How Data Science and Analytics are transforming industries today
Data science and analytics are more than simply IT departments' technical tools in today's hyper connected, digital-first world; they are essential to contemporary decision-making, innovation, and competitive advantage. Organizations in almost every industry now depend on data to inform strategy, streamline operations, customize consumer experiences, and predict future trends as a result of the rise of big data, artificial intelligence (AI), and machine learning (ML). Data science has the unquestionable ability to revolutionize a variety of industries, including marketing, manufacturing, healthcare, and finance. Understanding Data Science and Analytics It's crucial to understand the differences between data science and analytics before exploring their transformative potential. Data science is a multidisciplinary field that uses scientific methods, processes, algorithms, and systems to extract knowledge and insights from both structured and unstructured data, while data analytics is a subset of data science that specifically refers to the process of analyzing datasets to make inferences and guide decision-making. Together, they make a powerful combination: data analytics shows "what" is happening, while data science frequently delves deeper into "why" it's happening and "what might happen next." Health Care: Precision and Predictive Medicine Healthcare has seen a profound transformation thanks to data science. With the introduction of wearable technology, genomics, and electronic health records (EHRs), an unprecedented amount of data is now being collected and analyzed. Predictive analytics in healthcare helps identify high-risk patients, potential epidemics, and the progression of diseases. For instance, by analyzing historical patient data, machine learning models can predict hospital readmissions or the onset of chronic diseases like diabetes. Precision medicine, which customizes treatment plans based on individual variability in genes and lifestyle, is also becoming a reality thanks to advanced data analysis techniques. The Human Genome Project, and the subsequent rise of bioinformatics, owes much of its success to the computational power of data science. AI models can mimic how drugs interact with the human body, predicting side effects and success rates even before clinical trials start. Pharmaceutical companies also use data science for drug discovery and development, which drastically cuts down on the time and expense needed to bring new drugs to market. Finance: Risk Management and Algorithmic Trading Although data has always been at the heart of the financial industry, its size and scope have significantly increased. These days, data science plays a key role in algorithmic trading, fraud detection, credit scoring, and client segmentation. Banks can prevent fraud before it happens by using machine learning algorithms to detect suspect transaction patterns in real time. To evaluate credit risk more precisely than with conventional scoring techniques, credit card firms employ large databases and prediction models. To create complex models that can evaluate market trends, forecast stock prices, and execute transactions in milliseconds, quantitative analysts, or quants, use data science in the trading industry. High-frequency trading (HFT) platforms, powered by AI, make transactions based on real-time data feeds, ensuring both speed and efficiency. Additionally, budgeting programs like YNAB and personal finance apps like Mint leverage data analytics to provide users with personalized recommendations, spending insights, and saving techniques. Retail: Personalization and Inventory Optimization The clever use of customer data has permanently altered the retail environment. Retailers are more able to comprehend and predict customer behavior than ever before, whether through e-commerce platforms or physical storefronts with integrated digital systems. One of the first companies to use data science to power recommendation engines is Amazon. Amazon provides highly tailored recommendations by examining previous purchases, search histories, and browsing habits, which raises conversion rates and improves consumer happiness. Analytics are also used by retailers to improve inventory control. Businesses can lessen overstock and stockouts by using predictive models that forecast demand based on geographical trends, promotions, and seasonality. By guaranteeing product availability, this raises consumer satisfaction in addition to profits. In order to stay responsive to public opinion, brands can make real-time adjustments to their marketing strategy and product offerings by using sentiment analysis, which involves examining social media posts and customer evaluations. Manufacturing: Predictive Maintenance and Smart Factories Smart manufacturing and the Industrial Internet of Things (IIoT), which both mainly rely on data science and analytics, are
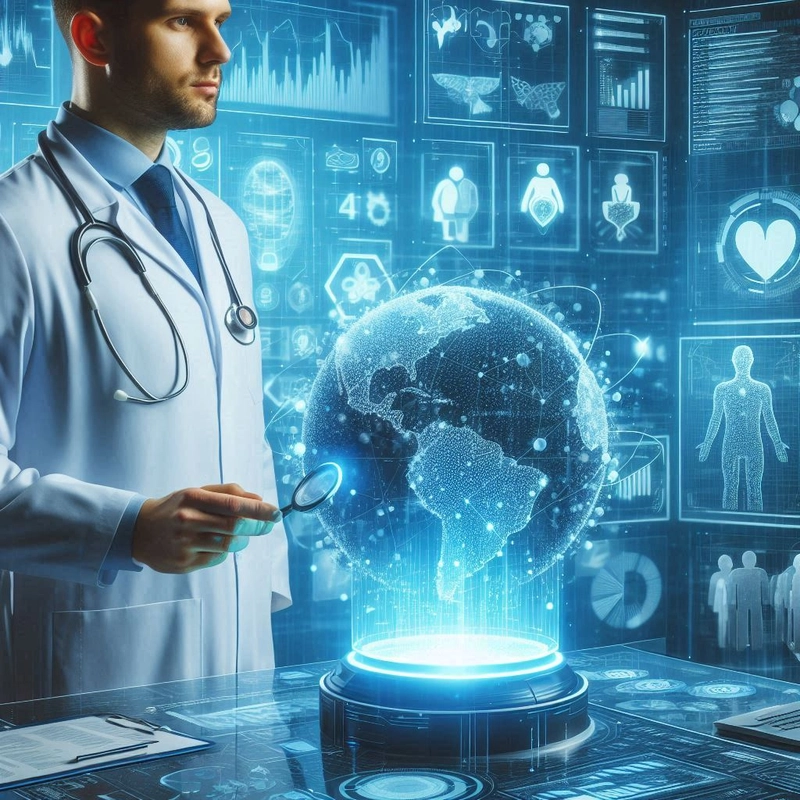
Data science and analytics are more than simply IT departments' technical tools in today's hyper connected, digital-first world; they are essential to contemporary decision-making, innovation, and competitive advantage. Organizations in almost every industry now depend on data to inform strategy, streamline operations, customize consumer experiences, and predict future trends as a result of the rise of big data, artificial intelligence (AI), and machine learning (ML). Data science has the unquestionable ability to revolutionize a variety of industries, including marketing, manufacturing, healthcare, and finance.
Understanding Data Science and Analytics
It's crucial to understand the differences between data science and analytics before exploring their transformative potential. Data science is a multidisciplinary field that uses scientific methods, processes, algorithms, and systems to extract knowledge and insights from both structured and unstructured data, while data analytics is a subset of data science that specifically refers to the process of analyzing datasets to make inferences and guide decision-making. Together, they make a powerful combination: data analytics shows "what" is happening, while data science frequently delves deeper into "why" it's happening and "what might happen next."
Health Care: Precision and Predictive Medicine
Healthcare has seen a profound transformation thanks to data science. With the introduction of wearable technology, genomics, and electronic health records (EHRs), an unprecedented amount of data is now being collected and analyzed.
Predictive analytics in healthcare helps identify high-risk patients, potential epidemics, and the progression of diseases. For instance, by analyzing historical patient data, machine learning models can predict hospital readmissions or the onset of chronic diseases like diabetes.
Precision medicine, which customizes treatment plans based on individual variability in genes and lifestyle, is also becoming a reality thanks to advanced data analysis techniques. The Human Genome Project, and the subsequent rise of bioinformatics, owes much of its success to the computational power of data science.
AI models can mimic how drugs interact with the human body, predicting side effects and success rates even before clinical trials start. Pharmaceutical companies also use data science for drug discovery and development, which drastically cuts down on the time and expense needed to bring new drugs to market.
Finance: Risk Management and Algorithmic Trading
Although data has always been at the heart of the financial industry, its size and scope have significantly increased. These days, data science plays a key role in algorithmic trading, fraud detection, credit scoring, and client segmentation.
Banks can prevent fraud before it happens by using machine learning algorithms to detect suspect transaction patterns in real time. To evaluate credit risk more precisely than with conventional scoring techniques, credit card firms employ large databases and prediction models.
To create complex models that can evaluate market trends, forecast stock prices, and execute transactions in milliseconds, quantitative analysts, or quants, use data science in the trading industry. High-frequency trading (HFT) platforms, powered by AI, make transactions based on real-time data feeds, ensuring both speed and efficiency.
Additionally, budgeting programs like YNAB and personal finance apps like Mint leverage data analytics to provide users with personalized recommendations, spending insights, and saving techniques.
Retail: Personalization and Inventory Optimization
The clever use of customer data has permanently altered the retail environment. Retailers are more able to comprehend and predict customer behavior than ever before, whether through e-commerce platforms or physical storefronts with integrated digital systems.
One of the first companies to use data science to power recommendation engines is Amazon. Amazon provides highly tailored recommendations by examining previous purchases, search histories, and browsing habits, which raises conversion rates and improves consumer happiness.
Analytics are also used by retailers to improve inventory control. Businesses can lessen overstock and stockouts by using predictive models that forecast demand based on geographical trends, promotions, and seasonality. By guaranteeing product availability, this raises consumer satisfaction in addition to profits.
In order to stay responsive to public opinion, brands can make real-time adjustments to their marketing strategy and product offerings by using sentiment analysis, which involves examining social media posts and customer evaluations.
Manufacturing: Predictive Maintenance and Smart Factories
Smart manufacturing and the Industrial Internet of Things (IIoT), which both mainly rely on data science and analytics, are the driving forces behind Industry 4.0, the fourth industrial revolution.
Predictive analytics can be used to predict equipment failures based on the constant data generated by sensors integrated in manufacturing equipment. Predictive maintenance extends the life of machinery, improves operating efficiency, and decreases unscheduled downtime.
Data science is also essential to quality control, as computer vision and machine learning algorithms check products for flaws faster and more accurately than a human can.
In addition, entire smart factories are being constructed in which all of the components, from supply chains to assembly lines, are digitally connected and optimized in real time, and production is streamlined and scenarios tested using digital twins and simulations before any physical changes are made.
Marketing: Targeting, Optimization and Campaign Optimization
Without data, marketing in the digital age is like driving blind. With the help of data science, marketers can gain a detailed understanding of consumer behavior, enabling hyper-targeted campaigns that send the appropriate message to the right person at the right moment.
Marketers employ clustering algorithms to categorize consumers based on demographics, internet behavior, purchase history, and psychographics. These insights lead to more relevant adverts and content, enhancing engagement rates.
Marketers can optimize anything from landing page layouts to email subject lines by comparing performance metrics across variations using A/B testing, a basic analytical technique.
Additionally, social media analytics monitor brand sentiment and engagement on various platforms, providing businesses with a real-time understanding of public opinion. Data on audience demographics, reach, and engagement frequently serve as the basis for influencer marketing tactics.
Transportation and Logistics: Route Optimization and Autonomous Vehicles
Data has always been important to logistics companies like UPS and FedEx, but recent advances in analytics have boosted their operations. For example, ride-sharing services like Uber and Lyft use real-time analytics for dynamic pricing, demand forecasting, and driver allocation; these platforms predict where demand will surge and deploy drivers accordingly, minimizing wait times and maximizing profits. In the world of autonomous vehicles, data science is essential: self-driving cars process massive amounts of sensor data in real time, from cameras and LiDAR to radar and GPS, to make safe and effective driving decisions.
Challenges and Ethical Considerations
Data science and analytics present significant ethical and technical issues despite their advantages. Concern over data privacy is growing, especially as more businesses gather and keep private data. These issues have been addressed by regulatory frameworks such as the CCPA and GDPR, but compliance and enforcement are still difficult.
Algorithm and data bias is another significant problem. In industries like hiring, lending, and law enforcement, in particular, predictive models have the potential to reinforce existing disparities if a dataset is biased or lacking.
Additionally, there is a bottleneck for companies looking to implement these technologies since the demand for qualified data scientists frequently outpaces the supply. For insights to be not only generated but also applied successfully, companies need to invest in data literacy for all employees.
The Future: Data Driven Everything
In the future, augmented intelligence—where people and robots collaborate to make smarter decisions—is probably going to be a reality as computing power keeps increasing and data becomes progressively more abundant. Data science will be pushed to its limits by technologies like edge computing, quantum computing, and real-time analytics.
In order to address some of the most important issues facing the globe today, from food security to climate change, emerging sectors like agritech, edtech, and climate tech are also starting to use data-driven approaches.