InsightFlow Part 6: Implementing ETL Processes with AWS Glue for InsightFlow
InsightFlow GitHub Repo In this post, we’ll explore how AWS Glue was used to implement the ETL (Extract, Transform, Load) processes for the InsightFlow project. AWS Glue provides a serverless, fully managed environment for building and running ETL pipelines, making it an ideal choice for transforming raw data into a structured, queryable format. Why AWS Glue? AWS Glue simplifies the process of building ETL pipelines by offering the following key features: Serverless Architecture: No need to manage infrastructure; Glue automatically provisions resources. Schema Discovery: Automatically detects and catalogs data schemas using the Glue Data Catalog. Integration with AWS Services: Seamlessly integrates with S3, Athena, and other AWS services. Scalability: Automatically scales to handle large datasets. Cost Efficiency: Pay only for the resources used during ETL jobs. For InsightFlow, AWS Glue was used to: Discover and catalog raw data stored in S3. Transform raw data into a structured format. Load the transformed data into a partitioned data warehouse layer in S3. Overview of the ETL Workflow The ETL process in InsightFlow involves the following steps: Extract: Fetch raw data from S3 buckets. Transform: Clean, normalize, and enrich the data using Glue jobs. Load: Write the transformed data back to S3 in a partitioned format for efficient querying with Athena. Key Components Glue Data Catalog: Stores metadata about the raw and transformed datasets. Glue Crawlers: Automatically discover schemas and update the Data Catalog. Glue Jobs: Perform the actual data transformations. Step 1: Setting Up the Glue Data Catalog The Glue Data Catalog acts as a central repository for metadata about the datasets. It enables Athena to query the data without requiring explicit schema definitions. Defining the Glue Database A Glue database was created to organize the tables for the project. Here’s the Terraform configuration: resource "aws_glue_catalog_database" "dbt_database" { name = "insightflow_prod" tags = { Environment = "prod" Project = "InsightFlow" } } Step 2: Discovering Data with Glue Crawlers Glue Crawlers were used to automatically discover the schema of raw data stored in S3 and populate the Data Catalog. Configuring the Glue Crawler The crawler scans the raw S3 bucket and creates tables in the Glue database: resource "aws_glue_crawler" "raw_data_crawler" { name = "insightflow-prod-raw-data-crawler" role = aws_iam_role.glue_crawler_role.arn database_name = aws_glue_catalog_database.dbt_database.name s3_target { path = "s3://insightflow-prod-raw-data/raw/" } schema_change_policy { update_behavior = "UPDATE_IN_DATABASE" delete_behavior = "LOG" } tags = { Environment = "prod" Project = "InsightFlow" } } Running the Crawler The crawler is triggered using the AWS CLI or programmatically via the AWS SDK: aws glue start-crawler --name insightflow-prod-raw-data-crawler Once the crawler completes, the raw data is available as tables in the Glue Data Catalog. Step 3: Transforming Data with Glue Jobs Glue jobs were used to clean, normalize, and enrich the raw data. These jobs are written in PySpark, allowing for scalable, distributed data processing. Example Glue Job: Aggregating Fuel Prices The following Glue job aggregates weekly fuel prices into monthly averages: import sys from pyspark.context import SparkContext from pyspark.sql import SparkSession from pyspark.sql.functions import col, avg, year, month # Initialize Spark session sc = SparkContext() spark = SparkSession(sc) # Read raw fuel price data from S3 raw_data = spark.read.format("parquet").load("s3://insightflow-prod-raw-data/raw/fuelprice/") # Aggregate weekly prices to monthly averages monthly_data = raw_data.groupBy(year("ymd_date").alias("year"), month("ymd_date").alias("month")) \\ .agg( avg("ron95").alias("avg_ron95_price"), avg("ron97").alias("avg_ron97_price"), avg("diesel").alias("avg_diesel_price") ) # Write the transformed data back to S3 monthly_data.write.partitionBy("year", "month").parquet("s3://insightflow-prod-processed-data/fuelprice_monthly/") Scheduling Glue Jobs Glue jobs can be scheduled to run at regular intervals using Glue Triggers or external orchestration tools like Kestra. Step 4: Querying Transformed Data with Athena The transformed data is stored in a partitioned format in the processed S3 bucket. Athena queries can leverage these partitions for efficient data retrieval. Example Query Here’s an example query to analyze the correlation between retail sales and fuel prices: SELECT r.year, r.month, r.sales_value_rm_mil, f.avg_ron95_price, f.avg_ron97_price, f.avg_diesel
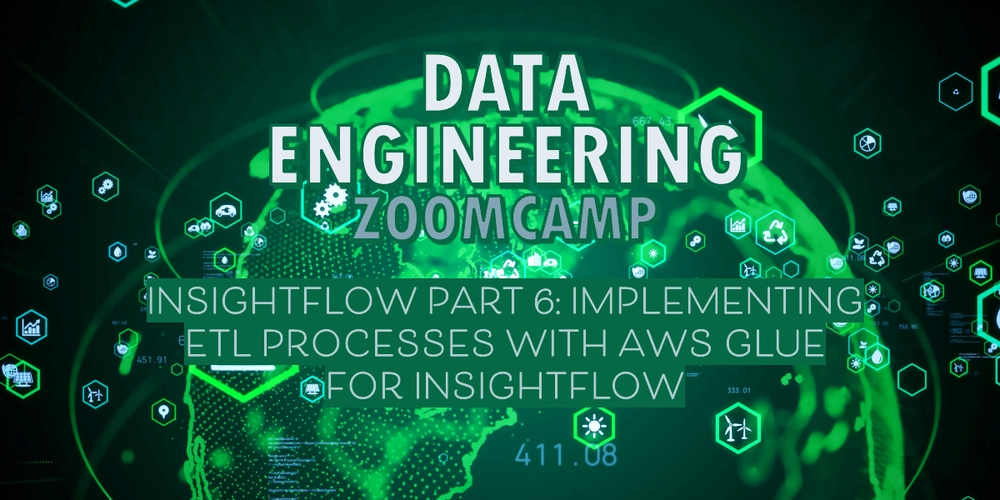
In this post, we’ll explore how AWS Glue was used to implement the ETL (Extract, Transform, Load) processes for the InsightFlow project. AWS Glue provides a serverless, fully managed environment for building and running ETL pipelines, making it an ideal choice for transforming raw data into a structured, queryable format.
Why AWS Glue?
AWS Glue simplifies the process of building ETL pipelines by offering the following key features:
- Serverless Architecture: No need to manage infrastructure; Glue automatically provisions resources.
- Schema Discovery: Automatically detects and catalogs data schemas using the Glue Data Catalog.
- Integration with AWS Services: Seamlessly integrates with S3, Athena, and other AWS services.
- Scalability: Automatically scales to handle large datasets.
- Cost Efficiency: Pay only for the resources used during ETL jobs.
For InsightFlow, AWS Glue was used to:
- Discover and catalog raw data stored in S3.
- Transform raw data into a structured format.
- Load the transformed data into a partitioned data warehouse layer in S3.
Overview of the ETL Workflow
The ETL process in InsightFlow involves the following steps:
- Extract: Fetch raw data from S3 buckets.
- Transform: Clean, normalize, and enrich the data using Glue jobs.
- Load: Write the transformed data back to S3 in a partitioned format for efficient querying with Athena.
Key Components
- Glue Data Catalog: Stores metadata about the raw and transformed datasets.
- Glue Crawlers: Automatically discover schemas and update the Data Catalog.
- Glue Jobs: Perform the actual data transformations.
Step 1: Setting Up the Glue Data Catalog
The Glue Data Catalog acts as a central repository for metadata about the datasets. It enables Athena to query the data without requiring explicit schema definitions.
Defining the Glue Database
A Glue database was created to organize the tables for the project. Here’s the Terraform configuration:
resource "aws_glue_catalog_database" "dbt_database" {
name = "insightflow_prod"
tags = {
Environment = "prod"
Project = "InsightFlow"
}
}
Step 2: Discovering Data with Glue Crawlers
Glue Crawlers were used to automatically discover the schema of raw data stored in S3 and populate the Data Catalog.
Configuring the Glue Crawler
The crawler scans the raw
S3 bucket and creates tables in the Glue database:
resource "aws_glue_crawler" "raw_data_crawler" {
name = "insightflow-prod-raw-data-crawler"
role = aws_iam_role.glue_crawler_role.arn
database_name = aws_glue_catalog_database.dbt_database.name
s3_target {
path = "s3://insightflow-prod-raw-data/raw/"
}
schema_change_policy {
update_behavior = "UPDATE_IN_DATABASE"
delete_behavior = "LOG"
}
tags = {
Environment = "prod"
Project = "InsightFlow"
}
}
Running the Crawler
The crawler is triggered using the AWS CLI or programmatically via the AWS SDK:
aws glue start-crawler --name insightflow-prod-raw-data-crawler
Once the crawler completes, the raw data is available as tables in the Glue Data Catalog.
Step 3: Transforming Data with Glue Jobs
Glue jobs were used to clean, normalize, and enrich the raw data. These jobs are written in PySpark, allowing for scalable, distributed data processing.
Example Glue Job: Aggregating Fuel Prices
The following Glue job aggregates weekly fuel prices into monthly averages:
import sys
from pyspark.context import SparkContext
from pyspark.sql import SparkSession
from pyspark.sql.functions import col, avg, year, month
# Initialize Spark session
sc = SparkContext()
spark = SparkSession(sc)
# Read raw fuel price data from S3
raw_data = spark.read.format("parquet").load("s3://insightflow-prod-raw-data/raw/fuelprice/")
# Aggregate weekly prices to monthly averages
monthly_data = raw_data.groupBy(year("ymd_date").alias("year"), month("ymd_date").alias("month")) \\
.agg(
avg("ron95").alias("avg_ron95_price"),
avg("ron97").alias("avg_ron97_price"),
avg("diesel").alias("avg_diesel_price")
)
# Write the transformed data back to S3
monthly_data.write.partitionBy("year", "month").parquet("s3://insightflow-prod-processed-data/fuelprice_monthly/")
Scheduling Glue Jobs
Glue jobs can be scheduled to run at regular intervals using Glue Triggers or external orchestration tools like Kestra.
Step 4: Querying Transformed Data with Athena
The transformed data is stored in a partitioned format in the processed
S3 bucket. Athena queries can leverage these partitions for efficient data retrieval.
Example Query
Here’s an example query to analyze the correlation between retail sales and fuel prices:
SELECT
r.year,
r.month,
r.sales_value_rm_mil,
f.avg_ron95_price,
f.avg_ron97_price,
f.avg_diesel_price
FROM
fct_retail_sales_monthly r
JOIN
fuelprice_monthly f
ON
r.year = f.year AND r.month = f.month
WHERE
r.year = 2025
ORDER BY
r.month;
Challenges and Lessons Learned
-
Schema Evolution: Managing schema changes in Glue required careful configuration of the
schema_change_policy
. - Partitioning: Proper partitioning of the transformed data significantly improved query performance in Athena.
- IAM Permissions: Ensuring the Glue job role had the necessary permissions to access S3 and the Data Catalog was critical.