Exploring Federated AI for Decentralized Data Training
Introduction: As data continues to grow exponentially, it has become a challenge for traditional centralized AI systems to efficiently process and train large datasets. The rise of federated AI, also known as decentralized AI, offers a promising solution to these challenges. In this article, we will explore the advantages, disadvantages, and features of federated AI for decentralized data training. Advantages: One of the major advantages of federated AI is its ability to train models on decentralized data without the need for data sharing. This eliminates the privacy concerns that come with sharing sensitive data. Additionally, decentralized data training allows for increased collaboration between different organizations or parties without compromising their data. Another advantage is the ability to train models on data from various sources, making the training process more comprehensive and accurate. With this approach, the AI model can learn from diverse datasets, leading to better performance and generalization. Disadvantages: One of the main challenges of federated AI is the complexity of implementing and managing the federated learning process. It requires specialized tools, techniques, and expertise to set up a secure and efficient federated AI system. Moreover, since the data is distributed across multiple parties, it can be challenging to ensure data quality and consistency. This can potentially lead to biased or inaccurate models. Features: Federated AI offers unique features that set it apart from centralized AI. It utilizes techniques such as differential privacy, secure aggregation, and homomorphic encryption to ensure privacy and security of the data during training. Additionally, the model updates its parameters locally without the need for a central server, making it more resilient to system failures. Conclusion: Federated AI for decentralized data training has emerged as a promising approach to address the challenges of processing and training large datasets. It offers a balance between privacy, collaboration, and accuracy, making it a valuable tool for various industries. However, it is still in its early stages and requires further research and development to fully harness its potential. As the field of AI continues to evolve, federated AI is expected to play a significant role in shaping the future of data training.
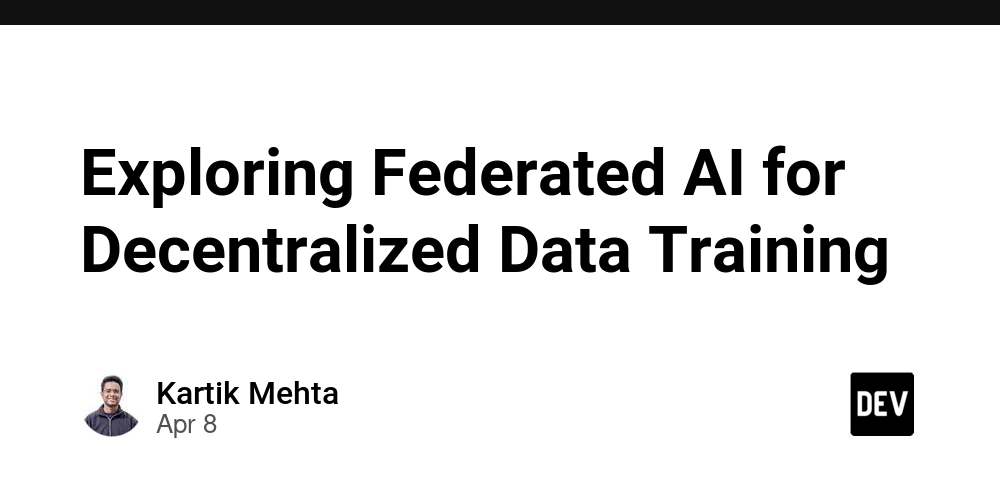
Introduction:
As data continues to grow exponentially, it has become a challenge for traditional centralized AI systems to efficiently process and train large datasets. The rise of federated AI, also known as decentralized AI, offers a promising solution to these challenges. In this article, we will explore the advantages, disadvantages, and features of federated AI for decentralized data training.
Advantages:
One of the major advantages of federated AI is its ability to train models on decentralized data without the need for data sharing. This eliminates the privacy concerns that come with sharing sensitive data. Additionally, decentralized data training allows for increased collaboration between different organizations or parties without compromising their data.
Another advantage is the ability to train models on data from various sources, making the training process more comprehensive and accurate. With this approach, the AI model can learn from diverse datasets, leading to better performance and generalization.
Disadvantages:
One of the main challenges of federated AI is the complexity of implementing and managing the federated learning process. It requires specialized tools, techniques, and expertise to set up a secure and efficient federated AI system.
Moreover, since the data is distributed across multiple parties, it can be challenging to ensure data quality and consistency. This can potentially lead to biased or inaccurate models.
Features:
Federated AI offers unique features that set it apart from centralized AI. It utilizes techniques such as differential privacy, secure aggregation, and homomorphic encryption to ensure privacy and security of the data during training. Additionally, the model updates its parameters locally without the need for a central server, making it more resilient to system failures.
Conclusion:
Federated AI for decentralized data training has emerged as a promising approach to address the challenges of processing and training large datasets. It offers a balance between privacy, collaboration, and accuracy, making it a valuable tool for various industries. However, it is still in its early stages and requires further research and development to fully harness its potential. As the field of AI continues to evolve, federated AI is expected to play a significant role in shaping the future of data training.