Reinforcement Learning for Email Agents: OpenPipe’s ART·E Outperforms o3 in Accuracy, Latency, and Cost
OpenPipe has introduced ART·E (Autonomous Retrieval Tool for Email), an open-source research agent designed to answer user questions based on inbox contents with a focus on accuracy, responsiveness, and computational efficiency. ART·E demonstrates the practical utility of reinforcement learning (RL) in fine-tuning large language model (LLM) agents for specialized, high-signal use cases. Addressing Limitations in […] The post Reinforcement Learning for Email Agents: OpenPipe’s ART·E Outperforms o3 in Accuracy, Latency, and Cost appeared first on MarkTechPost.
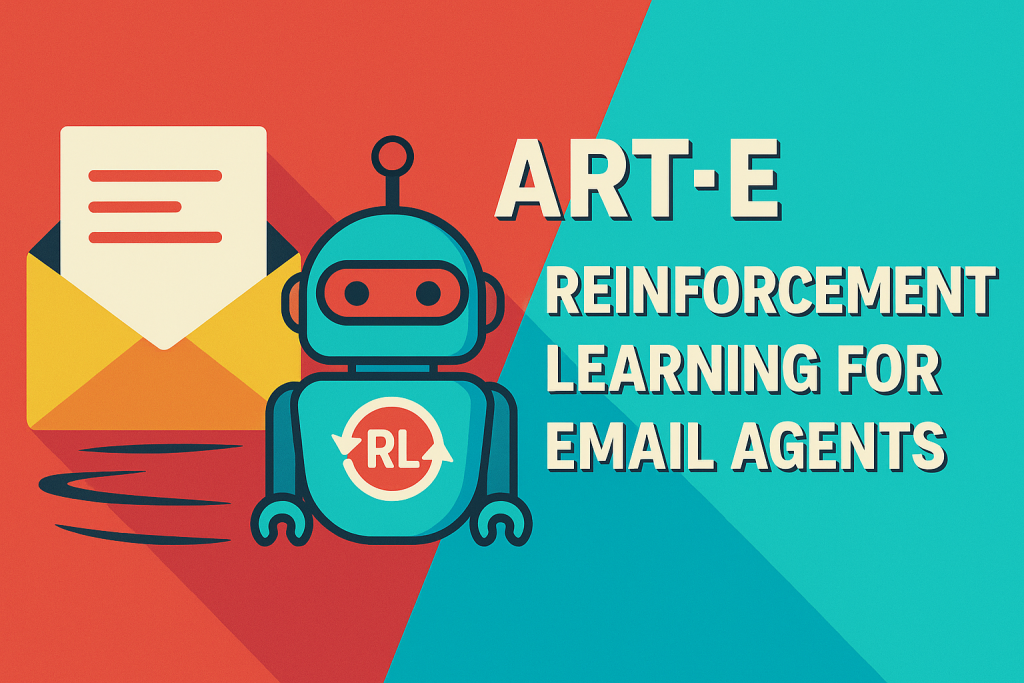
OpenPipe has introduced ART·E (Autonomous Retrieval Tool for Email), an open-source research agent designed to answer user questions based on inbox contents with a focus on accuracy, responsiveness, and computational efficiency. ART·E demonstrates the practical utility of reinforcement learning (RL) in fine-tuning large language model (LLM) agents for specialized, high-signal use cases.
Addressing Limitations in Email-Centric Agent Workflows
Despite significant advances in retrieval-augmented generation (RAG), current LLM-based agents often exhibit inefficiencies when applied to structured personal data such as emails. Existing approaches tend to rely on generic prompting and multi-tool execution, leading to:
- Increased latency due to excessive processing steps
- High inference costs, particularly when using proprietary models
- Variable accuracy caused by ambiguity in email content and intent
The objective behind ART·E is to investigate whether reinforcement learning techniques, in combination with curated data and domain-focused design, can improve agent effectiveness across these dimensions.
ART·E: Architecture and Reinforcement Learning Workflow
OpenPipe developed ART·E as a lightweight email question-answering agent that integrates retrieval and generation with a streamlined decision policy. It is trained using a reinforcement learning setup, following a Proximal Policy Optimization (PPO) regime after initial supervised fine-tuning. The core components include:
- Retriever Module: Identifies relevant emails using embeddings derived from compact, efficient encoders.
- LLM Policy Head: Generates responses informed by the retrieved content, optimized through iterative RL based on feedback signals.
- Evaluation Pipeline: Implements automated correctness evaluation and utility scoring to guide learning during the RL phase.
This architecture supports modularity, allowing independent improvements or substitutions of retrievers, evaluators, or policy heads.


Evaluation: ART·E Compared to o3 Agent
Benchmarking against OpenAI’s o3 agent on real-world email queries, ART·E demonstrates:
Metric o3 Agent ART·E Agent Response Accuracy Baseline +12.4% Average Latency 1.0x 0.2x (5× faster) Inference Cost 1.0x 0.016x (64× cheaper)
These gains result from a tailored execution path, reduced reliance on external API calls, and a narrower, more relevant context window. The cost-performance tradeoff is particularly favorable for users deploying agents at scale or within privacy-sensitive environments.
Open-Source Release and Integration Potential
The ART·E codebase is publicly available on GitHub, offering an extensible platform for further research and practical deployments. Key features of the repository include:
- A configurable evaluator with built-in feedback collection tools
- Abstractions for retriever and language model components
- Interfaces for connecting to common email providers
- Training scripts supporting both supervised learning and RL via the
trlx
library
This release provides a reproducible framework for applying RLHF in agent design across adjacent domains.
Broader Implications: RLHF in Narrow Agent Tasks
While RLHF is traditionally associated with alignment in general-purpose LLMs, ART·E exemplifies its applicability in narrow, goal-oriented tasks. In constrained domains such as email summarization or question answering, reinforcement learning enables agents to:
- Execute more targeted and efficient retrievals
- Develop preference-aware response policies
- Maintain robustness in noisy or partially structured data environments
The ART·E training methodology thus offers a compelling path forward for organizations aiming to optimize LLM-based agents for vertical-specific workflows.
Conclusion
ART·E represents a technically grounded application of RL in agent development, targeting a clearly defined, practical problem space. Its performance improvements across accuracy, latency, and cost metrics highlight the value of integrating reinforcement learning with domain-aware system design. As interest in domain-specialized AI agents continues to grow, ART·E serves as a reproducible and extensible example for future research and development.
Check out the GitHub Page and Technical details. Also, don’t forget to follow us on Twitter and join our Telegram Channel and LinkedIn Group. Don’t Forget to join our 90k+ ML SubReddit.