OpenAI launches enterprise AI adoption guide with real-world use cases
OpenAI's guide reveals how enterprises use AI tools like GPT-4 for research, task automation, and internal knowledge management.
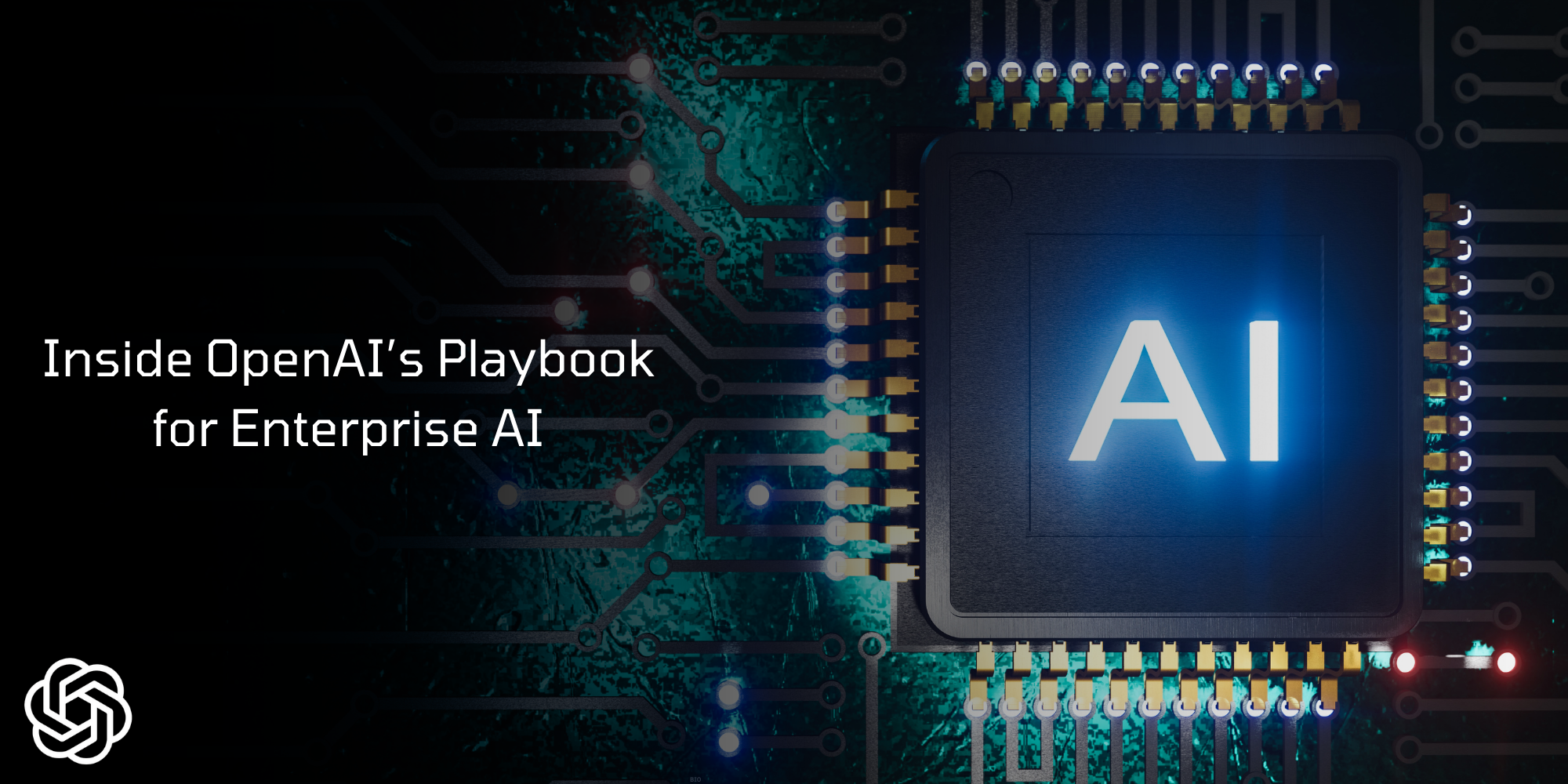
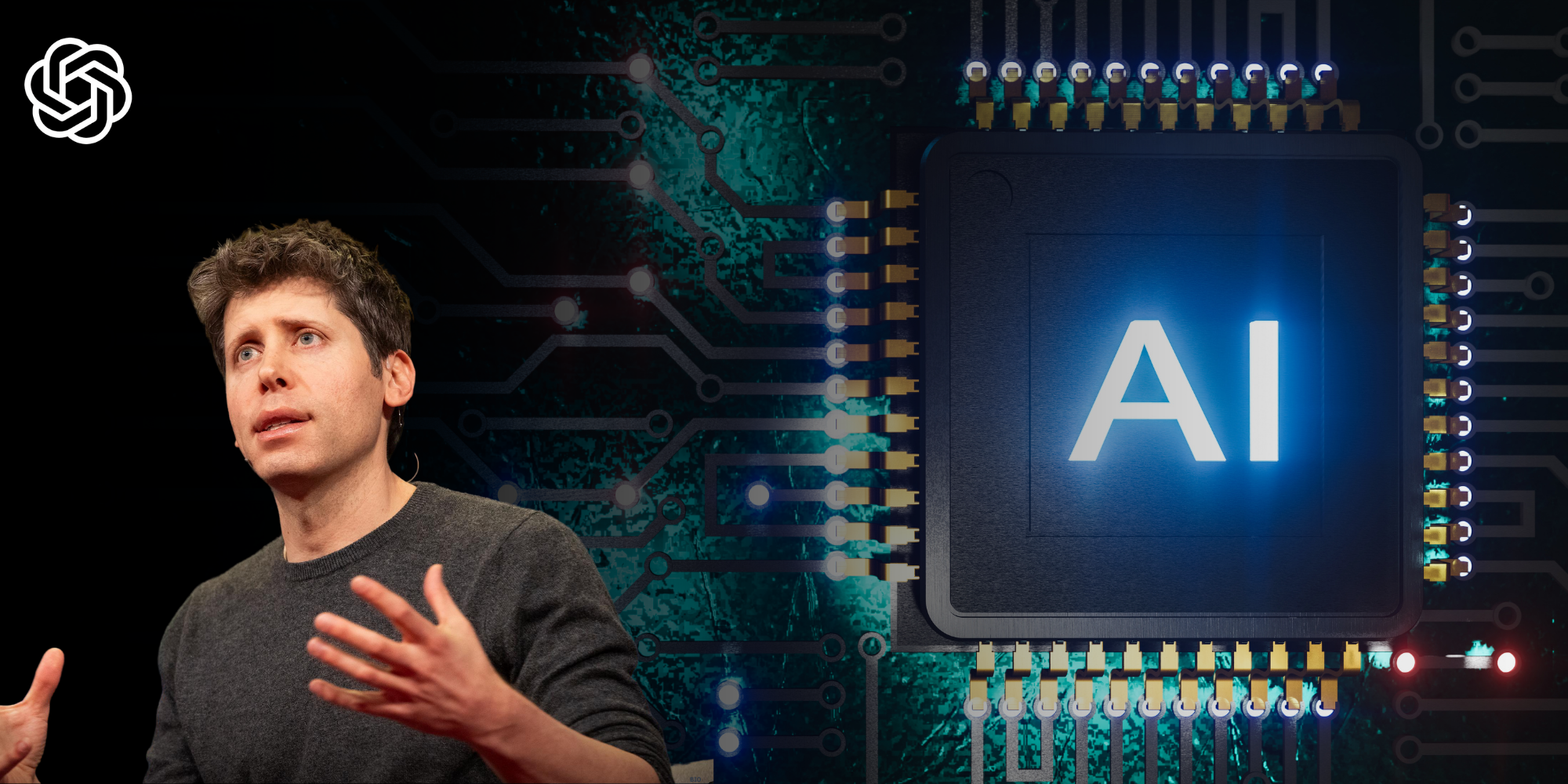
Sam Altman-led OpenAI has released a new document aimed at organisations exploring the adoption of artificial intelligence tools in their operations. The guide, titled AI in the Enterprise, outlines lessons learned from enterprise collaborations and details practical steps for deploying AI systems at scale.
The release was announced on May 3 and is available publicly via OpenAI’s official channels.
Based on field deployments, not theory
The 24-page document compiles implementation strategies based on OpenAI’s work with enterprise clients including Morgan Stanley, Klarna, Mercado Libre, and Lowe’s. Rather than projecting hypothetical use cases, the guide focuses on actual applications of large language models in business environments.
According to OpenAI, the document draws from observed challenges and outcomes in these deployments. It covers a range of topics such as system evaluation, infrastructure setup, model fine-tuning, and internal team coordination. Each recommendation is tied to specific use cases or deployment stages, aiming to provide a structured approach to AI integration.
Example: AI use in financial services
One example cited in the guide is Morgan Stanley’s internal tool powered by GPT-4, which supports financial advisors in retrieving information from proprietary research and summarising client meetings. As reported by Business Insider (May 2025), the tool has been integrated into the firm’s research and advisory workflows and is being used across departments.
Introduction of experimental AI agent
The guide also introduces “Operator,” an experimental AI agent under development at OpenAI. According to All About AI, the agent is designed to perform web-based and internal system tasks with minimal input. While Operator is not yet available for general enterprise use, its inclusion suggests potential directions in automation within enterprise contexts.
Emphasis on early investment and customisation
Among the key points in the document is the recommendation that enterprises invest early and tailor AI systems to their own operational requirements. The guide notes that domain-specific fine-tuning and close collaboration between developers and business teams are often necessary to achieve reliable outputs.
The company also stresses the need for organisations to evaluate model behavior continuously and set realistic expectations around capabilities and limitations.