How Reinforcement Learning Powers Decision-Making in AI
Artificial intelligence requires autonomous machines to perform tasks through their ability to make decision outputs. Reinforcement learning stands as one of the most effective methods that enables this capability due to its work in machine learning, as agents learn decision sequences through environment interaction. Supervised learning relies on trained models using labeled data, but reinforcement learning deduces knowledge from the effects of agent actions without training on labeled information. This method replicates how human and animal species gain knowledge by experimenting. RL functions as a fundamental technique in the teaching process of optimal behavior for AI systems operating within complex environments through its applications in training autonomous machines and dynamic pricing systems along with gameplay bots. To explore advanced AI domains effectively, you should take a machine learning course in Canada to gain practical experience combined with theoretical knowledge that leads to success. What is reinforcement learning? Reinforcement Learning operates as a machine learning method through which agents develop their decision-making behaviors after executing actions and then receiving positive or negative feedback from their environments. The agent aims to discover a policy that provides optimal decisions for achieving maximum rewards throughout its operation. The main elements of RL comprise a learning entity called the agent, along with its system environment, move actions, environmental states, and feedback rewards. The feedback loop enables agents in RL to enhance their strategy through step-by-step improvement using algorithms that include Q-learning as well as Deep Q-Networks (DQN) and Proximal Policy Optimization (PPO). Real-World Applications of Reinforcement Learning Reinforcement learning enables technology solutions for multiple practical scenarios across different fields. Through autonomous driving, RL permits self-driving cars to handle roads alongside collision prevention and traffic condition adaptation by observing diverse driving environments through simulation. The application of RL in robotics grants machines the capability to execute elaborate procedures like component assembly and object handling, alongside autonomous exploration of unknown terrains without substantial human supervision. RL plays a crucial role in algorithmic trading by creating optimization strategies based on market analysis and past trading patterns to maximize financial returns. The healthcare industry uses RL to develop personalized treatments through strategy recommendations, which improve long-term patient results. Recommendation systems functioning on Netflix and YouTube platforms implement RL methodologies to generate real-time user preference learning for more personalized content presentations. Students who enroll in a machine learning course in Canada can apply their learning through real-world projects, alongside additional industry mentorship, and by using simulation tools. How Reinforcement Learning Enhances Decision-Making The fundamental process of decision-making requires picking the most suitable step under existing circumstances. RL provides several beneficial methods to improve the decision-making process. The agents in RL learn through repetitive attempts and subsequent adjustments to their actions based on the received feedback. The method becomes highly beneficial when operating in uncertain situations that obscure the correct decisions. In video game settings, loose rounds enable RL agents to gain effective strategies through the process of feedback. Secondly, RL supports long-term planning. The approach aims to optimize total rewards accumulated throughout a period, thus benefiting situations that require short-term disadvantages to secure superior long-term performance. How systems operate based on immediate feedback alone provides no equivalent benefit to this method. RL delivers effective control over the fundamental trade-off between examining new opportunities and maximizing the use of existing resources. Between retaining proven strategies and searching for new approaches exists a permanent conflict during decision-making. RL algorithms manage to combine innovative elements with reliable system design to deliver efficient functional solutions. Lastly, RL agents exhibit adaptability. Agents under this system make continuous environment adjustments while adapting strategy instructions from new information and practical experiences to preserve their operational value. AI systems depend heavily on this capability set for their modern functionality. Reinforcement learning expertise gained from an industry-aligned machine learning course in Canada gives professionals the ability to develop AI systems that learn to make independent decisions. Key Algorithms in Reinforcement Learning Reinforcement learning utilizes several important al
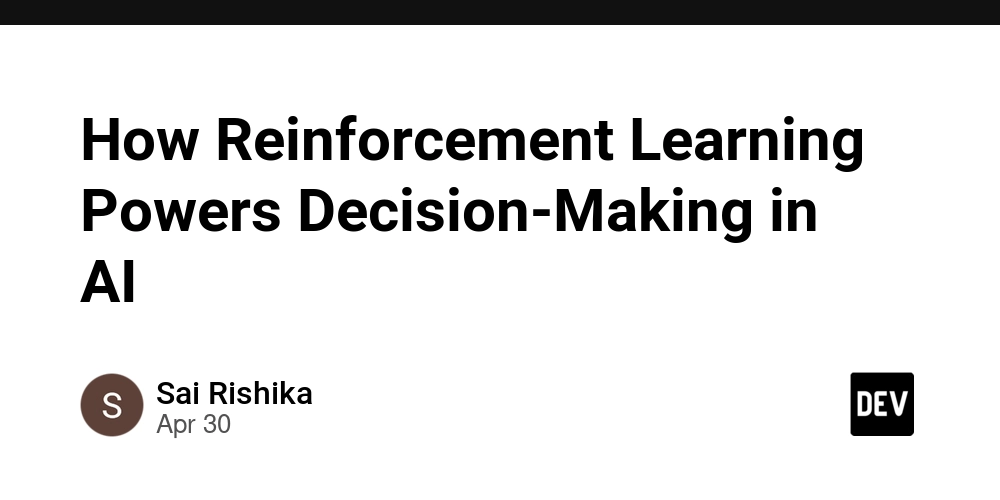
Artificial intelligence requires autonomous machines to perform tasks through their ability to make decision outputs. Reinforcement learning stands as one of the most effective methods that enables this capability due to its work in machine learning, as agents learn decision sequences through environment interaction. Supervised learning relies on trained models using labeled data, but reinforcement learning deduces knowledge from the effects of agent actions without training on labeled information. This method replicates how human and animal species gain knowledge by experimenting.
RL functions as a fundamental technique in the teaching process of optimal behavior for AI systems operating within complex environments through its applications in training autonomous machines and dynamic pricing systems along with gameplay bots. To explore advanced AI domains effectively, you should take a machine learning course in Canada to gain practical experience combined with theoretical knowledge that leads to success.
What is reinforcement learning?
Reinforcement Learning operates as a machine learning method through which agents develop their decision-making behaviors after executing actions and then receiving positive or negative feedback from their environments. The agent aims to discover a policy that provides optimal decisions for achieving maximum rewards throughout its operation.
The main elements of RL comprise a learning entity called the agent, along with its system environment, move actions, environmental states, and feedback rewards.
The feedback loop enables agents in RL to enhance their strategy through step-by-step improvement using algorithms that include Q-learning as well as Deep Q-Networks (DQN) and Proximal Policy Optimization (PPO).
Real-World Applications of Reinforcement Learning
Reinforcement learning enables technology solutions for multiple practical scenarios across different fields. Through autonomous driving, RL permits self-driving cars to handle roads alongside collision prevention and traffic condition adaptation by observing diverse driving environments through simulation. The application of RL in robotics grants machines the capability to execute elaborate procedures like component assembly and object handling, alongside autonomous exploration of unknown terrains without substantial human supervision.
RL plays a crucial role in algorithmic trading by creating optimization strategies based on market analysis and past trading patterns to maximize financial returns. The healthcare industry uses RL to develop personalized treatments through strategy recommendations, which improve long-term patient results. Recommendation systems functioning on Netflix and YouTube platforms implement RL methodologies to generate real-time user preference learning for more personalized content presentations.
Students who enroll in a machine learning course in Canada can apply their learning through real-world projects, alongside additional industry mentorship, and by using simulation tools.
How Reinforcement Learning Enhances Decision-Making
The fundamental process of decision-making requires picking the most suitable step under existing circumstances. RL provides several beneficial methods to improve the decision-making process.
The agents in RL learn through repetitive attempts and subsequent adjustments to their actions based on the received feedback. The method becomes highly beneficial when operating in uncertain situations that obscure the correct decisions. In video game settings, loose rounds enable RL agents to gain effective strategies through the process of feedback.
Secondly, RL supports long-term planning. The approach aims to optimize total rewards accumulated throughout a period, thus benefiting situations that require short-term disadvantages to secure superior long-term performance. How systems operate based on immediate feedback alone provides no equivalent benefit to this method.
RL delivers effective control over the fundamental trade-off between examining new opportunities and maximizing the use of existing resources. Between retaining proven strategies and searching for new approaches exists a permanent conflict during decision-making. RL algorithms manage to combine innovative elements with reliable system design to deliver efficient functional solutions.
Lastly, RL agents exhibit adaptability. Agents under this system make continuous environment adjustments while adapting strategy instructions from new information and practical experiences to preserve their operational value. AI systems depend heavily on this capability set for their modern functionality. Reinforcement learning expertise gained from an industry-aligned machine learning course in Canada gives professionals the ability to develop AI systems that learn to make independent decisions.
Key Algorithms in Reinforcement Learning
Reinforcement learning utilizes several important algorithms as part of its operation. Through its lack of algorithms, Q-learning allows agents to learn exactly how much benefit they will obtain when choosing actions from specific states. SARSA, as an algorithm, shares similarities with Q-learning, yet it adds the essential element of considering the active choices governed by existing policies.
The Deep Q-Network algorithm combines Q-learning methods with deep neural networks to handle control systems with extensive input dimensions. Policy gradient methods optimize the policy structure directly instead of using value function dependencies to guide their approach.
In-depth AI and ML courses in Canada focus on advanced RL topics through both classroom lectures and project-based assignments to strengthen students' understanding.
Challenges in Reinforcement Learning
Reinforcement learning offers numerous benefits to users, but it also creates challenges that agents need to overcome. RL faces a primary difficulty because it requires countless environmental interactions during learning, yet these demands lead to resource-intensive processes.
Another challenge is sparse rewards. The agent faces difficulties in determining which actions produce successful outcomes because meaningful feedback occurs very rarely in various environments. RL agents pose risks to safety and ethics because their solution techniques might meet goals through technically valid means, which breach confirmed protocols or violate ethical guidelines.
Lastly, generalization remains a hurdle. An agent that receives training in particular environmental conditions will not deliver good results when encountering situations that differ slightly from those trained conditions. High-quality AI and ML courses in Canada offer students access to computational resources, together with principles of reinforcement learning and real-world experience needed to overcome these challenges.
Why Learn Reinforcement Learning Today?
The technological world has recognized reinforcement learning as an absolute requirement for industry operations. The next-generation intelligent applications are being developed through the implementation of RL in systems created by Tesla and Google's AlphaGo. Understanding of reinforcement learning can lead to access to successful careers in autonomous systems, finance, robotics, and personalized healthcare sectors.
Canadian research institutions and a thriving technological ecosystem create a perfect environment for students who want to study reinforcement learning with machine learning. A machine learning course in Canada with a proper structure provides students with both theoretical knowledge and hands-on experience in implementing RL solutions to real-world problems.
Final Thoughts
The field of artificial intelligence decision-making currently reaches its highest level through reinforcement learning. This key AI system component enables learning through interactions, adapting over time, and delivers optimal long-term goals due to its ability to learn and adapt. The demand for professionals trained in RL will rise because industries are implementing growing numbers of intelligent automation and data-driven methods.
Choosing the appropriate education path becomes vital for all those who wish to become AI professionals. Students who choose a machine learning course in Canada gain access to premium educational facilities and top-notch teaching staff, as well as excellent employment prospects. Investing in top-tier AI and ML courses in Canada can provide you with the education needed to emerge as a leader in the advanced AI domain.