Retrieval Metrics Demystified: From BM25 Baselines to EM@5 & Answer F1
“If a fact falls in a database and nobody retrieves it, does it make a sound?” Retrieval‑Augmented Generation (RAG) lives or dies on that first hop—can the system put the right snippets in front of the language model? In this post, we peel back the buzzwords (BM25, EM@5, F1) and show how to turn them into levers you can actually pull. 1. Why bother measuring retrieval separately? End‑to‑end metrics (BLEU, ROUGE, human ratings) blur two questions together: Did I pull the right passages? Did the generator use them well? Untangling the knot matters. If you log a 5‑point jump in answer F1, you want to know where the jump came from—better retrieval, a smarter prompt, or a lucky seed? The retrieval metrics below give you that X‑ray. 2. BM25—the keyword workhorse Before transformers, there was the inverted index: a glorified phonebook where every word points to the documents it lives in. BM25 (“Best Match 25”) is the score those phonebooks still use today: BM25(q,d)=∑t∈qIDF(t) f(t,d)(k1+1)f(t,d)+k1(1−b+b∣d∣∣d∣‾) \operatorname{BM25}(q,d)=\sum_{t\in q} \text{IDF}(t)\;\frac{f(t,d)(k_1+1)}{f(t,d)+k_1\left(1-b+b\frac{|d|}{\overline{|d|}}\right)} BM25(q,d)=t∈q∑IDF(t)f(t,d)+k1(1−b+b∣d∣∣d∣)f(t,d)(k1+1) f(t,d) = term frequency of t in document d |d| = token length of d IDF(t) = inverse document frequency Default hyper‑parameters: k1≈1.2k_1 \approx 1.2k1≈1.2 , b≈0.75b \approx 0.75b≈0.75 Mental model: BM25 is a tug‑of‑war between how often a query word shows up and how common that word is across the whole corpus. Why keep it around? Speed – microseconds per query on millions of docs. Transparency – devs can still debug with Ctrl‑F. Baseline gravity – if you can’t beat BM25, something’s off. 3. EM@k—Exact Match at k Imagine playing Where’s Waldo? but you’re allowed to search the first k pages instead of the whole book. EM@k asks: “Does any of my top‑k passages contain the gold answer string **exactly?” Algorithm for a question set of size NNN : Retrieve top‑k passages per question. Mark hit = 1 if at least one passage contains the gold answer, otherwise 0. EM@k=∑i=1NhitiN\text{EM@k} = \frac{\sum_{i=1}^{N} \text{hit}_i}{N} EM@k=N∑i=1Nhiti Why the fuss over exact match? Because partial overlaps (“2008 financial crash” vs. “the 2008 recession”) are slippery to grade at retrieval time. EM@k stays dumb on purpose—either the string shows up or it doesn’t. Rule‑of‑thumb: EM@5 ≥ 80% → retrieval is likely not your bottleneck. EM@5 ≤ 60% → focus on the retriever before prompt‑tuning. 4. Answer‑level F1—did the generator actually use the context? Once your passages hit the jackpot, the generator still has to say the answer. For extractive QA, the go‑to metric is token‑level F1: F1=2×precision×recallprecision+recall \text{F1} = \frac{2 \times \text{precision} \times \text{recall}}{\text{precision} + \text{recall}} F1=precision+recall2×precision×recall Component Definition Precision Tokens in the model answer ∩ tokens in the gold answer ÷ tokens in the model answer Recall Tokens in the model answer ∩ tokens in the gold answer ÷ tokens in the gold answer F1 forgives small wording tweaks—“Barack Obama” vs. “Obama”—in a way EM cannot. 5. From BM25 to Dense Retrieval & Reranking Stage Model What changes Why you win Dual‑encoder Dense Passage Retriever Index contains 768‑D vectors, not word positions Captures synonyms (“terminate” ≈ “cancel”) Cross‑encoder MiniLM, MonoT5… Re‑score [CLS]q [SEP] d[\text{CLS}] q\;[SEP]\;d[CLS]q[SEP]d with full token interactions Sharp ordering; filters noise A typical contract QA study logged: BM25 → 61% EM@5 DPR + Cross‑encoder → 79% EM@5 Same corpus, same questions—just a richer notion of “relevance”. 6. Other retrieval diagnostics you’ll meet in the wild Metric What it asks Best when… Recall@k Any gold passage in top‑k? Gold labels are full passages, not spans MRR How early is the first correct hit? You care about position 1 above all MAP How well are all relevant docs ranked? Multiple correct passages per query nDCG@k Same as MAP but with graded (0–3) relevance Web search, ad ranking 7. Hands‑on: computing EM@5 in Python from typing import List def em_at_k(retrieved: List[List[str]], gold: List[str], k: int = 5) -> float: """retrieved[i] is the ranked list for question i; gold[i] the gold answer string""" hits = sum(any(gold[i] in doc for doc in retrieved[i][:k]) for i in range(len(gold))) return hits / len(gold) Pro tip: pre‑lowercase and strip punctuation on both sides to avoid false misses. 8. Cheat‑sheet
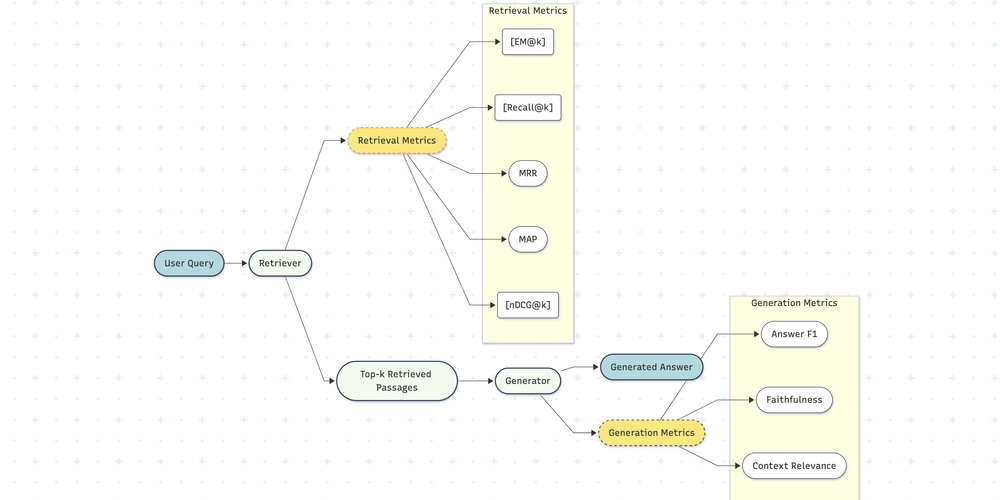
“If a fact falls in a database and nobody retrieves it, does it make a sound?”
Retrieval‑Augmented Generation (RAG) lives or dies on that first hop—can the system put the right snippets in front of the language model?
In this post, we peel back the buzzwords (BM25, EM@5, F1) and show how to turn them into levers you can actually pull.
1. Why bother measuring retrieval separately?
End‑to‑end metrics (BLEU, ROUGE, human ratings) blur two questions together:
- Did I pull the right passages?
- Did the generator use them well?
Untangling the knot matters. If you log a 5‑point jump in answer F1, you want to know where the jump came from—better retrieval, a smarter prompt, or a lucky seed? The retrieval metrics below give you that X‑ray.
2. BM25—the keyword workhorse
Before transformers, there was the inverted index: a glorified phonebook where every word points to the documents it lives in. BM25 (“Best Match 25”) is the score those phonebooks still use today:
- f(t,d) = term frequency of t in document d
- |d| = token length of d
- IDF(t) = inverse document frequency
- Default hyper‑parameters: k1≈1.2k_1 \approx 1.2k1≈1.2 , b≈0.75b \approx 0.75b≈0.75
Mental model: BM25 is a tug‑of‑war between how often a query word shows up and how common that word is across the whole corpus.
Why keep it around?
- Speed – microseconds per query on millions of docs.
- Transparency – devs can still debug with Ctrl‑F.
- Baseline gravity – if you can’t beat BM25, something’s off.
3. EM@k—Exact Match at k
Imagine playing Where’s Waldo? but you’re allowed to search the first k pages instead of the whole book. EM@k asks: “Does any of my top‑k passages contain the gold answer string **exactly?”
Algorithm for a question set of size NNN :
- Retrieve top‑k passages per question.
- Mark hit = 1 if at least one passage contains the gold answer, otherwise 0.
-
EM@k=∑i=1NhitiN\text{EM@k} = \frac{\sum_{i=1}^{N} \text{hit}_i}{N} EM@k=N∑i=1Nhiti
Why the fuss over exact match?
Because partial overlaps (“2008 financial crash” vs. “the 2008 recession”) are slippery to grade at retrieval time. EM@k stays dumb on purpose—either the string shows up or it doesn’t.
Rule‑of‑thumb:
EM@5 ≥ 80% → retrieval is likely not your bottleneck.
EM@5 ≤ 60% → focus on the retriever before prompt‑tuning.
4. Answer‑level F1—did the generator actually use the context?
Once your passages hit the jackpot, the generator still has to say the answer. For extractive QA, the go‑to metric is token‑level F1:
Component | Definition |
---|---|
Precision | Tokens in the model answer ∩ tokens in the gold answer ÷ tokens in the model answer |
Recall | Tokens in the model answer ∩ tokens in the gold answer ÷ tokens in the gold answer |
F1 forgives small wording tweaks—“Barack Obama” vs. “Obama”—in a way EM cannot.
5. From BM25 to Dense Retrieval & Reranking
Stage | Model | What changes | Why you win |
---|---|---|---|
Dual‑encoder | Dense Passage Retriever | Index contains 768‑D vectors, not word positions | Captures synonyms (“terminate” ≈ “cancel”) |
Cross‑encoder | MiniLM, MonoT5… | Re‑score [CLS]q [SEP] d[\text{CLS}] q\;[SEP]\;d[CLS]q[SEP]d with full token interactions | Sharp ordering; filters noise |
A typical contract QA study logged:
- BM25 → 61% EM@5
- DPR + Cross‑encoder → 79% EM@5
Same corpus, same questions—just a richer notion of “relevance”.
6. Other retrieval diagnostics you’ll meet in the wild
Metric | What it asks | Best when… |
---|---|---|
Recall@k | Any gold passage in top‑k? | Gold labels are full passages, not spans |
MRR | How early is the first correct hit? | You care about position 1 above all |
MAP | How well are all relevant docs ranked? | Multiple correct passages per query |
nDCG@k | Same as MAP but with graded (0–3) relevance | Web search, ad ranking |
7. Hands‑on: computing EM@5 in Python
from typing import List
def em_at_k(retrieved: List[List[str]], gold: List[str], k: int = 5) -> float:
"""retrieved[i] is the ranked list for question i; gold[i] the gold answer string"""
hits = sum(any(gold[i] in doc for doc in retrieved[i][:k]) for i in range(len(gold)))
return hits / len(gold)
Pro tip: pre‑lowercase and strip punctuation on both sides to avoid false misses.