InsightFlow Part 3: Building the Data Ingestion Layer with AWS Batch
InsightFlow GitHub Repo In this post, we’ll explore how the data ingestion layer for the InsightFlow project was built using AWS Batch. This layer is responsible for fetching raw data from public sources, processing it, and storing it in an S3 data lake for further transformation and analysis. By leveraging AWS Batch, we were able to create a scalable, cost-efficient, and fully managed solution for running containerized ingestion jobs. Why AWS Batch? AWS Batch is a fully managed service that enables you to run batch computing jobs at any scale. It dynamically provisions the optimal compute resources (e.g., Fargate, EC2) based on the volume and requirements of your jobs. For InsightFlow, AWS Batch was the perfect choice because: Scalability: Automatically scales resources to handle varying workloads. Cost Efficiency: Supports Fargate Spot instances for cost savings. Containerized Workflows: Runs containerized Python scripts for ingestion, ensuring consistency across environments. Integration with AWS Services: Natively integrates with S3, CloudWatch, and IAM for seamless data processing and monitoring. Overview of the Ingestion Workflow The ingestion layer is designed to: Fetch Data: Download datasets from public APIs and direct download links (e.g., data.gov.my). Process Data: Perform lightweight transformations, such as renaming columns and converting formats. Store Data: Upload the processed data to an S3 bucket in a structured format (e.g., partitioned by year and month). Key Components AWS Batch Job Definition: Defines the container image, resource requirements, and environment variables for the ingestion job. AWS Batch Job Queue: Manages the execution of jobs and maps them to compute environments. AWS Batch Compute Environment: Specifies the underlying compute resources (e.g., Fargate Spot). Python Ingestion Script: A containerized script that handles data fetching, processing, and uploading to S3. Step 1: Setting Up the Infrastructure The infrastructure for the ingestion layer was provisioned using Terraform. Below are the key resources and their configurations. 1. AWS Batch Compute Environment The compute environment uses Fargate Spot for cost efficiency. Here’s the Terraform configuration: resource "aws_batch_compute_environment" "fargate_spot_ce" { compute_environment_name = "insightflow-prod-fargate-spot-ce" type = "MANAGED" state = "ENABLED" service_role = aws_iam_role.batch_service_role.arn compute_resources { type = "FARGATE_SPOT" max_vcpus = 16 subnets = data.aws_subnets.default.ids security_group_ids = [aws_security_group.default.id] } tags = { Project = "InsightFlow" } } 2. AWS Batch Job Queue The job queue prioritizes jobs and maps them to the compute environment: resource "aws_batch_job_queue" "job_queue" { name = "insightflow-prod-job-queue" state = "ENABLED" priority = 1 compute_environment_order { order = 1 compute_environment = aws_batch_compute_environment.fargate_spot_ce.arn } tags = { Project = "InsightFlow" } } 3. AWS Batch Job Definition The job definition specifies the container image, commands, and environment variables: resource "aws_batch_job_definition" "ingestion_job_def" { name = "insightflow-prod-ingestion-job-def" type = "container" platform_capabilities = ["FARGATE"] container_properties = jsonencode({ image = "864899839546.dkr.ecr.ap-southeast-2.amazonaws.com/insightflow-ingestion:latest" command = ["python", "main.py"] environment = [ { name = "TARGET_BUCKET", value = "insightflow-prod-raw-data" }, { name = "AWS_REGION", value = "ap-southeast-2" } ] resourceRequirements = [ { type = "VCPU", value = "1" }, { type = "MEMORY", value = "2048" } ] }) retry_strategy { attempts = 1 } timeout { attempt_duration_seconds = 3600 } tags = { Project = "InsightFlow" } } Step 2: Writing the Ingestion Script The ingestion script is a Python program that: Downloads datasets from public sources. Processes the data (e.g., renames columns, converts formats). Uploads the processed data to the raw S3 bucket. Here’s a simplified version of the script: import os import pandas as pd import boto3 from botocore.exceptions import ClientError # S3 Configuration TARGET_BUCKET = os.environ.get("TARGET_BUCKET") s3_client = boto3.client("s3") def upload_to_s3(data_bytes, bucket, s3_key): try: s3_client.put_object(Bucket=bucket, Key=s3_key, Body=data_bytes) print(f"Uploaded to s3://{bucket}/{s3_key}") except ClientError as e: print(f"Failed to upload: {e}") def process_and_upload(dataset_url, dataset_name): df = pd.read_parquet(dataset_url)
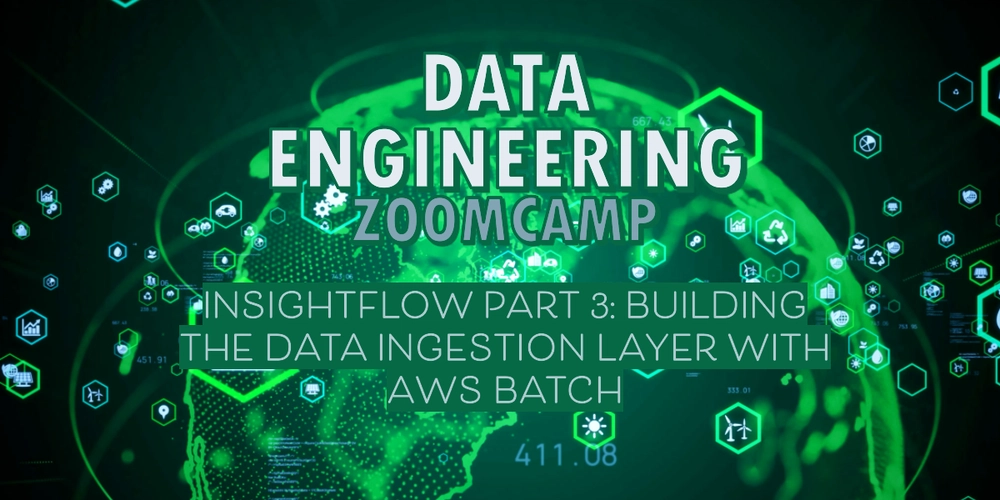
In this post, we’ll explore how the data ingestion layer for the InsightFlow project was built using AWS Batch. This layer is responsible for fetching raw data from public sources, processing it, and storing it in an S3 data lake for further transformation and analysis. By leveraging AWS Batch, we were able to create a scalable, cost-efficient, and fully managed solution for running containerized ingestion jobs.
Why AWS Batch?
AWS Batch is a fully managed service that enables you to run batch computing jobs at any scale. It dynamically provisions the optimal compute resources (e.g., Fargate, EC2) based on the volume and requirements of your jobs. For InsightFlow, AWS Batch was the perfect choice because:
- Scalability: Automatically scales resources to handle varying workloads.
- Cost Efficiency: Supports Fargate Spot instances for cost savings.
- Containerized Workflows: Runs containerized Python scripts for ingestion, ensuring consistency across environments.
- Integration with AWS Services: Natively integrates with S3, CloudWatch, and IAM for seamless data processing and monitoring.
Overview of the Ingestion Workflow
The ingestion layer is designed to:
-
Fetch Data: Download datasets from public APIs and direct download links (e.g.,
data.gov.my
). - Process Data: Perform lightweight transformations, such as renaming columns and converting formats.
- Store Data: Upload the processed data to an S3 bucket in a structured format (e.g., partitioned by year and month).
Key Components
- AWS Batch Job Definition: Defines the container image, resource requirements, and environment variables for the ingestion job.
- AWS Batch Job Queue: Manages the execution of jobs and maps them to compute environments.
- AWS Batch Compute Environment: Specifies the underlying compute resources (e.g., Fargate Spot).
- Python Ingestion Script: A containerized script that handles data fetching, processing, and uploading to S3.
Step 1: Setting Up the Infrastructure
The infrastructure for the ingestion layer was provisioned using Terraform. Below are the key resources and their configurations.
1. AWS Batch Compute Environment
The compute environment uses Fargate Spot for cost efficiency. Here’s the Terraform configuration:
resource "aws_batch_compute_environment" "fargate_spot_ce" {
compute_environment_name = "insightflow-prod-fargate-spot-ce"
type = "MANAGED"
state = "ENABLED"
service_role = aws_iam_role.batch_service_role.arn
compute_resources {
type = "FARGATE_SPOT"
max_vcpus = 16
subnets = data.aws_subnets.default.ids
security_group_ids = [aws_security_group.default.id]
}
tags = {
Project = "InsightFlow"
}
}
2. AWS Batch Job Queue
The job queue prioritizes jobs and maps them to the compute environment:
resource "aws_batch_job_queue" "job_queue" {
name = "insightflow-prod-job-queue"
state = "ENABLED"
priority = 1
compute_environment_order {
order = 1
compute_environment = aws_batch_compute_environment.fargate_spot_ce.arn
}
tags = {
Project = "InsightFlow"
}
}
3. AWS Batch Job Definition
The job definition specifies the container image, commands, and environment variables:
resource "aws_batch_job_definition" "ingestion_job_def" {
name = "insightflow-prod-ingestion-job-def"
type = "container"
platform_capabilities = ["FARGATE"]
container_properties = jsonencode({
image = "864899839546.dkr.ecr.ap-southeast-2.amazonaws.com/insightflow-ingestion:latest"
command = ["python", "main.py"]
environment = [
{ name = "TARGET_BUCKET", value = "insightflow-prod-raw-data" },
{ name = "AWS_REGION", value = "ap-southeast-2" }
]
resourceRequirements = [
{ type = "VCPU", value = "1" },
{ type = "MEMORY", value = "2048" }
]
})
retry_strategy {
attempts = 1
}
timeout {
attempt_duration_seconds = 3600
}
tags = {
Project = "InsightFlow"
}
}
Step 2: Writing the Ingestion Script
The ingestion script is a Python program that:
- Downloads datasets from public sources.
- Processes the data (e.g., renames columns, converts formats).
- Uploads the processed data to the raw S3 bucket.
Here’s a simplified version of the script:
import os
import pandas as pd
import boto3
from botocore.exceptions import ClientError
# S3 Configuration
TARGET_BUCKET = os.environ.get("TARGET_BUCKET")
s3_client = boto3.client("s3")
def upload_to_s3(data_bytes, bucket, s3_key):
try:
s3_client.put_object(Bucket=bucket, Key=s3_key, Body=data_bytes)
print(f"Uploaded to s3://{bucket}/{s3_key}")
except ClientError as e:
print(f"Failed to upload: {e}")
def process_and_upload(dataset_url, dataset_name):
df = pd.read_parquet(dataset_url)
s3_key = f"raw/{dataset_name}/data.parquet"
upload_to_s3(df.to_parquet(index=False), TARGET_BUCKET, s3_key)
if __name__ == "__main__":
process_and_upload(" ", "iowrt")
Step 3: Submitting the Job
The ingestion job is triggered using the AWS CLI or programmatically via the AWS SDK. Here’s an example of submitting the job using the CLI:
aws batch submit-job \\
--region ap-southeast-2 \\
--job-name "insightflow-ingestion-job" \\
--job-queue "insightflow-prod-job-queue" \\
--job-definition "insightflow-prod-ingestion-job-def"
Step 4: Monitoring and Logging
Logs from the ingestion job are sent to CloudWatch Logs for monitoring and debugging. The following configuration in the job definition enables logging:
logConfiguration = {
logDriver = "awslogs"
options = {
"awslogs-group" = "/aws/batch/job"
"awslogs-region" = "ap-southeast-2"
"awslogs-stream-prefix" = "batch"
}
}
You can view the logs in the CloudWatch Console under the /aws/batch/job
log group.
Challenges and Lessons Learned
- IAM Permissions: Ensuring the Batch job role had the necessary permissions to access S3 and CloudWatch was critical.
- Error Handling: Adding retries and timeouts in the job definition helped handle transient errors during data fetching.
- Cost Optimization: Using Fargate Spot instances significantly reduced costs without compromising performance.
Conclusion
By leveraging AWS Batch, we built a robust and scalable data ingestion layer for InsightFlow. This layer automates the process of fetching, processing, and storing raw data, laying the foundation for downstream transformations and analysis.