersecurity is no longer just about setting up firewalls and running occasional vulnerability scans. In today’s rapidly evolving threat landscape, traditional vulnerability management falls short because it relies on static, point-in-time assessments. Attackers, however, don’t wait for quarterly security audits—they exploit vulnerabilities the moment they surface.
This is where AI-driven continuous vulnerability monitoring comes into play. Instead of reacting to threats after the damage is done, AI enables security teams to proactively identify, prioritize, and mitigate vulnerabilities in real time. By integrating AI with cybersecurity workflows, organizations gain adaptive security posture management that evolves dynamically with the threat landscape. Let’s explore how AI is transforming continuous vulnerability monitoring and what security leaders need to consider to stay ahead of adversaries.
Why Traditional Vulnerability Management Falls Short
For years, cybersecurity teams have relied on periodic vulnerability scans, often conducted monthly, quarterly, or even annually. These scans identify weaknesses but fail to provide ongoing visibility into an organization’s risk posture. Meanwhile, attackers move quickly, exploiting newly discovered vulnerabilities before they even make it onto a security team’s radar.
Static vulnerability management has three major shortcomings:
- Delayed Risk Visibility: By the time a vulnerability is detected, attackers may have already weaponized it.
- Lack of Context: Traditional scans don’t consider an organization’s specific risk profile, leaving security teams overwhelmed with generic severity scores.
- Reactive Approach: Organizations often scramble to patch vulnerabilities only after they become a known exploit.
Real-World Example of a Missed Exploit:
Consider the Log4Shell vulnerability (CVE-2021-44228). Many organizations ran routine scans and identified affected systems. However, attackers had already been exploiting this vulnerability in the wild before many security teams even received an alert.
Organizations relying on periodic scanning were left exposed, while those with AI-driven continuous monitoring detected exploit attempts in real time and applied defenses proactively.
How AI Enables Real-Time Vulnerability Monitoring
AI doesn’t just automate existing processes, it fundamentally changes how vulnerabilities are identified and managed. Here’s how:
1. Automated Data Collection & Analysis
AI-powered security platforms continuously scan networks, endpoints, and applications for emerging threats. Unlike traditional tools, AI integrates multiple data sources, including:
- Threat intelligence feeds (dark web, exploit databases, MITRE ATT&CK framework)
- Behavioral analytics (monitoring traffic patterns for anomaly detection)
- Asset context (understanding the criticality of affected systems)
Instead of relying solely on CVE databases, AI dynamically updates its threat model based on real-time attack patterns. This ensures that security teams are alerted about high-risk vulnerabilities the moment they emerge.
2. Contextual Risk Analysis
AI enhances vulnerability assessment by analyzing risk within the context of an organization’s unique environment. A vulnerability affecting an internet-facing web server is far more dangerous than the same flaw on an isolated internal system. AI prioritizes vulnerabilities by considering factors such as:
- Exploitability in the wild: AI checks active exploit attempts.
- Business impact: AI assesses whether a vulnerability affects critical business operations.
- Network exposure: AI determines whether an affected system is externally accessible.
3. Behavioral Analysis & Anomaly Detection
Traditional vulnerability management tools can identify known threats but struggle with novel attack techniques. AI-driven behavioral analysis detects suspicious activity even when no specific CVE exists. For instance:
- AI might detect repeated failed login attempts on a newly patched system, signaling an attacker testing for vulnerabilities.
- AI can recognize unusual data exfiltration patterns that indicate an exploited zero-day vulnerability.
This proactive approach helps organizations detect and mitigate threats before attackers can take advantage of them.
AI-Driven Risk Prioritization: Moving Beyond CVSS Scores
Why CVSS Alone Isn’t Enough
Most vulnerability management programs rely heavily on the Common Vulnerability Scoring System (CVSS). While CVSS provides a standardized severity rating, it lacks context. Not all “critical” vulnerabilities pose an immediate risk, and some “low” severity vulnerabilities can be devastating in specific environments.
For example, CVE-2022-30190 (the Follina vulnerability) had a low initial CVSS score but was widely exploited in ransomware campaigns. AI-driven prioritization ensures that vulnerabilities with high exploitability receive immediate attention, even if their CVSS score suggests otherwise.
1. AI-Based Prioritization Models
AI improves risk prioritization by considering multiple factors, such as:
- Threat Intelligence Integration: AI checks if a vulnerability is actively exploited in the wild.
- Environmental Factors: AI determines whether the affected system is externally exposed or contains sensitive data.
- Exploit Complexity: AI assesses how easy or difficult it is for attackers to exploit the vulnerability.
If companies implement AI-powered vulnerability management platforms like Transilience AI, they would be able to prioritize vulnerabilities and their manual headache is just gone! Their security teams will be free from thousands of vulnerabilities which are often flagged as “high risk.”
However, after deploying AI-based prioritization, they will be able to discover that only a fraction of these vulnerabilities had real-world exploitability. This will allow them to focus on patching critical threats while reducing unnecessary.
2. Adaptive Security Model
AI doesn’t just track vulnerabilities, it adapts security policies dynamically. For example:
- If a high-risk vulnerability is detected on a public-facing server, AI can automatically enforce stricter firewall rules until a patch is applied.
- If an AI model detects increased exploit attempts on a specific vulnerability, it can prioritize patching across affected systems.
3. Compliance Automation
Many industries require strict compliance with regulations such as HIPAA, PCI-DSS, and NIST. AI ensures continuous compliance by:
- Flagging misconfigurations that violate regulatory frameworks.
- Generating compliance reports in real time.
- Automating remediation steps to meet security standards.
Security teams are frequently overloaded with notifications from traditional vulnerability scoring techniques that lack a defined hierarchy. This is altered by AI-driven risk assessment, which dynamically assesses exploitability, business effect, and actual attack patterns to concentrate remedial efforts where they are most needed.
Organizations can transition from a reactive to a proactive defense strategy that adjusts to new threats by going beyond static CVSS scores. Security teams can then effectively cut through the clutter, lower risk exposure, and improve their overall security posture using AI-powered prioritizing.
Implementing AI-Driven Continuous Monitoring: Best Practices
- Choose the right AI-driven vulnerability management tool that integrates with SIEM, XDR, and SOAR platforms.
- Train AI models with relevant data to improve detection accuracy.
- Automate remediation workflows to reduce manual effort and response time.
- Continuously evaluate AI effectiveness by simulating attack scenarios and validating response mechanisms.
AI-Powered Security is the New Standard for Cyber Resilience
AI-driven continuous vulnerability monitoring is no longer a luxury, it’s a necessity. By shifting from static, reactive vulnerability management to real-time, AI-powered security posture management, organizations gain an unprecedented level of threat visibility and proactive risk mitigation.
Looking ahead, AI’s role in cybersecurity will continue to evolve. Future advancements may include predictive threat modeling, AI-driven patch automation, and deeper integration with attack surface management solutions. The organizations that embrace AI-driven security today will be the ones best prepared for tomorrow’s threats.
Cyber threats are evolving, shouldn’t your vulnerability management strategy evolve too?