How do decision trees handle missing data values?
Decision trees are a popular machine learning algorithm known for their simplicity and interpretability. However, handling missing data values is an important challenge when building decision trees, as missing values can impact the model’s accuracy and decision-making process. There are several strategies that decision trees use to manage missing data: Surrogate Splits: One common approach is using surrogate splits. When the primary feature (used for a split) is missing for a record, the decision tree looks for another feature that closely mimics the behavior of the primary feature. This secondary feature acts as a substitute, allowing the record to continue its journey through the tree without interruption. Assigning to the Most Common Branch: Another method is assigning the record to the most frequent branch at the split. If a value is missing, the record follows the branch that the majority of records follow, based on training data distribution at that node. Probability-Based Assignment: In some implementations, records with missing values are divided across branches according to the probabilities observed in training data. For instance, if 70% of records go left and 30% go right at a certain node, a record with missing data is split accordingly in a weighted fashion. Preprocessing Missing Values: Before building the tree, missing values can be handled at the data preprocessing stage using imputation techniques such as filling with the mean, median, mode, or using more advanced methods like k-nearest neighbors (KNN) imputation. These strategies ensure that decision trees remain robust and effective even when data is incomplete. Proper handling of missing values leads to models that generalize better and maintain performance when faced with real-world, imperfect data. Understanding these concepts deeply is crucial for anyone pursuing a data science and machine learning course.
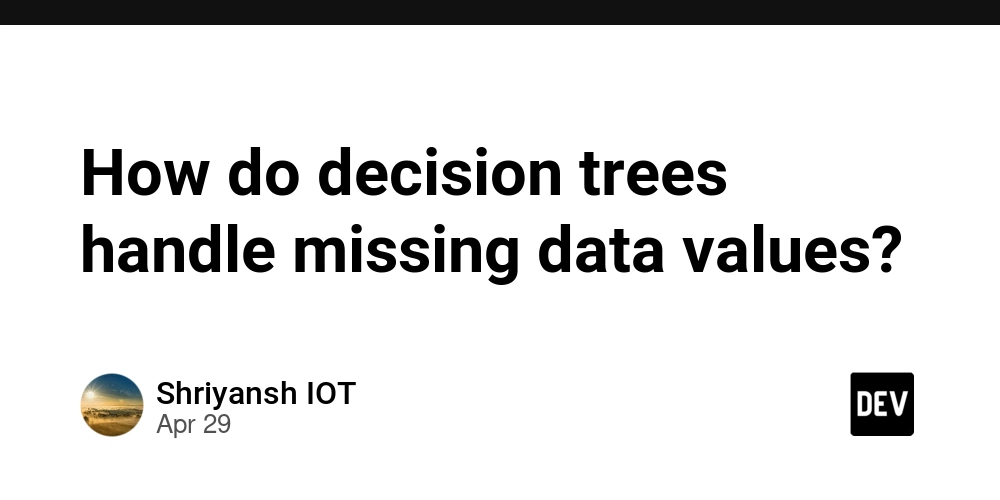
Decision trees are a popular machine learning algorithm known for their simplicity and interpretability. However, handling missing data values is an important challenge when building decision trees, as missing values can impact the model’s accuracy and decision-making process.
There are several strategies that decision trees use to manage missing data:
Surrogate Splits:
One common approach is using surrogate splits. When the primary feature (used for a split) is missing for a record, the decision tree looks for another feature that closely mimics the behavior of the primary feature. This secondary feature acts as a substitute, allowing the record to continue its journey through the tree without interruption.
Assigning to the Most Common Branch:
Another method is assigning the record to the most frequent branch at the split. If a value is missing, the record follows the branch that the majority of records follow, based on training data distribution at that node.
Probability-Based Assignment:
In some implementations, records with missing values are divided across branches according to the probabilities observed in training data. For instance, if 70% of records go left and 30% go right at a certain node, a record with missing data is split accordingly in a weighted fashion.
Preprocessing Missing Values:
Before building the tree, missing values can be handled at the data preprocessing stage using imputation techniques such as filling with the mean, median, mode, or using more advanced methods like k-nearest neighbors (KNN) imputation.
These strategies ensure that decision trees remain robust and effective even when data is incomplete. Proper handling of missing values leads to models that generalize better and maintain performance when faced with real-world, imperfect data.
Understanding these concepts deeply is crucial for anyone pursuing a data science and machine learning course.