How I Tamed a Wild AI to Answer Banking Questions Without Making Things Up: A Spring Boot + OpenAI Story
Banking info should be easier to find than car keys on a Monday morning. Sadly, it isn’t. So we built a chatbot to fix that. A behind-the-scenes look at designing an AI-driven chatbot for the future of banking — And My first encounter with Open AI. Introduction: The Spark Behind the Idea In today’s digital-first world, banks and financial institutions face an ongoing challenge: how to provide quick, reliable, and accessible information to both customers and employees. Whether it’s finding the nearest branch, understanding service policies, or accessing executive contacts — information is often buried across multiple systems, leading to inefficiencies. While working as a Senior Full Stack Developer specializing in innovation projects, I set out to tackle this challenge. My mission was clear: build a smart, intuitive banking chatbot powered by AI — a solution that could instantly answer banking queries using the capabilities of Azure OpenAI Service and the robustness of a Spring Boot backend. Why Azure OpenAI + Spring Boot? While OpenAI’s GPT models have demonstrated powerful natural language capabilities, enterprise financial services demand stricter compliance, security, and privacy standards. This made Azure OpenAI a natural choice — offering both powerful models and enterprise-grade security. For backend development, Spring Boot offered the flexibility and reliability needed to create a secure API service layer around the AI model. Solution Overview Here’s the high-level system architecture of the solution: How the Solution Works Users interact with the chatbot via a simple web UI or mobile app. They authenticate via OAuth2 or Single Sign-On (SSO). The query passes through the Chatbot API built using Spring Boot. The API securely communicates with Azure OpenAI’s fine-tuned endpoint. Responses are generated based on curated internal banking knowledge. The answer is sent back to the user — fast, accurate, and secure. Implementation Journey: Step-by-Step Step 1: Curating the Data The first step was to build a high-quality, domain-specific dataset. Collected internal documentation: branch locations, services, executives, operational policies. Structured the information into Q&A pairs for fine-tuning. Ensured that sensitive customer data was excluded. Step 2: Fine-tuning the Azure OpenAI Model Azure allowed for secure fine-tuning of GPT-3 with financial service-specific data: Uploaded the curated dataset. Tuned parameters (e.g., temperature, frequency penalty) for better factual reliability. Deployed the fine-tuned model under private, secure access environments. Step 3: Building the Spring Boot Backend To securely interface with Azure OpenAI: Created a Spring Boot REST API. Implemented user authentication middleware. Built secure, scalable APIs to handle communication with Azure services. Applied rate limiting and exception handling for better fault tolerance. Step 4: Deployment on Cloud Infrastructure To ensure scalability and compliance: Deployed the backend service in a cloud-native environment (using Kubernetes services like AWS EKS). Applied API Gateway security layers. Monitored performance with centralized logging and alerting systems. Key Challenges Faced: Challenge 1: Data Security Compliance Building an AI for banking is like inviting a dragon to dinner — cool, but you need a lot of safety rules. Financial institutions demand tight compliance standards. Solution: All data flows were secured within virtual private clouds (VPCs) and encrypted in transit. Challenge 2: Managing Hallucinations in AI Large language models sometimes “hallucinate” facts. Solution: Continuous feedback loops and retraining were established to improve accuracy over time. Challenge 3: API Latency Optimization External API calls to Azure AI introduced slight latencies. Solution: We optimized critical paths by implementing query caching for frequent questions. Impact: What Changed? Response time for internal banking queries improved dramatically. Operational efficiency increased, freeing up staff from manual lookups. Provided a foundation for customer-facing AI-driven banking initiatives. Demonstrated that enterprise-grade AI solutions can be securely and responsibly implemented in regulated industries. Conclusion: What I Learned Turns out, AI can answer your branch location faster than your colleague in the next cubicle. If AI can book your travel, recommend your next song, and now answer your banking questions — maybe it’s time we stop fearing it will steal our lunch. One chatbot at a time, we’re making banking conversations a little smarter — and a lot more human. Building an AI-powered chatbot was not just about leveraging APIs — it was about building trust. Trust that the system would deliver accurate answers, keep information secure, and be reliably available when needed. This project strengthened my expertise in: AI integration into real-world systems. Cloud-native API development. Enterprise AI complian
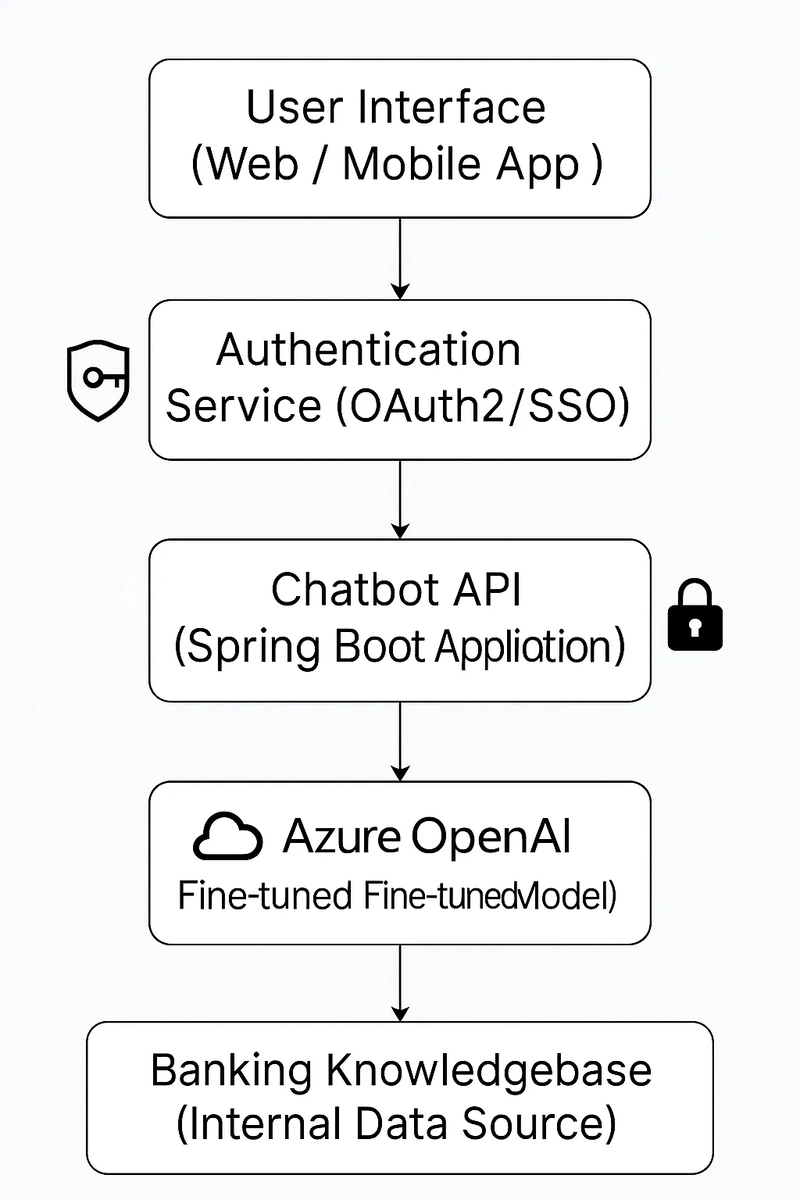
Banking info should be easier to find than car keys on a Monday morning. Sadly, it isn’t. So we built a chatbot to fix that. A behind-the-scenes look at designing an AI-driven chatbot for the future of banking — And My first encounter with Open AI.
Introduction: The Spark Behind the Idea
In today’s digital-first world, banks and financial institutions face an ongoing challenge: how to provide quick, reliable, and accessible information to both customers and employees.
Whether it’s finding the nearest branch, understanding service policies, or accessing executive contacts — information is often buried across multiple systems, leading to inefficiencies.
While working as a Senior Full Stack Developer specializing in innovation projects, I set out to tackle this challenge.
My mission was clear: build a smart, intuitive banking chatbot powered by AI — a solution that could instantly answer banking queries using the capabilities of Azure OpenAI Service and the robustness of a Spring Boot backend.
Why Azure OpenAI + Spring Boot?
While OpenAI’s GPT models have demonstrated powerful natural language capabilities, enterprise financial services demand stricter compliance, security, and privacy standards.
This made Azure OpenAI a natural choice — offering both powerful models and enterprise-grade security.
For backend development, Spring Boot offered the flexibility and reliability needed to create a secure API service layer around the AI model.
Solution Overview
Here’s the high-level system architecture of the solution:
How the Solution Works
Users interact with the chatbot via a simple web UI or mobile app.
They authenticate via OAuth2 or Single Sign-On (SSO).
The query passes through the Chatbot API built using Spring Boot.
The API securely communicates with Azure OpenAI’s fine-tuned endpoint.
Responses are generated based on curated internal banking knowledge.
The answer is sent back to the user — fast, accurate, and secure.
Implementation Journey: Step-by-Step
Step 1: Curating the Data
The first step was to build a high-quality, domain-specific dataset.
Collected internal documentation: branch locations, services, executives, operational policies.
Structured the information into Q&A pairs for fine-tuning.
Ensured that sensitive customer data was excluded.
Step 2: Fine-tuning the Azure OpenAI Model
Azure allowed for secure fine-tuning of GPT-3 with financial service-specific data:
Uploaded the curated dataset.
Tuned parameters (e.g., temperature, frequency penalty) for better factual reliability.
Deployed the fine-tuned model under private, secure access environments.
Step 3: Building the Spring Boot Backend
To securely interface with Azure OpenAI:
Created a Spring Boot REST API.
Implemented user authentication middleware.
Built secure, scalable APIs to handle communication with Azure services.
Applied rate limiting and exception handling for better fault tolerance.
Step 4: Deployment on Cloud Infrastructure
To ensure scalability and compliance:
Deployed the backend service in a cloud-native environment (using Kubernetes services like AWS EKS).
Applied API Gateway security layers.
Monitored performance with centralized logging and alerting systems.
Key Challenges Faced:
Challenge 1: Data Security Compliance
Building an AI for banking is like inviting a dragon to dinner — cool, but you need a lot of safety rules. Financial institutions demand tight compliance standards.
Solution: All data flows were secured within virtual private clouds (VPCs) and encrypted in transit.
Challenge 2: Managing Hallucinations in AI
Large language models sometimes “hallucinate” facts.
Solution: Continuous feedback loops and retraining were established to improve accuracy over time.
Challenge 3: API Latency Optimization
External API calls to Azure AI introduced slight latencies.
Solution: We optimized critical paths by implementing query caching for frequent questions.
Impact: What Changed?
Response time for internal banking queries improved dramatically.
Operational efficiency increased, freeing up staff from manual lookups.
Provided a foundation for customer-facing AI-driven banking initiatives.
Demonstrated that enterprise-grade AI solutions can be securely and responsibly implemented in regulated industries.
Conclusion: What I Learned
Turns out, AI can answer your branch location faster than your colleague in the next cubicle. If AI can book your travel, recommend your next song, and now answer your banking questions — maybe it’s time we stop fearing it will steal our lunch. One chatbot at a time, we’re making banking conversations a little smarter — and a lot more human.
Building an AI-powered chatbot was not just about leveraging APIs — it was about building trust.
Trust that the system would deliver accurate answers, keep information secure, and be reliably available when needed.
This project strengthened my expertise in:
AI integration into real-world systems.
Cloud-native API development.
Enterprise AI compliance and security.
It reaffirmed my belief that true innovation happens at the intersection of cutting-edge technology and real-world business challenges.
And the journey is just beginning.
Final Note
Are you exploring how AI can transform banking or enterprise systems?
Let’s connect — I’m always excited to exchange ideas and build the future together!