A Beginner’s Guide to Time Series Forecasting Using Linear Regression
What Is Time Series Forecasting? Have you ever considered what your sales might be next month? Or how forecasted weather might change next week? Maybe you’re even curious whether your website traffic will keep growing at the same pace? Time series forecasting can help answer these questions by revealing trends in patterns of data over time, and it's simpler than you realise to get started! In this introductory guide to time series forecasting we will show you how to use linear regression to make future predictions, a great starting point for anyone wanting insight into forecasting methods. Whether you are a college student, a small business owner, or a data nerd like us, you will be able to see trends and make predictions with the help of a few lines of code in Python. We will start with the basic components in this guide to time series analysis, and then you are ready to forecast using linear regression, before moving on to more advanced forecasting techniques such as ARIMA or SARIMAX. So, with that said, let’s get started and see what the future holds! Why Use Linear Regression for Time Series? Linear regression is a classic technique that finds a straight line to describe the relationship between variables. In time series, we often use it to model trends over time—like how sales increase month by month. Here’s why it’s perfect for beginners: Simple to Understand: It assumes a straight-line trend (e.g., sales go up steadily), making it easy to grasp and apply. Works with Trends: It’s great for data with a clear upward or downward pattern, like growing website traffic over months. Fast to Implement: With Python, you can build a model in just a few lines of code. Foundation for More: Linear regression teaches you core concepts before moving to advanced models like ARIMA or LSTM. While it’s not perfect for complex patterns (like seasonal cycles or sudden spikes), it’s an excellent way to dip your toes into forecasting and build confidence with time series data. How Does Time Series Forecasting with Linear Regression Work? Using linear regression for forecasting is like drawing a straight line through your data points to predict where they’ll go next. Here’s the step-by-step process: Collect Time Series Data: Gather data over time, like monthly sales or daily temperatures, ensuring it’s in chronological order. Prepare the Data: Turn time into a number (e.g., month 1, month 2) and check for trends. Linear regression works best with data that shows a steady trend. Fit the Model: Use linear regression to find the best straight line that matches your data, like “sales increase by $500 per month.” Make Predictions: Extend the line into the future to forecast new values, like next month’s sales. Evaluate and Adjust: Compare your predictions to actual data (if available) to see how well the model performs, and tweak as needed. This approach is straightforward but powerful for spotting trends, making it a great starting point for beginners. Building It: A Simple Code Example Let’s build a time series forecasting model using linear regression in Python. We’ll predict monthly sales for a small store, using pandas for data handling, scikit-learn for linear regression, and matplotlib for visualization—tools you’ve shown interest in before in your time series projects. This example is beginner-friendly and shows the full process. # Import libraries import pandas as pd import numpy as np from sklearn.linear_model import LinearRegression import matplotlib.pyplot as plt # Sample dataset: monthly sales (in thousands) data = pd.DataFrame({ 'Month': range(1, 13), # Months 1 to 12 'Sales': [120, 130, 125, 140, 145, 150, 160, 155, 170, 180, 175, 190] }) # Step 1: Prepare data for linear regression X = data['Month'].values.reshape(-1, 1) # Independent variable (time) y = data['Sales'].values # Dependent variable (sales) # Step 2: Fit the linear regression model model = LinearRegression() model.fit(X, y) # Step 3: Forecast the next 3 months (months 13, 14, 15) future_months = np.array([13, 14, 15]).reshape(-1, 1) forecast = model.predict(future_months) # Step 4: Visualize the data and forecast plt.scatter(data['Month'], data['Sales'], color='blue', label='Actual Sales') plt.plot(data['Month'], model.predict(X), color='red', label='Trend Line') plt.scatter(future_months, forecast, color='green', label='Forecast') plt.xlabel('Month') plt.ylabel('Sales (in thousands)') plt.title('Sales Forecasting with Linear Regression') plt.legend() plt.grid(True) plt.savefig('sales_forecast.png') # Step 5: Print the forecast print("3-Month Sales Forecast:") for month, sales in zip([13, 14, 15], forecast): print(f"Month {month}: {sales:.1f} thousand") Output 3-Month Sales Forecast: Month 13: 193.2 thousand Month 14: 197.8 thousand Month 15: 202.4 thousand What’s Happening? Data Setup: We create a small dataset of 12 months of sale
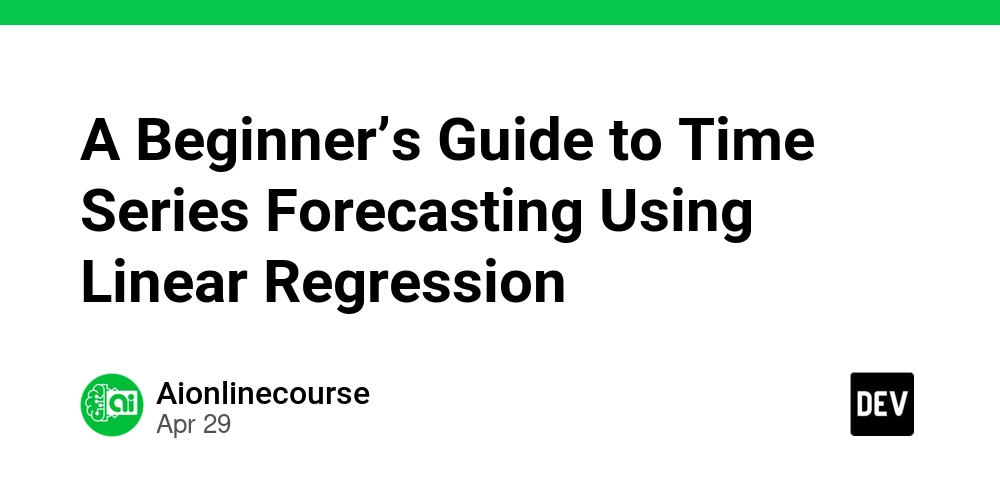
What Is Time Series Forecasting?
Have you ever considered what your sales might be next month? Or how forecasted weather might change next week? Maybe you’re even curious whether your website traffic will keep growing at the same pace? Time series forecasting can help answer these questions by revealing trends in patterns of data over time, and it's simpler than you realise to get started! In this introductory guide to time series forecasting we will show you how to use linear regression to make future predictions, a great starting point for anyone wanting insight into forecasting methods. Whether you are a college student, a small business owner, or a data nerd like us, you will be able to see trends and make predictions with the help of a few lines of code in Python. We will start with the basic components in this guide to time series analysis, and then you are ready to forecast using linear regression, before moving on to more advanced forecasting techniques such as ARIMA or SARIMAX. So, with that said, let’s get started and see what the future holds!
Why Use Linear Regression for Time Series?
Linear regression is a classic technique that finds a straight line to describe the relationship between variables. In time series, we often use it to model trends over time—like how sales increase month by month.
Here’s why it’s perfect for beginners:
- Simple to Understand: It assumes a straight-line trend (e.g., sales go up steadily), making it easy to grasp and apply.
- Works with Trends: It’s great for data with a clear upward or downward pattern, like growing website traffic over months.
- Fast to Implement: With Python, you can build a model in just a few lines of code.
- Foundation for More: Linear regression teaches you core concepts before moving to advanced models like ARIMA or LSTM.
While it’s not perfect for complex patterns (like seasonal cycles or sudden spikes), it’s an excellent way to dip your toes into forecasting and build confidence with time series data.
How Does Time Series Forecasting with Linear Regression Work?
Using linear regression for forecasting is like drawing a straight line through your data points to predict where they’ll go next. Here’s the step-by-step process:
- Collect Time Series Data: Gather data over time, like monthly sales or daily temperatures, ensuring it’s in chronological order.
- Prepare the Data: Turn time into a number (e.g., month 1, month 2) and check for trends. Linear regression works best with data that shows a steady trend.
- Fit the Model: Use linear regression to find the best straight line that matches your data, like “sales increase by $500 per month.”
- Make Predictions: Extend the line into the future to forecast new values, like next month’s sales.
- Evaluate and Adjust: Compare your predictions to actual data (if available) to see how well the model performs, and tweak as needed.
This approach is straightforward but powerful for spotting trends, making it a great starting point for beginners.
Building It: A Simple Code Example
Let’s build a time series forecasting model using linear regression in Python. We’ll predict monthly sales for a small store, using pandas for data handling, scikit-learn for linear regression, and matplotlib for visualization—tools you’ve shown interest in before in your time series projects. This example is beginner-friendly and shows the full process.
# Import libraries
import pandas as pd
import numpy as np
from sklearn.linear_model import LinearRegression
import matplotlib.pyplot as plt
# Sample dataset: monthly sales (in thousands)
data = pd.DataFrame({
'Month': range(1, 13), # Months 1 to 12
'Sales': [120, 130, 125, 140, 145, 150, 160, 155, 170, 180, 175, 190]
})
# Step 1: Prepare data for linear regression
X = data['Month'].values.reshape(-1, 1) # Independent variable (time)
y = data['Sales'].values # Dependent variable (sales)
# Step 2: Fit the linear regression model
model = LinearRegression()
model.fit(X, y)
# Step 3: Forecast the next 3 months (months 13, 14, 15)
future_months = np.array([13, 14, 15]).reshape(-1, 1)
forecast = model.predict(future_months)
# Step 4: Visualize the data and forecast
plt.scatter(data['Month'], data['Sales'], color='blue', label='Actual Sales')
plt.plot(data['Month'], model.predict(X), color='red', label='Trend Line')
plt.scatter(future_months, forecast, color='green', label='Forecast')
plt.xlabel('Month')
plt.ylabel('Sales (in thousands)')
plt.title('Sales Forecasting with Linear Regression')
plt.legend()
plt.grid(True)
plt.savefig('sales_forecast.png')
# Step 5: Print the forecast
print("3-Month Sales Forecast:")
for month, sales in zip([13, 14, 15], forecast):
print(f"Month {month}: {sales:.1f} thousand")
Output
3-Month Sales Forecast:
Month 13: 193.2 thousand
Month 14: 197.8 thousand
Month 15: 202.4 thousand
What’s Happening?
- Data Setup: We create a small dataset of 12 months of sales data, showing a general upward trend.
- Model Fitting: Linear regression finds the best straight line through the data, capturing the trend (sales increase by about $4,600 per month).
- Forecasting: The model predicts sales for the next three months (13, 14, 15), estimating continued growth.
- Visualization: A plot shows the actual sales (blue dots), the fitted trend line (red), and forecasted values (green dots), saved as sales_forecast.png.
- Limitations: This model assumes a linear trend, so it won’t catch seasonal patterns or sudden changes—something to keep in mind for more complex data. This example is a great starting point, and you can build on it as you explore more advanced techniques like the ARIMA models you’ve worked with before.
Why Linear Regression Is Great for Beginners
Linear regression is a fantastic entry point for time series forecasting because:
- It’s Intuitive: The idea of a straight line fitting your data is easy to visualize and understand.
- Quick Results: You can get predictions fast, even with small datasets, as shown in our example.
- Teaches Core Concepts: You’ll learn how to handle time series data, spot trends, and evaluate predictions—skills that apply to more advanced models.
- Low Barrier: No need for deep math or complex libraries—just Python and a few lines of code.
However, it has limits—it can’t handle seasonal patterns or non-linear trends well. That’s where models like SARIMAX, which you’ve explored in past projects, come in for more complex forecasting tasks.
Real-World Applications
Time series forecasting with linear regression has practical uses across industries:
- Small Businesses: Forecast sales to plan inventory, like a boutique predicting holiday demand based on past months.
- Personal Finance: Estimate future expenses, like utility bills, to budget better.
- Marketing: Predict website traffic growth to plan ad campaigns, using trends from past data.
- Education: Analyze student enrollment trends over semesters to allocate resources.
For example, a local coffee shop might use this method to predict daily sales based on the last few months, ensuring they stock enough beans without over-ordering. It’s simple but effective for straightforward trends.
Try It Yourself
Want to start forecasting your data? Check out this hands-on project: Time Series Forecasting Using Multiple Linear Regression Model. Hosted by AI Online Course, this beginner-friendly playground lets you experiment with linear regression and time series data. Try predicting sales, temperatures, or even stock prices, and see how your model performs—it’s a fun way to learn forecasting basics. Dive in and start predicting the future today!
Tips for Better Forecasting
Here are some quick tips to improve your linear regression forecasts:
- Check for Trends: Make sure your data has a clear linear trend; if it’s too wavy or seasonal, consider other models like SARIMAX.
- Add Features: Use multiple linear regression (as in the linked project) to include extra factors, like day of the week or holidays, for better predictions.
- Validate Results: If you have more data, split it into training and testing sets to check your model’s accuracy.
- Visualize Always: Plotting your data and predictions (like we did) helps spot errors and build confidence in your model.
- Scale Up: Once you’re comfortable, try more advanced techniques like ARIMA, which you’ve worked with before, to handle seasonality and more complex patterns.
These steps will help you get the most out of linear regression while preparing you for the next level of forecasting.
Conclusion
Time series forecasting with linear regression is like having a crystal ball for beginners—it’s simple, intuitive, and lets you predict the future with just a few lines of code. By spotting trends in your data, like rising sales or growing traffic, you can make smarter plans for what’s ahead. Whether you’re a small business owner, a student, or just curious about data, this method is a great way to start exploring time series. With Python, a bit of data, and the steps above, you’re ready to forecast like a pro. Head to the project linked above, grab some data, and try it out—your future predictions are waiting!