The basis for successful AI: data quality and structure
Unlocking AI's potential for SMBs with clean data, driving innovation and efficiency across industries.
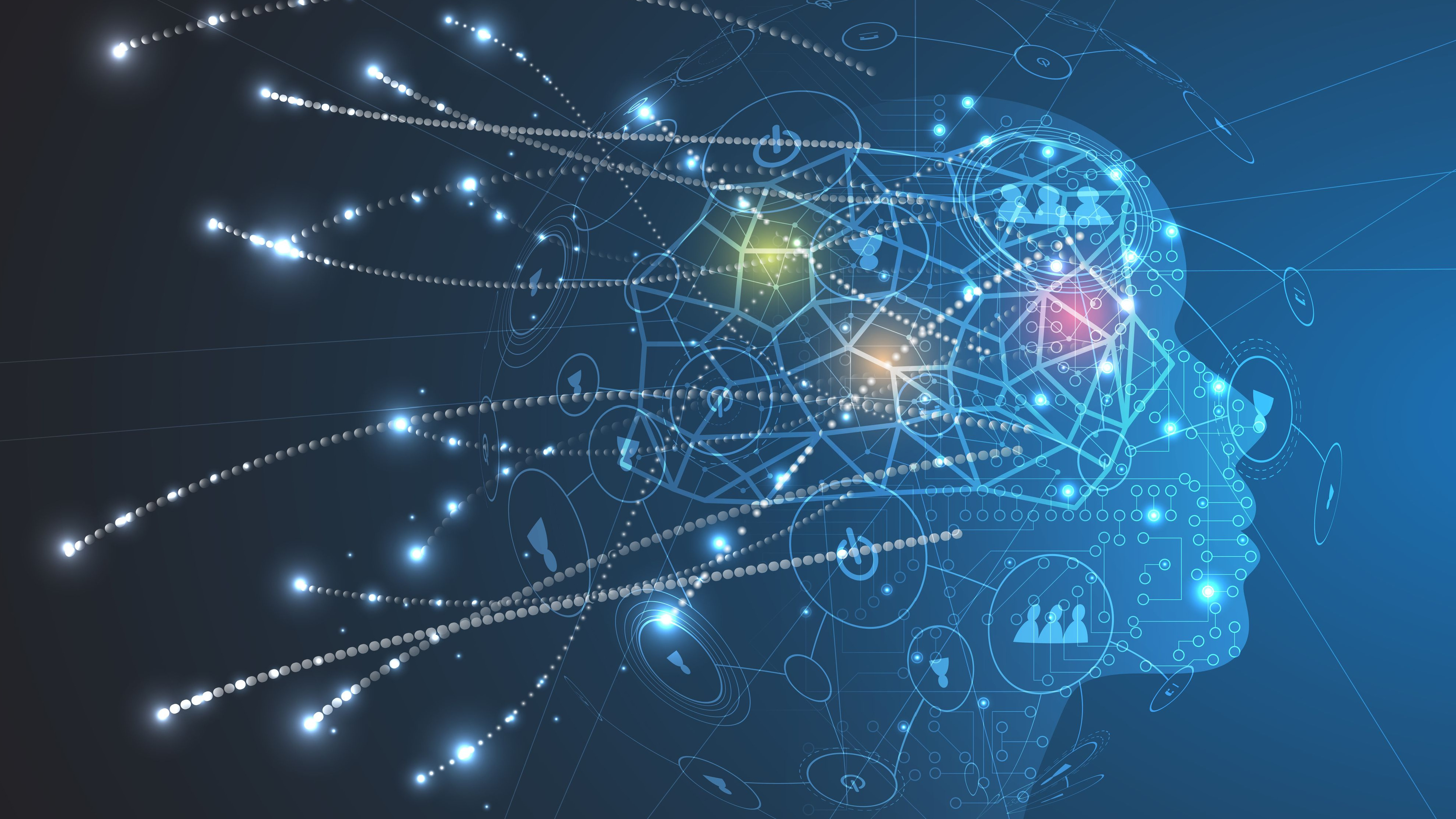
As artificial intelligence becomes more integral to businesses across all industries, small and medium-sized companies are slowly integrating it. In 2024, only 26% of these types of businesses used the technology, despite 76% recognizing its value.
However, as AI's benefits become more pronounced, these businesses will only benefit from integrating it into their operations. As a critical tool, AI can help these businesses build and foster stronger relationships with clients, develop innovative solutions that allow them to compete better with larger institutions and increase efficiency, allowing them to focus on business growth.
Transformative potential AI has for small-and medium-sized businesses
In the coming years, small and medium-sized businesses must incorporate AI to remain competitive in an ever-changing business landscape. The good news is that AI can enable smaller organizations to break through competitively and provide more personalized offerings to clients across industries.
The impact across industries is telling. In the accounting and finance industry, the shift to AI can empower businesses to move from traditional number-crunching services to personalized advisory relationships. Within sales and marketing, AI can go beyond providing predictive insights and can offer real-time personalization to improve sales conversion rates.
AI can provide employees with seamless service and connectivity in the IT industry, where building a digital workplace is the standard. Furthermore, within customer service, AI-powered agents and chatbots help maintain a consistent brand voice across all client engagements while automating inquiries and communications to provide answers at a previously impossible pace.
The bottom line: no matter the industry, AI is improving business outcomes, and small- to medium-sized businesses have much to gain from the technology.
Where to start: clean data is crucial for successful AI integration
It is clear that AI has many benefits, but an AI algorithm is only as good as the data it learns from. Clean, well-structured data is essential for AI models to function accurately and efficiently. AI systems can produce biased, misleading or outright incorrect results without it. Data must be accurate, complete, consistent, unique, valid and timely.
One of the most significant risks of poor data quality is bias. If an AI model is trained on incomplete, inconsistent or skewed data, it will replicate and even amplify those biases. Overrepresenting data from one source while unnecessarily reducing the representation of another can have serious consequences, from discriminatory hiring practices to inaccurate medical diagnoses.
In short, AI models rely on patterns within datasets to make predictions and decisions. The outputs will be unreliable if the data contains errors—such as duplicates, missing values or incorrect labels.
Furthermore, inconsistent and inaccurate data could slow down processing times, increase business costs, and require extensive human intervention to correct mistakes. On the other hand, when data is clean, AI models can train faster and operate more effectively, saving both time and resources. Whether it’s customer interactions, financial transactions or healthcare records, people need to know that AI-driven decisions are based on reliable information.
Poor data quality erodes confidence, while clean data strengthens the credibility of AI systems. Good, clean data is the foundation of successful AI. Without it, even the most sophisticated models will fail to deliver meaningful results. Ensuring high data quality should be a top priority for any organization looking to use AI.
Steps to take to improve data quality
For small and medium-sized businesses to reap the benefits of AI, they must use modern data management tools to guard data quality, including implementing high data quality standards, data structuring and data governance policies.
The first step is to clean and structure the data into a format that AI algorithms can efficiently process and analyze to extract meaningful insights and make accurate predictions. Data is gathered from various sources, including databases, files and application programming interfaces. Once collected, data cleaning is performed to remove inconsistencies, errors and irrelevant information. The data is then converted into a format suitable for AI algorithms, such as numerical values, vectors or graphs.
Data structuring techniques vary based on the type and purpose of the data. For example, relational databases store data in tables with rows and columns, making them ideal for structured data. In contrast, NoSQL databases offer more flexibility by storing data in various formats, making them suitable for unstructured or semi-structured data.
Finally, data storage ensures that the structured data is efficiently organized and accessible for AI processing. Each step is critical for optimizing AI performance and delivering accurate, meaningful insights.
Ensuring data governance
Organizations need a robust data governance framework to maintain high data quality. This internal governance structure—like a cross-functional committee or task force—sets policies, processes and accountability measures.
First, this framework should assign clear roles and responsibilities for managing data, which will help ensure accountability and safeguard critical information. Next, businesses must enforce data controls and standardize formatting and data structures across systems to promote consistency.
Once organizations have established their framework, they should maintain real-time updates and scheduled data refreshes, keeping data relevant. Additionally, compliance with validation rules and predefined formats must be maintained.
Finally, businesses of all sizes must provide user-friendly interfaces, clear documentation and efficient retrieval systems to ensure data is accessible and valuable. Comprehensive data coverage across all relevant systems and processes is necessary.
AI has the potential to transform small and medium-sized businesses significantly. However, the success of these AI initiatives depends heavily on the quality and structure of the data they utilize. By improving data quality through robust standards, effective structuring, comprehensive governance policies and modern management tools, these businesses can fully leverage AI to gain a competitive edge and drive innovation.
We've featured the best small business software.
This article was produced as part of TechRadarPro's Expert Insights channel where we feature the best and brightest minds in the technology industry today. The views expressed here are those of the author and are not necessarily those of TechRadarPro or Future plc. If you are interested in contributing find out more here: https://www.techradar.com/news/submit-your-story-to-techradar-pro