Integrating AI in QE: Best Practices for Intelligent Test Strategies
As per research, by 2025, approximately 80% of the software engineering tasks will be carried out by the AI platform. This will in turn change the IT landscape within enterprises. More applications will be worked out by AI testing services and more amount of code will be generated. This new paradigm will change how we approach quality and what we test in software development. To ensure reliable and robust software products and stay competitive, the platform of Quality Engineering (QE) is brought into perspective. Furthermore, integrating AI in QE is considered to be a strategic and wise move. In this article, you will get to know the significance of integrating AI in QE. The tactical role of Artificial Intelligence (AI) in Quality Engineering (QE): Continuous advancements, DevOps and agile platforms in technology have led to significant transformations in the landscape of deployment and software engineering. Testing and development teams, particularly Software Development and Test Engineers (SDETs), grapple with the continued demand for frequent and swift releases. Independent Software Vendors (ISVs) are interested in integrating hyperautomation into their entire product development lifecycle. However, when a specific speed limit is reached by traditional development practices, there is a strategic need for ISVs to adopt AI testing services and Machine Learning (ML). Through this integration, the establishment of development paradigms and autonomous processes is facilitated. In order to make sure that superior digital products are created at an accelerated pace, incorporating AI in QE is considered to be a good practice. In an enterprise digital strategy, modernizing quality engineering is considered to be a key activity. This in turn helps in assisting with predictive analytics, data generation, validation, risk-based and model-based testing tasks. Advanced capabilities are introduced by AI powered QE platform that enhance the effectiveness, efficiency and reliability of the QA process. *Following are a few key strategies for the tactful use of Artificial intelligence in quality engineering: * Self-learning and intelligent test scripts: SDETs can relieve themselves of tedious tasks, enhance test coverage and test fast through the automated test scripts. Consider a scenario wherein test scripts for automation need not be specifically changed or created along the way and hence, by learning, they could scale on their own from similar scenarios. Through the machine learning platform, the system is able to understand how test cases are automated by humans depending on scenarios that can be mimicked by the AI testing services platform. Conversational tools like AI chatbots can constantly work with both development teams and stakeholders so that the feedback can be understood and communicated to the concerned teams. Improving granularity and testing speed with AI: There are platforms and test automation tools available that can enable QA professionals to speed up agility and easily automate test cases in software testing. But, there is an increase in complexity, when products grow larger. Considerations for interfaces, integrations and modular architecture are taken into account by test strategies. Existing software test automation platforms can be stretched by this complexity. Improve estimations and quality: AI’s ability can be leveraged by software engineering teams so that a vast pool of data can be scanned and inferred instantly. Proper budgeting can be done through development and testing efforts. The most accurate timelines can be estimated by the AI platform for the completion of different stages of QA for a specific software product development project. A better sense of effort and time can be gained by Engineering VPs to deliver a product of desired quality. This can be taken after factoring in the testing, development and rework effort. These insights are provided by AI through analyzing historical data. This, in turn, helps organizations to prepare billing processes and transparent budgeting. Hence, AI powered QE is a strategic and feasible platform. Conclusion: If you are looking forward to implementing AI testing services for your specific project, then do get connected with a leading software testing services company that will provide you with structured testing solutions that are in line with your project specific requirements.
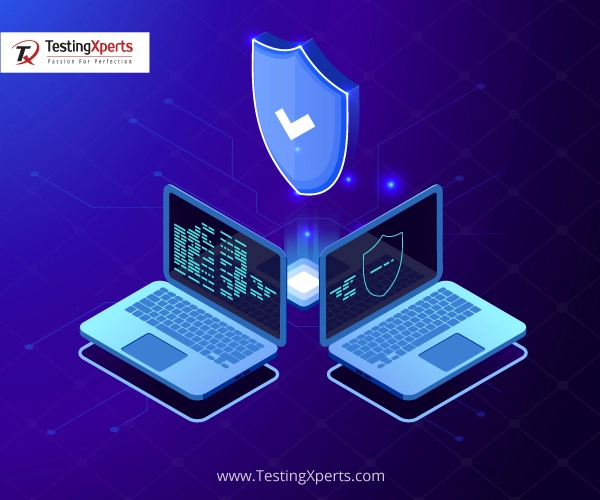
As per research, by 2025, approximately 80% of the software engineering tasks will be carried out by the AI platform. This will in turn change the IT landscape within enterprises. More applications will be worked out by AI testing services and more amount of code will be generated. This new paradigm will change how we approach quality and what we test in software development. To ensure reliable and robust software products and stay competitive, the platform of Quality Engineering (QE) is brought into perspective. Furthermore, integrating AI in QE is considered to be a strategic and wise move. In this article, you will get to know the significance of integrating AI in QE.
The tactical role of Artificial Intelligence (AI) in Quality Engineering (QE):
Continuous advancements, DevOps and agile platforms in technology have led to significant transformations in the landscape of deployment and software engineering. Testing and development teams, particularly Software Development and Test Engineers (SDETs), grapple with the continued demand for frequent and swift releases. Independent Software Vendors (ISVs) are interested in integrating hyperautomation into their entire product development lifecycle.
However, when a specific speed limit is reached by traditional development practices, there is a strategic need for ISVs to adopt AI testing services and Machine Learning (ML). Through this integration, the establishment of development paradigms and autonomous processes is facilitated. In order to make sure that superior digital products are created at an accelerated pace, incorporating AI in QE is considered to be a good practice.
In an enterprise digital strategy, modernizing quality engineering is considered to be a key activity. This in turn helps in assisting with predictive analytics, data generation, validation, risk-based and model-based testing tasks. Advanced capabilities are introduced by AI powered QE platform that enhance the effectiveness, efficiency and reliability of the QA process.
*Following are a few key strategies for the tactful use of Artificial intelligence in quality engineering: *
- Self-learning and intelligent test scripts: SDETs can relieve themselves of tedious tasks, enhance test coverage and test fast through the automated test scripts. Consider a scenario wherein test scripts for automation need not be specifically changed or created along the way and hence, by learning, they could scale on their own from similar scenarios.
Through the machine learning platform, the system is able to understand how test cases are automated by humans depending on scenarios that can be mimicked by the AI testing services platform. Conversational tools like AI chatbots can constantly work with both development teams and stakeholders so that the feedback can be understood and communicated to the concerned teams.
Improving granularity and testing speed with AI: There are platforms and test automation tools available that can enable QA professionals to speed up agility and easily automate test cases in software testing. But, there is an increase in complexity, when products grow larger. Considerations for interfaces, integrations and modular architecture are taken into account by test strategies. Existing software test automation platforms can be stretched by this complexity.
Improve estimations and quality: AI’s ability can be leveraged by software engineering teams so that a vast pool of data can be scanned and inferred instantly. Proper budgeting can be done through development and testing efforts. The most accurate timelines can be estimated by the AI platform for the completion of different stages of QA for a specific software product development project.
A better sense of effort and time can be gained by Engineering VPs to deliver a product of desired quality. This can be taken after factoring in the testing, development and rework effort. These insights are provided by AI through analyzing historical data. This, in turn, helps organizations to prepare billing processes and transparent budgeting. Hence, AI powered QE is a strategic and feasible platform.
Conclusion: If you are looking forward to implementing AI testing services for your specific project, then do get connected with a leading software testing services company that will provide you with structured testing solutions that are in line with your project specific requirements.