Meta-Learning: Teaching Machines to Learn How to Learn
The domain of artificial intelligence now features a remarkable concept that expands machine learning capabilities: the system of meta-learning, which allows machines to learn new information from prior experiences. The objective of meta-learning differs from task instruction because it trains machines to use their former experiences to adapt their behavior when facing new, unknown assignments. The method duplicates human learning behaviors to deliver substantial progress toward developing adaptable, self-operating AI systems. Students and professionals who want quality education about AI and machine learning have become more active in seeking learning resources because these fields have become vital for innovation. Aggressively pursuing a machine learning course in Canada constitutes a highly effective educational path to build a core and progressive understanding of pioneering fields like meta-learning. What is meta-learning? Meta-learning describes the capacity of machine learning models to develop better learning methods from past training sessions. Standard machine learning models exhibit poor performance after being trained for their designated tasks because they struggle to handle different problem domains. The development of models for quick and low-data learning of new tasks represents the goal of meta-learning approaches. The process of teaching one math problem to a student through conventional machine learning methods resembles the meta-learning approach of providing generic solution methods that apply to diverse problems. Why Meta-Learning Matters Meta-learning serves environments well when there is limited available data or task requirements change often. The need for fast and intelligent adaptations arises usually in robotics and personalized healthcare in addition to real-time fraud detection. A robot designed to walk on flat surfaces is an example. The standard model demonstrates difficulty when facing a sudden switch to uneven terrain. Through meta-learning, a model can apply past knowledge of different walking gaits to immediately handle new terrains. The ability of systems to change in response to new circumstances is a key reason why research into meta-learning has increased. Students who want to pursue AI engineering can study advanced machine learning in courses in Canada while training with modern tools and real-world datasets that mimic these diverse challenges. How Does Meta-Learning Work? The main components of meta-learning models consist of three essential elements. The successful execution of a task needs a base learner, which operates as the main algorithm. The meta-learning system enhances the base learner's performance when applied to different tasks. The task distribution consists of every set of tasks, which helps the model develop its learning abilities. Multiple approaches operate within the field of meta-learning. Neural structures created through model-based methods allow for fast adjustment based on input feedback, using Long Short-Term Memory (LSTM) networks for parameter modification. Learning similarity functions through metric-based methods enables new tasks to be solved by comparing them with previously learned tasks. Prototypical networks and Siamese networks represent two such methods. Model-Agnostic Meta-Learning (MAML), along with other optimization-based methods, works to improve the learning algorithm's performance for faster acquisition of new tasks. Students learning AI and ML courses in Canada work through practical assignments that incorporate these frameworks, as well as research and laboratory experiences, to better understand these complex systems. Real-World Applications of Meta-Learning The practical application of meta-learning is found in various industrial sectors. Healthcare organizations use meta-learning algorithms to create individualized treatment plans that quickly adopt treatment options based on limited patient data. The natural language processing technology of NLP applies model generalization from limited examples to achieve translation and sentiment analysis in languages with reduced programming support. The finance industry uses meta-learning systems to detect fraud by creating adaptable software that defeats newly developed deceptive methods. The increasing significance of meta-learning research has transformed the field into an exciting pursuit for machine learning courses in Canada, particularly those focused on practical applications. Challenges and Future Directions Multiple barriers exist on the path to meta-learning, despite its potential value. Running training processes for meta-learning models requires significant computational resources, so these models can face limited acceptance. The present challenge exists in maintaining generalization alongside preventing overfitting because models need to extract knowledge from past assignments, yet retain enough flexibility
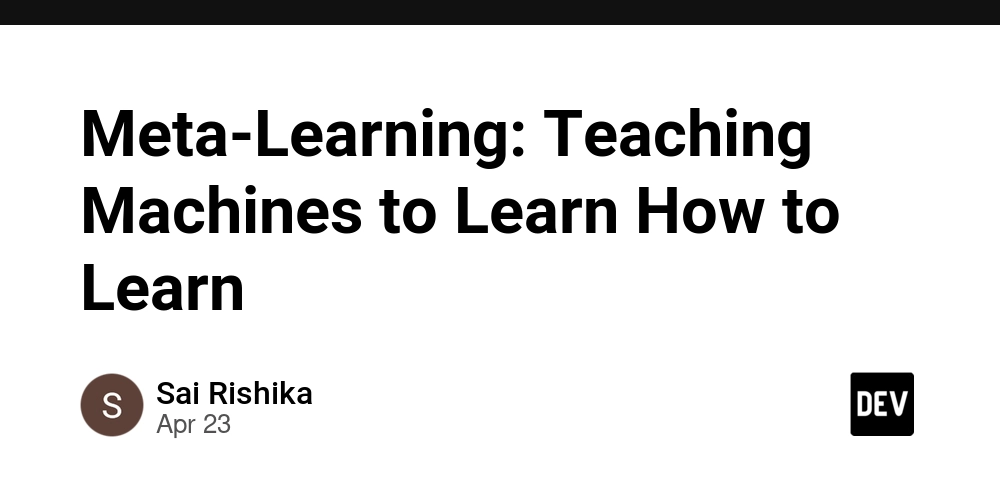
The domain of artificial intelligence now features a remarkable concept that expands machine learning capabilities: the system of meta-learning, which allows machines to learn new information from prior experiences. The objective of meta-learning differs from task instruction because it trains machines to use their former experiences to adapt their behavior when facing new, unknown assignments. The method duplicates human learning behaviors to deliver substantial progress toward developing adaptable, self-operating AI systems.
Students and professionals who want quality education about AI and machine learning have become more active in seeking learning resources because these fields have become vital for innovation. Aggressively pursuing a machine learning course in Canada constitutes a highly effective educational path to build a core and progressive understanding of pioneering fields like meta-learning.
What is meta-learning?
Meta-learning describes the capacity of machine learning models to develop better learning methods from past training sessions. Standard machine learning models exhibit poor performance after being trained for their designated tasks because they struggle to handle different problem domains. The development of models for quick and low-data learning of new tasks represents the goal of meta-learning approaches.
The process of teaching one math problem to a student through conventional machine learning methods resembles the meta-learning approach of providing generic solution methods that apply to diverse problems.
Why Meta-Learning Matters
Meta-learning serves environments well when there is limited available data or task requirements change often. The need for fast and intelligent adaptations arises usually in robotics and personalized healthcare in addition to real-time fraud detection.
A robot designed to walk on flat surfaces is an example. The standard model demonstrates difficulty when facing a sudden switch to uneven terrain. Through meta-learning, a model can apply past knowledge of different walking gaits to immediately handle new terrains. The ability of systems to change in response to new circumstances is a key reason why research into meta-learning has increased.
Students who want to pursue AI engineering can study advanced machine learning in courses in Canada while training with modern tools and real-world datasets that mimic these diverse challenges.
How Does Meta-Learning Work?
The main components of meta-learning models consist of three essential elements. The successful execution of a task needs a base learner, which operates as the main algorithm. The meta-learning system enhances the base learner's performance when applied to different tasks. The task distribution consists of every set of tasks, which helps the model develop its learning abilities.
Multiple approaches operate within the field of meta-learning. Neural structures created through model-based methods allow for fast adjustment based on input feedback, using Long Short-Term Memory (LSTM) networks for parameter modification. Learning similarity functions through metric-based methods enables new tasks to be solved by comparing them with previously learned tasks. Prototypical networks and Siamese networks represent two such methods. Model-Agnostic Meta-Learning (MAML), along with other optimization-based methods, works to improve the learning algorithm's performance for faster acquisition of new tasks.
Students learning AI and ML courses in Canada work through practical assignments that incorporate these frameworks, as well as research and laboratory experiences, to better understand these complex systems.
Real-World Applications of Meta-Learning
The practical application of meta-learning is found in various industrial sectors. Healthcare organizations use meta-learning algorithms to create individualized treatment plans that quickly adopt treatment options based on limited patient data. The natural language processing technology of NLP applies model generalization from limited examples to achieve translation and sentiment analysis in languages with reduced programming support. The finance industry uses meta-learning systems to detect fraud by creating adaptable software that defeats newly developed deceptive methods.
The increasing significance of meta-learning research has transformed the field into an exciting pursuit for machine learning courses in Canada, particularly those focused on practical applications.
Challenges and Future Directions
Multiple barriers exist on the path to meta-learning, despite its potential value. Running training processes for meta-learning models requires significant computational resources, so these models can face limited acceptance. The present challenge exists in maintaining generalization alongside preventing overfitting because models need to extract knowledge from past assignments, yet retain enough flexibility to handle fresh tasks. Research investigators continue to work on developing successful evaluation standards for various functions that will serve as standards across numerous tasks.
The development to resolve these problems is progressing steadily.
Scientists develop integrated learning models that unite reinforcement learning with unsupervised learning to improve the versatility and operational speed of meta-learning systems.
Students taking a machine learning course in Canada gain special abilities to make scientific advancements in this field. The innovative AI sector of Canada supports educational and research activities through its leading institutions, including the Vector Institute and joint projects with major technology companies.
Getting Started with Meta-Learning
An interest in machines obtaining learning capabilities should drive students toward formal meta-learning education. Across Canada, universities maintain distinguished positions that teach AI programs, which include meta-learning within their curricula. Under the umbrella of AI and ML courses in Canada, students can find programs that establish basic skills in both theory and practice for artificial intelligence.
A standard machine learning course in Canadian institutions focuses on supervised and unsupervised learning alongside neural networks and reinforcement learning while also increasingly incorporating meta-learning concepts. Students achieve maximum success through instruction combined with academic research together with industrial connections that prepare them to handle actual challenges.
Conclusion
The development of meta-learning marks a significant progression in machine learning, taking systems from performing specific tasks to achieving widespread flexibility. The growth rate of AI will enhance the need for professionals who grasp these complex methods.
The present stands as the best opportunity for anyone desiring to study data science, AI research, or technology expertise. A machine learning course in Canada, combined with the diverse AI and ML courses available in Canada, will help students master this influential technology.