AI, Ethics, and Data Security: Navigating the Risks in Banking
The rise of AI in financial services has ushered in a new era of innovation, efficiency, and personalized banking. From automating risk assessments to enhancing customer interactions, AI is rapidly becoming the backbone of modern financial institutions. However, with this advancement comes a pressing need to address the ethical and security implications of AI systems. As financial institutions rely more heavily on artificial intelligence, it becomes critical to ensure ethical AI implementation and robust data protection strategies. The future of banking depends not only on the power of AI but also on the trust it can uphold. The Promise and Peril of AI in Financial Services The application of AI in financial services has transformed everything from loan underwriting to fraud detection. By analyzing vast datasets in real time, AI systems can identify hidden patterns, improve customer experiences, and make faster, more accurate decisions. Yet, these benefits also come with significant risks: Biased algorithms can reinforce social and economic inequalities. Opaque AI models may make decisions that are difficult to explain or audit. Sensitive financial data becomes a prime target for cybercriminals. Regulatory non-compliance can result in costly legal consequences. These risks make it essential for banks to strike a balance between innovation and responsibility. Ethical AI Implementation: Building Trust into Technology Ethical AI implementation in banking isn’t a luxury—it’s a necessity. Financial institutions are stewards of customer trust and must ensure that AI systems uphold fairness, transparency, and accountability. 1. Bias Detection and Mitigation AI algorithms learn from data. If the historical data used for training includes biases—intentional or not—the algorithm will likely reproduce them. This can result in discriminatory lending practices or unfair credit scoring. To counteract this, institutions must: Regularly audit AI models for bias Use diverse and representative datasets Incorporate fairness constraints in model design A commitment to inclusivity and equity should guide every AI initiative. 2. Transparency and Explainability Customers and regulators alike demand to know how and why AI-driven decisions are made. This is especially true in high-stakes scenarios like loan approvals, fraud alerts, or investment recommendations. Techniques like Explainable AI (XAI) are being developed to make machine learning decisions more understandable. Banks must ensure that AI systems can provide clear, human-readable justifications for their actions. 3. Human Oversight While automation is a core benefit of AI in financial services, it should never fully replace human judgment, especially in ethically sensitive or complex scenarios. Implementing "human-in-the-loop" systems ensures that AI decisions can be reviewed and reversed by qualified professionals when needed. This adds a layer of accountability and ensures compliance with ethical standards. Data Security in an AI-Driven Environment The digital transformation of banking has made cybersecurity more critical than ever. As AI systems process and store sensitive data, they also introduce new vulnerabilities. 1. Data Privacy and Consent Financial institutions must ensure that AI models only use data that has been lawfully collected and with full customer consent. With regulations like GDPR and CCPA in place, any mishandling of personal data can result in hefty penalties. Banks must clearly communicate how customer data is collected, used, and protected. Consent should be informed, voluntary, and revocable. 2. AI-Powered Cybersecurity On the flip side, AI can also strengthen data security. AI-powered cybersecurity tools can detect anomalies, identify threats in real time, and proactively defend against cyberattacks. By leveraging AI to secure AI, banks can build a more resilient digital ecosystem. 3. Robust Data Governance Frameworks Strong data governance is foundational to both ethical AI and cybersecurity. This involves: Data classification and encryption Access controls and identity management Regular audits and compliance checks By maintaining clear policies on data lifecycle management, financial institutions can reduce exposure and ensure compliance. Regulatory Landscape and Compliance Regulatory bodies around the world are catching up with the rapid adoption of AI. From the EU’s AI Act to proposed U.S. regulations on algorithmic accountability, the focus is on making AI more transparent and accountable. Banks must stay ahead of evolving regulations by: Collaborating with regulators Participating in ethical AI forums Implementing compliance-ready AI solutions Early adoption of compliance frameworks not only reduces legal risk but also demonstrates a proactive stance on responsible innovation. A Framework for Responsible AI in Financial Services To truly navigate the ethical and security challenges of AI, banks need
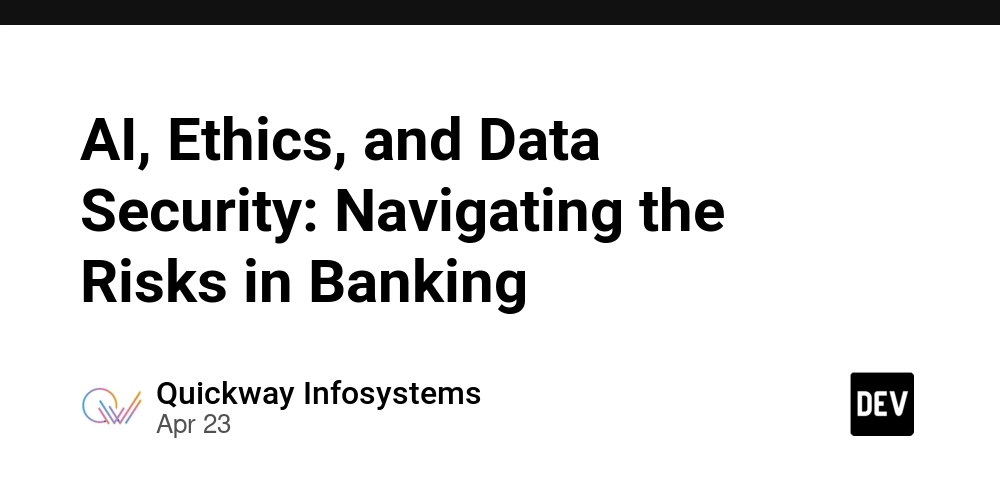
The rise of AI in financial services has ushered in a new era of innovation, efficiency, and personalized banking. From automating risk assessments to enhancing customer interactions, AI is rapidly becoming the backbone of modern financial institutions. However, with this advancement comes a pressing need to address the ethical and security implications of AI systems.
As financial institutions rely more heavily on artificial intelligence, it becomes critical to ensure ethical AI implementation and robust data protection strategies. The future of banking depends not only on the power of AI but also on the trust it can uphold.
The Promise and Peril of AI in Financial Services
The application of AI in financial services has transformed everything from loan underwriting to fraud detection. By analyzing vast datasets in real time, AI systems can identify hidden patterns, improve customer experiences, and make faster, more accurate decisions.
Yet, these benefits also come with significant risks:
Biased algorithms can reinforce social and economic inequalities.
Opaque AI models may make decisions that are difficult to explain or audit.
Sensitive financial data becomes a prime target for cybercriminals.
Regulatory non-compliance can result in costly legal consequences.
These risks make it essential for banks to strike a balance between innovation and responsibility.
Ethical AI Implementation: Building Trust into Technology
Ethical AI implementation in banking isn’t a luxury—it’s a necessity. Financial institutions are stewards of customer trust and must ensure that AI systems uphold fairness, transparency, and accountability.
1. Bias Detection and Mitigation
AI algorithms learn from data. If the historical data used for training includes biases—intentional or not—the algorithm will likely reproduce them. This can result in discriminatory lending practices or unfair credit scoring.
To counteract this, institutions must:
- Regularly audit AI models for bias
- Use diverse and representative datasets
- Incorporate fairness constraints in model design A commitment to inclusivity and equity should guide every AI initiative.
2. Transparency and Explainability
Customers and regulators alike demand to know how and why AI-driven decisions are made. This is especially true in high-stakes scenarios like loan approvals, fraud alerts, or investment recommendations.
Techniques like Explainable AI (XAI) are being developed to make machine learning decisions more understandable. Banks must ensure that AI systems can provide clear, human-readable justifications for their actions.
3. Human Oversight
While automation is a core benefit of AI in financial services, it should never fully replace human judgment, especially in ethically sensitive or complex scenarios.
Implementing "human-in-the-loop" systems ensures that AI decisions can be reviewed and reversed by qualified professionals when needed. This adds a layer of accountability and ensures compliance with ethical standards.
Data Security in an AI-Driven Environment
The digital transformation of banking has made cybersecurity more critical than ever. As AI systems process and store sensitive data, they also introduce new vulnerabilities.
1. Data Privacy and Consent
Financial institutions must ensure that AI models only use data that has been lawfully collected and with full customer consent. With regulations like GDPR and CCPA in place, any mishandling of personal data can result in hefty penalties.
Banks must clearly communicate how customer data is collected, used, and protected. Consent should be informed, voluntary, and revocable.
2. AI-Powered Cybersecurity
On the flip side, AI can also strengthen data security. AI-powered cybersecurity tools can detect anomalies, identify threats in real time, and proactively defend against cyberattacks.
By leveraging AI to secure AI, banks can build a more resilient digital ecosystem.
3. Robust Data Governance Frameworks
Strong data governance is foundational to both ethical AI and cybersecurity. This involves:
- Data classification and encryption
- Access controls and identity management
- Regular audits and compliance checks
By maintaining clear policies on data lifecycle management, financial institutions can reduce exposure and ensure compliance.
Regulatory Landscape and Compliance
Regulatory bodies around the world are catching up with the rapid adoption of AI. From the EU’s AI Act to proposed U.S. regulations on algorithmic accountability, the focus is on making AI more transparent and accountable.
Banks must stay ahead of evolving regulations by:
- Collaborating with regulators
- Participating in ethical AI forums
- Implementing compliance-ready AI solutions
Early adoption of compliance frameworks not only reduces legal risk but also demonstrates a proactive stance on responsible innovation.
A Framework for Responsible AI in Financial Services
To truly navigate the ethical and security challenges of AI, banks need a comprehensive framework that includes:
Ethical principles: Fairness, accountability, transparency, and inclusivity
Technical controls: Bias audits, explainability, and secure design
Organizational policies: AI ethics boards, internal training, and compliance teams
Customer engagement: Transparency in communications and accessible channels for dispute resolution
A holistic approach ensures that AI in financial services enhances—not erodes—customer trust.
Conclusion
The integration of AI in banking is no longer a future trend—it’s a current reality. But as institutions embrace this transformation, the importance of ethical AI implementation and rigorous data security grows exponentially.
Balancing innovation with responsibility will define the next generation of financial services. Those who get it right won’t just lead the market—they’ll set the gold standard for trust in the age of intelligent finance.