What is MCP? A Simple Guide to AI’s Next Big Thing
Imagine a World Where AI Integrates Effortlessly Meet MCP (Model Context Protocol)—an open standard that might be the game-changer developers and innovators have been dreaming of. Pioneered by Anthropic’s bold vision, MCP tackles a core LLM limitation: They’re brilliant but hands-off. They’ll write you an essay but won’t email it, craft a database query but won’t hit “run.” MCP rewires that with context—unlocking a new level of AI capability. How MCP Works MCP’s power is in its context. It’s a framework built on four pillars: resources, tools, prompts, and sampling. Here’s the real scoop: Resources: Your files, databases, or live feeds—like GitHub repos or Slack channels. MCP servers tap these, giving AI a window into your world. Tools: The magic sauce—executable functions. Think “fix this code” or “post this update.” The better the tools, the stronger the MCP server. Quality here is make-or-break. Prompts: Smart, pre-set guides that keep AI on track—think of them as the AI’s cheat sheet. A server with sharp prompts means sharper results. Sampling: The wild card—leveraging multiple LLMs to run different tools, blending outputs for richer solutions. It’s like an AI brainstorming session. Why It’s a Big Deal MCP’s strength hinges on its servers. A top-tier server with ace tools and prompts turns AI into a doer, not just a thinker. A shaky one? You’re stuck with suggestions, not solutions. And here’s the kicker: It’s not just for big players like Cursor or Windsurf. The race is wide open—new tools will emerge, and you could build one. Picture This Your AI: ✅ Dives into your GitHub repo, spots a pesky bug in your code ✅ Fixes it using a tool ✅ Checks your Slack channel for past chatter about the issue ✅ Uses sampling across models to validate the best fix That’s MCP flexing its muscles. MCP vs. APIs
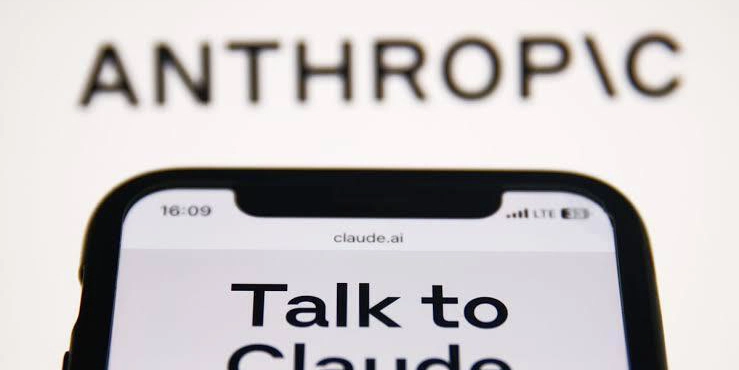
Imagine a World Where AI Integrates Effortlessly
Meet MCP (Model Context Protocol)—an open standard that might be the game-changer developers and innovators have been dreaming of.
Pioneered by Anthropic’s bold vision, MCP tackles a core LLM limitation: They’re brilliant but hands-off. They’ll write you an essay but won’t email it, craft a database query but won’t hit “run.” MCP rewires that with context—unlocking a new level of AI capability.
How MCP Works
MCP’s power is in its context. It’s a framework built on four pillars: resources, tools, prompts, and sampling. Here’s the real scoop:
- Resources: Your files, databases, or live feeds—like GitHub repos or Slack channels. MCP servers tap these, giving AI a window into your world.
- Tools: The magic sauce—executable functions. Think “fix this code” or “post this update.” The better the tools, the stronger the MCP server. Quality here is make-or-break.
- Prompts: Smart, pre-set guides that keep AI on track—think of them as the AI’s cheat sheet. A server with sharp prompts means sharper results.
- Sampling: The wild card—leveraging multiple LLMs to run different tools, blending outputs for richer solutions. It’s like an AI brainstorming session.
Why It’s a Big Deal
MCP’s strength hinges on its servers. A top-tier server with ace tools and prompts turns AI into a doer, not just a thinker. A shaky one? You’re stuck with suggestions, not solutions.
And here’s the kicker: It’s not just for big players like Cursor or Windsurf. The race is wide open—new tools will emerge, and you could build one.
Picture This
Your AI:
✅ Dives into your GitHub repo, spots a pesky bug in your code
✅ Fixes it using a tool
✅ Checks your Slack channel for past chatter about the issue
✅ Uses sampling across models to validate the best fix
That’s MCP flexing its muscles.
MCP vs. APIs