Nick Kathmann, CISO/CIO at LogicGate – Interview Series
Nicholas Kathmann is the Chief Information Security Officer (CISO) at LogicGate, where he leads the company’s information security program, oversees platform security innovations, and engages with customers on managing cybersecurity risk. With over two decades of experience in IT and 18+ years in cybersecurity, Kathmann has built and led security operations across small businesses and […] The post Nick Kathmann, CISO/CIO at LogicGate – Interview Series appeared first on Unite.AI.
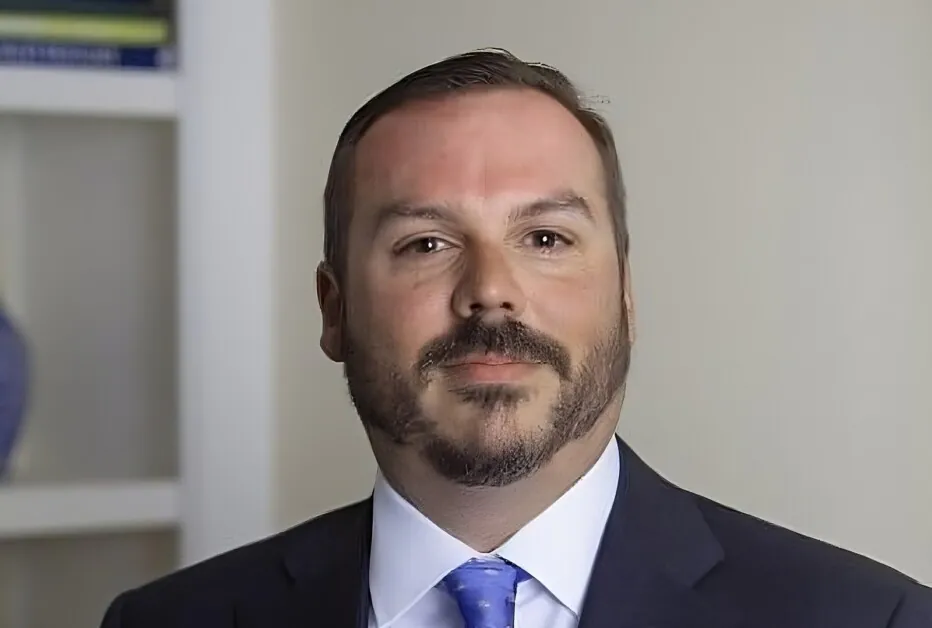
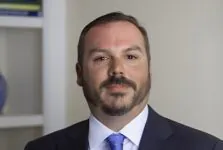
Nicholas Kathmann is the Chief Information Security Officer (CISO) at LogicGate, where he leads the company’s information security program, oversees platform security innovations, and engages with customers on managing cybersecurity risk. With over two decades of experience in IT and 18+ years in cybersecurity, Kathmann has built and led security operations across small businesses and Fortune 100 enterprises.
LogicGate is a risk and compliance platform that helps organizations automate and scale their governance, risk, and compliance (GRC) programs. Through its flagship product, Risk Cloud®, LogicGate enables teams to identify, assess, and manage risk across the enterprise with customizable workflows, real-time insights, and integrations. The platform supports a wide range of use cases, including third-party risk, cybersecurity compliance, and internal audit management, helping companies build more agile and resilient risk strategies
You serve as both CISO and CIO at LogicGate — how do you see AI transforming the responsibilities of these roles in the next 2–3 years?
AI is already transforming both of these roles, but in the next 2-3 years, I think we’ll see a major rise in Agentic AI that has the power to reimagine how we deal with business processes on a day-to-day basis. Anything that would usually go to an IT help desk — like resetting passwords, installing applications, and more — can be handled by an AI agent. Another critical use case will be leveraging AI agents to handle tedious audit assessments, allowing CISOs and CIOs to prioritize more strategic requests.
With federal cyber layoffs and deregulation trends, how should enterprises approach AI deployment while maintaining a strong security posture?
While we’re seeing a deregulation trend in the U.S., regulations are actually strengthening in the EU. So, if you’re a multinational enterprise, anticipate having to comply with global regulatory requirements around responsible use of AI. For companies only operating in the U.S., I see there being a learning period in terms of AI adoption. I think it’s important for those enterprises to form strong AI governance policies and maintain some human oversight in the deployment process, making sure nothing is going rogue.
What are the biggest blind spots you see today when it comes to integrating AI into existing cybersecurity frameworks?
While there are a couple of areas I can think of, the most impactful blind spot would be where your data is located and where it’s traversing. The introduction of AI is only going to make oversight in that area more of a challenge. Vendors are enabling AI features in their products, but that data doesn’t always go directly to the AI model/vendor. That renders traditional security tools like DLP and web monitoring effectively blind.
You’ve said most AI governance strategies are “paper tigers.” What are the core ingredients of a governance framework that actually works?
When I say “paper tigers,” I’m referring specifically to governance strategies where only a small team knows the processes and standards, and they are not enforced or even understood throughout the organization. AI is very pervasive, meaning it impacts every group and every team. “One size fits all” strategies aren’t going to work. A finance team implementing AI features into its ERP is different from a product team implementing an AI feature in a specific product, and the list continues. The core ingredients of a strong governance framework vary, but IAPP, OWASP, NIST, and other advisory bodies have pretty good frameworks for determining what to evaluate. The hardest part is figuring out when the requirements apply to each use case.
How can companies avoid AI model drift and ensure responsible use over time without over-engineering their policies?
Drift and degradation is just part of using technology, but AI can significantly accelerate the process. But if the drift becomes too great, corrective measures will be needed. A comprehensive testing strategy that looks for and measures accuracy, bias, and other red flags is necessary over time. If companies want to avoid bias and drift, they need to start by ensuring they have the tools in place to identify and measure it.
What role should changelogs, limited policy updates, and real-time feedback loops play in maintaining agile AI governance?
While they play a role right now to reduce risk and liability to the provider, real-time feedback loops hamper the ability of customers and users to perform AI governance, especially if changes in communication mechanisms happen too frequently.
What concerns do you have around AI bias and discrimination in underwriting or credit scoring, particularly with “Buy Now, Pay Later” (BNPL) services?
Last year, I spoke to an AI/ML researcher at a large, multinational bank who had been experimenting with AI/LLMs across their risk models. The models, even when trained on large and accurate data sets, would make really surprising, unsupported decisions to either approve or deny underwriting. For example, if the words “great credit” were mentioned in a chat transcript or communications with customers, the models would, by default, deny the loan — regardless of whether the customer said it or the bank employee said it. If AI is going to be relied upon, banks need better oversight and accountability, and those “surprises” need to be minimized.
What’s your take on how we should audit or assess algorithms that make high-stakes decisions — and who should be held accountable?
This goes back to the comprehensive testing model, where it’s necessary to continuously test and benchmark the algorithm/models in as close to real time as possible. This can be difficult, as the model output may have desirable results that will need humans to identify outliers. As a banking example, a model that denies all loans flat out will have a great risk rating, since zero loans it underwrites will ever default. In that case, the organization that implements the model/algorithm should be responsible for the outcome of the model, just like they would be if humans were making the decision.
With more enterprises requiring cyber insurance, how are AI tools reshaping both the risk landscape and insurance underwriting itself?
AI tools are great at disseminating large amounts of data and finding patterns or trends. On the customer side, these tools will be instrumental in understanding the organization’s actual risk and managing that risk. On the underwriter’s side, those tools will be helpful in finding inconsistencies and organizations that are becoming immature over time.
How can companies leverage AI to proactively reduce cyber risk and negotiate better terms in today’s insurance market?
Today, the best way to leverage AI for reducing risk and negotiating better insurance terms is to filter out noise and distractions, helping you focus on the most important risks. If you reduce those risks in a comprehensive way, your cyber insurance rates should go down. It’s too easy to get overwhelmed with the sheer volume of risks. Don’t get bogged down trying to address every single issue when focusing on the most critical ones can have a much larger impact.
What are a few tactical steps you recommend for companies that want to implement AI responsibly — but don’t know where to start?
First, you need to understand what your use cases are and document the desired outcomes. Everyone wants to implement AI, but it’s important to think of your goals first and work backwards from there — something I think a lot of organizations struggle with today. Once you have a good understanding of your use cases, you can research the different AI frameworks and understand which of the applicable controls matter to your use cases and implementation. Strong AI governance is also business critical, for risk mitigation and efficiency since automation is only as useful as its data input. Organizations leveraging AI must do so responsibly, as partners and prospects are asking tough questions around AI sprawl and usage. Not knowing the answer can mean missing out on business deals, directly impacting the bottom line.
If you had to predict the biggest AI-related security risk five years from now, what would it be — and how can we prepare today?
My prediction is that as Agentic AI is built into more business processes and applications, attackers will engage in fraud and misuse to manipulate those agents into delivering malicious outcomes. We have already seen this with the manipulation of customer service agents, resulting in unauthorized deals and refunds. Threat actors used language tricks to bypass policies and interfere with the agent’s decision-making.
Thank you for the great interview, readers who wish to learn more should visit LogicGate.
The post Nick Kathmann, CISO/CIO at LogicGate – Interview Series appeared first on Unite.AI.