SQL: The Secret Weapon Behind Data Analytics and Data Science
In today’s era, businesses, companies and organisations thrive more on data insights. Whether it’s understanding customer behaviour, staff behaviour, analysing the products and services in markets, optimising operations, or predicting future trends, data is at the heart of strategic decision-making. Behind the scenes of every impactful data story lies a powerful yet often underrated tool: SQL Exactly! What Exactly is SQL? SQL's full name is Structured Query Language, it's like the Swiss Army knife for anyone working with data.SQL lets you retrieve exactly the data one needs, organise and clean it, and get it ready for analysis. Whether a data analyst building reports or a data scientist preparing a machine learning model, chances are SQL is involved every in each step . Structured Query Language (SQL) is used to communicate with databases. SQL can’t build a website, but it is powerful for working with data in databases. On a practical level, what matters most is that SQL can help you complete the data analysis job. SQL is used to access, manipulate, and retrieve data from objects in a database. 1. SQL Makes Teamwork Manageable Even if you're not a programmer, you can usually understand what a SQL query is doing. This makes it a great language for collaborating between data teams, product managers, and business stakeholders, leading to easy understanding in businesses, organisations and companies. Whether you're debugging a data issue with engineers or sharing a report with executives, SQL acts as a common language that bridges technical and non-technical gaps. It makes conversations clearer, faster, and more productive, hence teamwork. 2. It Speaks the Universal Language of Data No matter what database or company a data analyst or a data scientist is working for, SQL is used. It's the common thread among Mysql, Postgresql, BigQuery, Redshift, Snowflake and many databases. This compatibility means that advantage of learning SQL is,one can work across a wide variety of tools and platforms with minimal additional training and less supervision. Either joining a startup organisation, company or a Fortune company, SQL skills will transfer seamlessly, hence opening doors in virtually any data-related role. 3.Exploring Data To easily find out what happened during the last years, why sales reduced during a certain period of time, SQL helps an analyst slice and dice data in seconds. Calculating statistics like averages, counts, and totals, identifying outliers that might skew your results, and merging multiple datasets to uncover deeper patterns. With just a few well written queries, SQL lets you go from a sea of raw numbers to clear, understandable data easily. 4. Handles large data sets SQL is designed to work within databases, which means it can process large amounts of data much faster than trying to do the same thing in Excel or even Python. When dealing with millions of records, SQL enables an analyst to run complex joins, aggregations, and transformations with minimal lag. And with modern cloud data warehouses, it’s now easier than ever to analyze big data without needing a supercomputer or a data engineering team. 5.BI Tools Run on SQL BI tools such as Tableau, Power BI, and Looker might look user-friendly, but they are using SQL. Knowing how to write SQL queries gives an analyst easier ways during building dashboards and reports. However, SQL also allows an analyst to customize metrics, control how data is aggregated, and troubleshoot issues when charts project a negative(-ve) outcomes .SQL transforms BI tools into powerful, decision-driving tools. USE OF SQL IN DATA ANALYTICS SQL is used to build dashboards that stakeholders will rely on in every meeting. AN analyst will use SQL to create reports that spark real decisions. SQL can uncover patterns like customer churn, pinpoint exactly where users drop off in a sales funnel, and break down revenue by product line, region, or even customer segment. It’s the background for storytelling with data. SQL becomes the heartbeat of your analytics workflow, driving clarity, confidence, and action across the organisation. USE OF SQL IN DATA SCIENCE SQL is a mandatory tool to get clean, relevant data for your machine learning model. It helps to engineer features that matter, like time-based user behaviour, frequency patterns, or categorical groupings, which can make or break your model’s performance. And once your model is built, SQL plays a critical role in validating your predictions, hence letting you quickly compare predicted vs. actual outcomes and dig into performance across different segments. In short, SQL turns the often painful, time-consuming data wrangling phase, which eats up most data science work, into something manageable, transparent, and even a bit enjoyable. CONCLUSION SQL is fast, powerful and gets the job done. It delivers results. learning SQL is essent
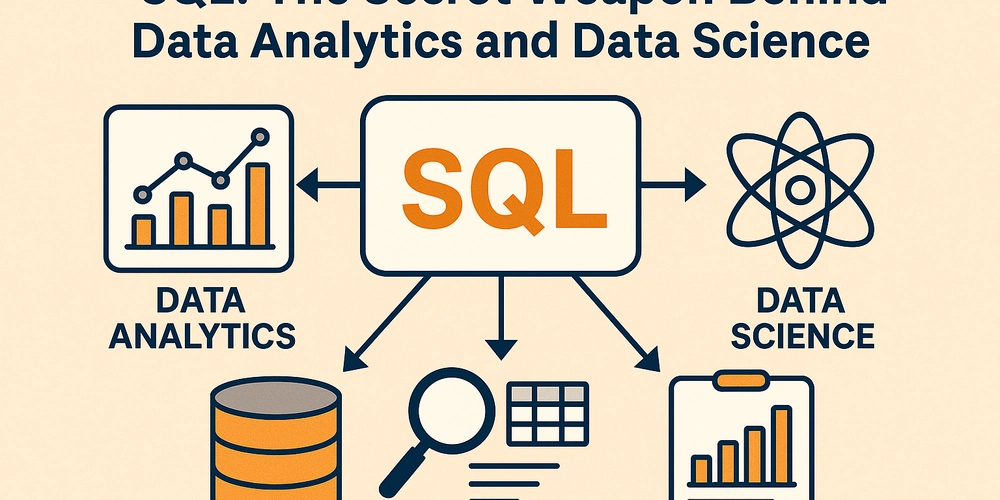
In today’s era, businesses, companies and organisations thrive more on data insights. Whether it’s understanding customer behaviour, staff behaviour, analysing the products and services in markets, optimising operations, or predicting future trends, data is at the heart of strategic decision-making. Behind the scenes of every impactful data story lies a powerful yet often underrated tool: SQL
Exactly! What Exactly is SQL?
SQL's full name is Structured Query Language, it's like the Swiss Army knife for anyone working with data.SQL lets you retrieve exactly the data one needs, organise and clean it, and get it ready for analysis. Whether a data analyst building reports or a data scientist preparing a machine learning model, chances are SQL is involved every in each step . Structured Query Language (SQL) is used to communicate with databases. SQL can’t build a website, but it is powerful for working with data in databases. On a practical level, what matters most is that SQL can help you complete the data analysis job. SQL is used to access, manipulate, and retrieve data from objects in a database.
1. SQL Makes Teamwork Manageable
Even if you're not a programmer, you can usually understand what a SQL query is doing. This makes it a great language for collaborating between data teams, product managers, and business stakeholders, leading to easy understanding in businesses, organisations and companies. Whether you're debugging a data issue with engineers or sharing a report with executives, SQL acts as a common language that bridges technical and non-technical gaps. It makes conversations clearer, faster, and more productive, hence teamwork.
2. It Speaks the Universal Language of Data
No matter what database or company a data analyst or a data scientist is working for, SQL is used. It's the common thread among Mysql, Postgresql, BigQuery, Redshift, Snowflake and many databases. This compatibility means that advantage of learning SQL is,one can work across a wide variety of tools and platforms with minimal additional training and less supervision. Either joining a startup organisation, company or a Fortune company, SQL skills will transfer seamlessly, hence opening doors in virtually any data-related role.
3.Exploring Data
To easily find out what happened during the last years, why sales reduced during a certain period of time, SQL helps an analyst slice and dice data in seconds. Calculating statistics like averages, counts, and totals, identifying outliers that might skew your results, and merging multiple datasets to uncover deeper patterns. With just a few well written queries, SQL lets you go from a sea of raw numbers to clear, understandable data easily.
4. Handles large data sets
SQL is designed to work within databases, which means it can process large amounts of data much faster than trying to do the same thing in Excel or even Python. When dealing with millions of records, SQL enables an analyst to run complex joins, aggregations, and transformations with minimal lag. And with modern cloud data warehouses, it’s now easier than ever to analyze big data without needing a supercomputer or a data engineering team.
5.BI Tools Run on SQL
BI tools such as Tableau, Power BI, and Looker might look user-friendly, but they are using SQL. Knowing how to write SQL queries gives an analyst easier ways during building dashboards and reports. However, SQL also allows an analyst to customize metrics, control how data is aggregated, and troubleshoot issues when charts project a negative(-ve) outcomes .SQL transforms BI tools into powerful, decision-driving tools.
USE OF SQL IN DATA ANALYTICS
SQL is used to build dashboards that stakeholders will rely on in every meeting. AN analyst will use SQL to create reports that spark real decisions. SQL can uncover patterns like customer churn, pinpoint exactly where users drop off in a sales funnel, and break down revenue by product line, region, or even customer segment. It’s the background for storytelling with data. SQL becomes the heartbeat of your analytics workflow, driving clarity, confidence, and action across the organisation.
USE OF SQL IN DATA SCIENCE
SQL is a mandatory tool to get clean, relevant data for your machine learning model. It helps to engineer features that matter, like time-based user behaviour, frequency patterns, or categorical groupings, which can make or break your model’s performance. And once your model is built, SQL plays a critical role in validating your predictions, hence letting you quickly compare predicted vs. actual outcomes and dig into performance across different segments. In short, SQL turns the often painful, time-consuming data wrangling phase, which eats up most data science work, into something manageable, transparent, and even a bit enjoyable.
CONCLUSION
SQL is fast, powerful and gets the job done. It delivers results. learning SQL is essential. It’s the foundation every data professional stands on.
So next time you're wrangling messy spreadsheets, trying to make sense of data silos, or waiting for a report to load, remember: SQL is your secret weapon. And it's ready when you are.
SQL NICKNAMES
- 1. Sequel
- 2.ess cue el
REFERENCE
- SQL For Data Analysis by Cathy Taimura