Analyzing YOLO Architecture: Part 2 - The Neck Component
The Neck Component in YOLO Architecture: Feature Aggregation and Multi-Scale Fusion I'm continuing my deep dive into YOLO architecture components with a new paper that examines the often overlooked but critically important "neck" component. What's the neck component? The neck serves as the bridge between the backbone network (feature extractor) and the head (detection component), performing critical functions including: Feature fusion across different scales Enhancement of information flow between layers Feature refinement Balancing of resolution and semantic information Evolution across YOLO versions My analysis traces the development from: YOLOv1's lack of a dedicated neck component YOLOv2's introduction of the passthrough layer YOLOv3's adoption of Feature Pyramid Networks YOLOv4 and beyond implementing advanced architectures like PANet Series progression This paper is the second in my YOLO architecture series: Part 1: YOLO Backbone Analysis Part 2: YOLO Neck Component I'm working through each major component to provide a comprehensive understanding of modern object detection architectures. Discussion I'd love to hear your thoughts and experiences with YOLO architectures! Have you implemented custom necks or experimented with different feature fusion techniques? What improvements have you seen? Stay tuned for Part 3 where I'll analyze the detection head component!
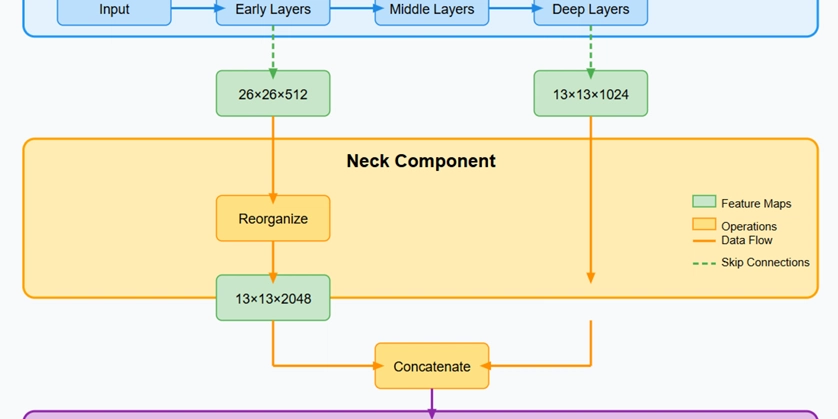
The Neck Component in YOLO Architecture: Feature Aggregation and Multi-Scale Fusion
I'm continuing my deep dive into YOLO architecture components with a new paper that examines the often overlooked but critically important "neck" component.
What's the neck component?
The neck serves as the bridge between the backbone network (feature extractor) and the head (detection component), performing critical functions including:
- Feature fusion across different scales
- Enhancement of information flow between layers
- Feature refinement
- Balancing of resolution and semantic information
Evolution across YOLO versions
My analysis traces the development from:
- YOLOv1's lack of a dedicated neck component
- YOLOv2's introduction of the passthrough layer
- YOLOv3's adoption of Feature Pyramid Networks
- YOLOv4 and beyond implementing advanced architectures like PANet
Series progression
This paper is the second in my YOLO architecture series:
I'm working through each major component to provide a comprehensive understanding of modern object detection architectures.
Discussion
I'd love to hear your thoughts and experiences with YOLO architectures! Have you implemented custom necks or experimented with different feature fusion techniques? What improvements have you seen?
Stay tuned for Part 3 where I'll analyze the detection head component!