InsightFlow Part 9: Workflow Orchestration with Kestra
9. Workflow Orchestration with Kestra In modern data engineering, orchestrating workflows is a critical component of building reliable, scalable, and automated data pipelines. For the InsightFlow project, we leverage Kestra, an open-source declarative orchestration platform, to manage the end-to-end workflow of ingesting, transforming, and analyzing retail and economic data from public sources. This blog post will walk you through how Kestra is used in this project and why it is an excellent choice for workflow orchestration. Why Kestra? Kestra is a modern orchestration platform designed to simplify the management of complex workflows. It offers several features that make it ideal for the InsightFlow project: Declarative Workflow Design: Workflows are defined in YAML, making them easy to read, version-control, and maintain. Scalability: Kestra can handle large-scale workflows with hundreds of tasks, ensuring reliability even under heavy loads. Extensibility: With over 600 plugins, Kestra supports a wide range of tasks, including AWS services, database queries, and custom scripts. Observability: Kestra provides detailed logs, metrics, and monitoring tools to track workflow execution and troubleshoot issues. Integration with Modern Tools: Kestra integrates seamlessly with Git, Terraform, and other tools, enabling a streamlined CI/CD pipeline. Kestra in the InsightFlow Project In the InsightFlow project, Kestra orchestrates the following key workflows: Data Ingestion: Fetching raw data from public sources using AWS Batch. Data Transformation: Running dbt models to clean, normalize, and structure the data. Data Cataloging: Updating the AWS Glue Data Catalog to reflect the latest data. Testing and Validation: Running dbt tests to ensure data quality. Scheduling and Automation: Automating the entire pipeline to run on a daily schedule. Workflow Overview The Kestra workflow for the production environment is defined in the file insightflow_prod_pipeline.yml. Below is an overview of the key tasks: 1. Data Ingestion via AWS Batch The workflow starts by submitting an AWS Batch job to ingest raw data from public sources into the S3 bucket insightflow-prod-raw-data. This is achieved using the following task: - id: submit_batch_ingestion_job_cli type: io.kestra.core.tasks.scripts.Bash commands: - | echo "Submitting AWS Batch Job..." JOB_DEF_NAME="insightflow-prod-ingestion-job-def" JOB_QUEUE_NAME="insightflow-prod-job-queue" TARGET_BUCKET_NAME="insightflow-prod-raw-data" AWS_REGION="ap-southeast-2" JOB_NAME="insightflow-ingestion-{{execution.id}}" JOB_OUTPUT=$(aws batch submit-job \\ --region "$AWS_REGION" \\ --job-name "$JOB_NAME" \\ --job-queue "$JOB_QUEUE_NAME" \\ --job-definition "$JOB_DEF_NAME" \\ --container-overrides '{ "environment": [ {"name": "TARGET_BUCKET", "value": "'"$TARGET_BUCKET_NAME"'"} ] }') JOB_ID=$(echo "$JOB_OUTPUT" | grep -o '"jobId": "[^"]*' | awk -F'"' '{print $4}') echo "Submitted Job ID: $JOB_ID" 2. Updating the Glue Data Catalog Once the raw data is ingested, the workflow triggers an AWS Glue Crawler to update the Glue Data Catalog. This ensures that the latest data is available for querying in Athena. - id: start_glue_crawler_cli type: io.kestra.core.tasks.scripts.Bash commands: - | echo "Starting AWS Glue Crawler..." CRAWLER_NAME="insightflow-prod-raw-data-crawler" AWS_REGION="ap-southeast-2" aws glue start-crawler --region $AWS_REGION --name "$CRAWLER_NAME" echo "Crawler $CRAWLER_NAME started." 3. Running dbt Models After the data is cataloged, the workflow runs dbt models to transform the raw data into an analysis-ready format. This includes tasks for syncing dbt files, installing dependencies, and running the models. - id: dbt_run type: io.kestra.plugin.dbt.cli.DbtCLI commands: - dbt run --target prod namespaceFiles: enabled: false containerImage: pizofreude/kestra-dbt-athena:latest 4. Testing and Validation To ensure data quality, the workflow runs dbt tests on the transformed data. Any issues are logged for further investigation. - id: dbt_test type: io.kestra.plugin.dbt.cli.DbtCLI commands: - dbt test --target prod namespaceFiles: enabled: false containerImage: pizofreude/kestra-dbt-athena:latest 5. Scheduling The workflow is scheduled to run daily at 5:00 AM UTC using Kestra's scheduling feature. triggers: - id: daily_schedule type: io.kestra.plugin.core.trigger.Schedule cron: "0 5 * * *" Benefits of Using Kestra Automation: Kestra automates the entire pipeline, reducing manual intervention and ensuring consistency. Error Handling: With built-in retry me
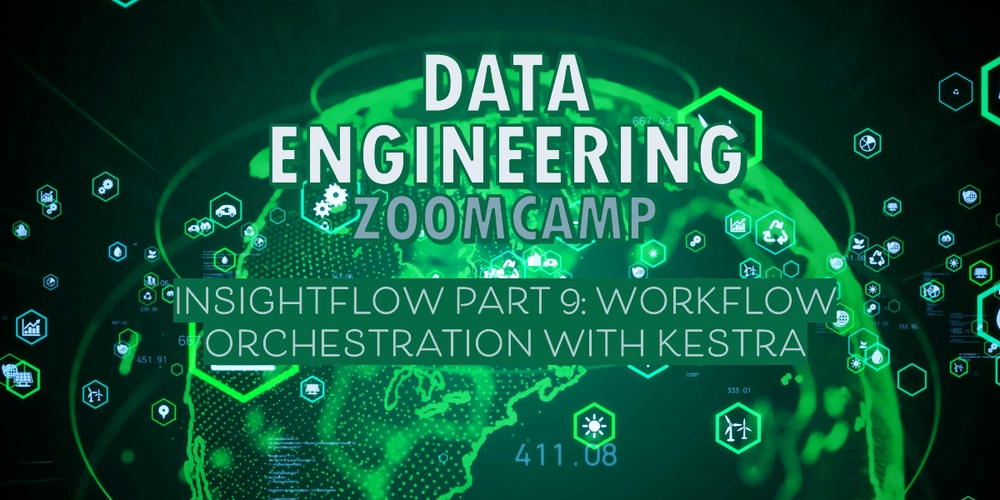
9. Workflow Orchestration with Kestra
In modern data engineering, orchestrating workflows is a critical component of building reliable, scalable, and automated data pipelines. For the InsightFlow project, we leverage Kestra, an open-source declarative orchestration platform, to manage the end-to-end workflow of ingesting, transforming, and analyzing retail and economic data from public sources. This blog post will walk you through how Kestra is used in this project and why it is an excellent choice for workflow orchestration.
Why Kestra?
Kestra is a modern orchestration platform designed to simplify the management of complex workflows. It offers several features that make it ideal for the InsightFlow project:
- Declarative Workflow Design: Workflows are defined in YAML, making them easy to read, version-control, and maintain.
- Scalability: Kestra can handle large-scale workflows with hundreds of tasks, ensuring reliability even under heavy loads.
- Extensibility: With over 600 plugins, Kestra supports a wide range of tasks, including AWS services, database queries, and custom scripts.
- Observability: Kestra provides detailed logs, metrics, and monitoring tools to track workflow execution and troubleshoot issues.
- Integration with Modern Tools: Kestra integrates seamlessly with Git, Terraform, and other tools, enabling a streamlined CI/CD pipeline.
Kestra in the InsightFlow Project
In the InsightFlow project, Kestra orchestrates the following key workflows:
- Data Ingestion: Fetching raw data from public sources using AWS Batch.
- Data Transformation: Running dbt models to clean, normalize, and structure the data.
- Data Cataloging: Updating the AWS Glue Data Catalog to reflect the latest data.
- Testing and Validation: Running dbt tests to ensure data quality.
- Scheduling and Automation: Automating the entire pipeline to run on a daily schedule.
Workflow Overview
The Kestra workflow for the production environment is defined in the file insightflow_prod_pipeline.yml
. Below is an overview of the key tasks:
1. Data Ingestion via AWS Batch
The workflow starts by submitting an AWS Batch job to ingest raw data from public sources into the S3 bucket insightflow-prod-raw-data
. This is achieved using the following task:
- id: submit_batch_ingestion_job_cli
type: io.kestra.core.tasks.scripts.Bash
commands:
- |
echo "Submitting AWS Batch Job..."
JOB_DEF_NAME="insightflow-prod-ingestion-job-def"
JOB_QUEUE_NAME="insightflow-prod-job-queue"
TARGET_BUCKET_NAME="insightflow-prod-raw-data"
AWS_REGION="ap-southeast-2"
JOB_NAME="insightflow-ingestion-{{execution.id}}"
JOB_OUTPUT=$(aws batch submit-job \\
--region "$AWS_REGION" \\
--job-name "$JOB_NAME" \\
--job-queue "$JOB_QUEUE_NAME" \\
--job-definition "$JOB_DEF_NAME" \\
--container-overrides '{
"environment": [
{"name": "TARGET_BUCKET", "value": "'"$TARGET_BUCKET_NAME"'"}
]
}')
JOB_ID=$(echo "$JOB_OUTPUT" | grep -o '"jobId": "[^"]*' | awk -F'"' '{print $4}')
echo "Submitted Job ID: $JOB_ID"
2. Updating the Glue Data Catalog
Once the raw data is ingested, the workflow triggers an AWS Glue Crawler to update the Glue Data Catalog. This ensures that the latest data is available for querying in Athena.
- id: start_glue_crawler_cli
type: io.kestra.core.tasks.scripts.Bash
commands:
- |
echo "Starting AWS Glue Crawler..."
CRAWLER_NAME="insightflow-prod-raw-data-crawler"
AWS_REGION="ap-southeast-2"
aws glue start-crawler --region $AWS_REGION --name "$CRAWLER_NAME"
echo "Crawler $CRAWLER_NAME started."
3. Running dbt Models
After the data is cataloged, the workflow runs dbt models to transform the raw data into an analysis-ready format. This includes tasks for syncing dbt files, installing dependencies, and running the models.
- id: dbt_run
type: io.kestra.plugin.dbt.cli.DbtCLI
commands:
- dbt run --target prod
namespaceFiles:
enabled: false
containerImage: pizofreude/kestra-dbt-athena:latest
4. Testing and Validation
To ensure data quality, the workflow runs dbt tests on the transformed data. Any issues are logged for further investigation.
- id: dbt_test
type: io.kestra.plugin.dbt.cli.DbtCLI
commands:
- dbt test --target prod
namespaceFiles:
enabled: false
containerImage: pizofreude/kestra-dbt-athena:latest
5. Scheduling
The workflow is scheduled to run daily at 5:00 AM UTC using Kestra's scheduling feature.
triggers:
- id: daily_schedule
type: io.kestra.plugin.core.trigger.Schedule
cron: "0 5 * * *"
Benefits of Using Kestra
- Automation: Kestra automates the entire pipeline, reducing manual intervention and ensuring consistency.
- Error Handling: With built-in retry mechanisms and detailed logs, Kestra makes it easy to identify and resolve issues.
- Scalability: Kestra can handle large-scale workflows with multiple tasks and dependencies.
- Flexibility: The declarative YAML syntax allows for easy customization and extension of workflows.
Getting Started with Kestra
To set up Kestra for your own projects, follow these steps:
- Install Kestra: Refer to the Kestra documentation for installation instructions.
- Define Workflows: Create YAML files to define your workflows, as shown in the examples above.
- Run Workflows: Use the Kestra UI or CLI to execute and monitor your workflows.
- Integrate with CI/CD: Use Git and Terraform to version-control and deploy your workflows.
Conclusion
Kestra is a powerful tool for orchestrating workflows in modern data pipelines. In the InsightFlow project, it plays a crucial role in automating the ingestion, transformation, and validation of retail and economic data. By leveraging Kestra's features, we ensure that the pipeline is reliable, scalable, and easy to maintain.
If you're building a similar project, consider using Kestra to simplify your workflow orchestration. For more details, check out the Kestra documentation or explore the InsightFlow repository.
Happy orchestrating!