Finding and Remediating Idle AWS Databases Made Easy
It’s easy to overlook idle databases in AWS. Spun up in a hurry for testing, migration, or “just in case” scenarios, they often outlive their purpose and quietly accumulate charges in the background. This isn’t just about wasted money—though that’s a major factor. It’s also about infrastructure sprawl, increased attack surface, and the risk of unintentionally deleting something still critical because no one remembers what it was for. Over time, this clutter adds friction to your operations. Auditing becomes harder, deployment pipelines get noisier, and cost forecasting gets skewed by ghost workloads. What is an Idle AWS Database? An idle AWS database is any database instance—RDS, Aurora, Redshift, or others—that shows little to no meaningful engagement over time. That means no active connections, low or flatline read/write IOPS, and negligible CPU usage. It's not about a single moment of quiet—it's about a sustained pattern of inactivity confirmed by metrics and usage history. These instances often originate from valid use cases. A developer might create a new RDS instance for performance testing and forget to decommission it. A data team might launch a Redshift cluster for a one-time warehouse import. Or a migration project might leave behind a “just in case” snapshot that never gets used. What begins as a useful resource turns into a silent cost center. Idle doesn’t always mean harmless. These forgotten databases consume compute and storage; they also carry operational and security risks. When left unmonitored, they miss patches, drift from configuration baselines, and become more vulnerable to misconfigurations or open endpoints. Multiply that across regions, environments, or accounts, and the scale of the problem grows fast. The key characteristics of an idle instance usually follow a clear trend: Zero or near-zero active connections: Measured through CloudWatch’s DatabaseConnections metric across a 7–31 day span. Minimal IOPS activity: ReadIOPS and WriteIOPS metrics show little to no disk activity, often under 20 operations per day. Consistently low CPU usage: Less than 5% average CPU utilization over time is a strong indicator of inactivity—especially for provisioned instances, where you're paying for compute regardless. What makes these databases tricky is that they don’t always show up when scanning for errors or failures. They’re not broken. They're just not doing anything. Unless you're specifically looking for them, they simply blend into the noise. That’s why a structured approach to AWS idle database management, like the one used at Tom Granot, helps teams identify and clean up these costly, hidden inefficiencies without risking disruption. Why Do Idle Databases Matter? The biggest offender here is cost. Idle RDS instances, for example, continue charging for compute, provisioned IOPS, and storage—even if the database hasn't handled a single query in weeks. A multi-AZ SQL Server Enterprise instance left running for 90 days can quietly rack up over $10,000 in charges. These aren’t rare edge cases—they’re the result of default behaviors like forgetting to disable instances after a migration, or leaving replica clusters online “just in case.” Beyond cost, idle databases introduce unnecessary decision friction across engineering teams. During remediation or infrastructure reviews, teams get stuck triaging long lists of resources no one remembers provisioning. Old project databases hang around, cluttering dashboards and inflating usage graphs. CI/CD pipelines that spin up temporary databases often forget to tear them down, adding even more noise. This mess slows down operations, introduces confusion in ownership, and makes enforcing resource tagging standards harder. Attack surface is another issue. Idle doesn’t mean isolated—many of these databases still have active endpoints, permissive security groups, or unused IAM roles attached. Without owners watching them, they miss routine hardening: no patching, no version upgrades, and often no alarms. That’s how they become convenient entry points for attackers or compliance violations waiting to happen. In one real-world audit, a previously decommissioned staging database was discovered still accepting external connections—with default credentials. Common Types of Idle AWS Databases Idle databases tend to follow predictable origin stories. Most of them weren’t mistakes at the time—they were part of legitimate workflows that just never had a proper exit. Understanding how they get there is key to designing reliable cleanup processes. Short-Lived Environments That Overstay Temporary environments are a known source of idle sprawl, but the root issue often lies in automation gaps. CI/CD pipelines may provision new RDS instances for integration tests or staging deployments, yet fail to include teardown logic on failure paths or timeouts. These instances don’t just persist—they accumulate silentl
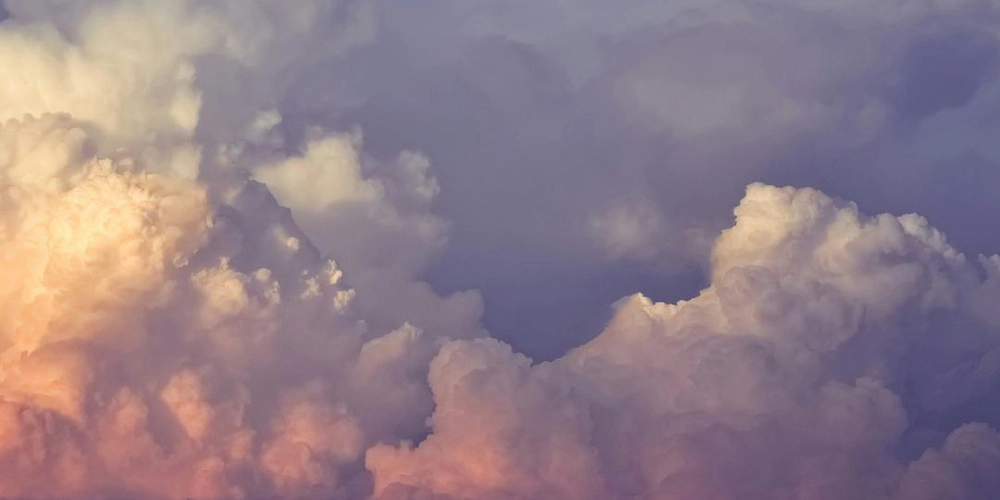
It’s easy to overlook idle databases in AWS. Spun up in a hurry for testing, migration, or “just in case” scenarios, they often outlive their purpose and quietly accumulate charges in the background.
This isn’t just about wasted money—though that’s a major factor. It’s also about infrastructure sprawl, increased attack surface, and the risk of unintentionally deleting something still critical because no one remembers what it was for.
Over time, this clutter adds friction to your operations. Auditing becomes harder, deployment pipelines get noisier, and cost forecasting gets skewed by ghost workloads.
What is an Idle AWS Database?
An idle AWS database is any database instance—RDS, Aurora, Redshift, or others—that shows little to no meaningful engagement over time. That means no active connections, low or flatline read/write IOPS, and negligible CPU usage. It's not about a single moment of quiet—it's about a sustained pattern of inactivity confirmed by metrics and usage history.
These instances often originate from valid use cases. A developer might create a new RDS instance for performance testing and forget to decommission it. A data team might launch a Redshift cluster for a one-time warehouse import. Or a migration project might leave behind a “just in case” snapshot that never gets used. What begins as a useful resource turns into a silent cost center.
Idle doesn’t always mean harmless. These forgotten databases consume compute and storage; they also carry operational and security risks. When left unmonitored, they miss patches, drift from configuration baselines, and become more vulnerable to misconfigurations or open endpoints. Multiply that across regions, environments, or accounts, and the scale of the problem grows fast.
The key characteristics of an idle instance usually follow a clear trend:
-
Zero or near-zero active connections: Measured through CloudWatch’s
DatabaseConnections
metric across a 7–31 day span. -
Minimal IOPS activity:
ReadIOPS
andWriteIOPS
metrics show little to no disk activity, often under 20 operations per day. - Consistently low CPU usage: Less than 5% average CPU utilization over time is a strong indicator of inactivity—especially for provisioned instances, where you're paying for compute regardless.
What makes these databases tricky is that they don’t always show up when scanning for errors or failures. They’re not broken. They're just not doing anything. Unless you're specifically looking for them, they simply blend into the noise. That’s why a structured approach to AWS idle database management, like the one used at Tom Granot, helps teams identify and clean up these costly, hidden inefficiencies without risking disruption.
Why Do Idle Databases Matter?
The biggest offender here is cost. Idle RDS instances, for example, continue charging for compute, provisioned IOPS, and storage—even if the database hasn't handled a single query in weeks. A multi-AZ SQL Server Enterprise instance left running for 90 days can quietly rack up over $10,000 in charges. These aren’t rare edge cases—they’re the result of default behaviors like forgetting to disable instances after a migration, or leaving replica clusters online “just in case.”
Beyond cost, idle databases introduce unnecessary decision friction across engineering teams. During remediation or infrastructure reviews, teams get stuck triaging long lists of resources no one remembers provisioning. Old project databases hang around, cluttering dashboards and inflating usage graphs. CI/CD pipelines that spin up temporary databases often forget to tear them down, adding even more noise. This mess slows down operations, introduces confusion in ownership, and makes enforcing resource tagging standards harder.
Attack surface is another issue. Idle doesn’t mean isolated—many of these databases still have active endpoints, permissive security groups, or unused IAM roles attached. Without owners watching them, they miss routine hardening: no patching, no version upgrades, and often no alarms. That’s how they become convenient entry points for attackers or compliance violations waiting to happen. In one real-world audit, a previously decommissioned staging database was discovered still accepting external connections—with default credentials.
Common Types of Idle AWS Databases
Idle databases tend to follow predictable origin stories. Most of them weren’t mistakes at the time—they were part of legitimate workflows that just never had a proper exit. Understanding how they get there is key to designing reliable cleanup processes.
Short-Lived Environments That Overstay
Temporary environments are a known source of idle sprawl, but the root issue often lies in automation gaps. CI/CD pipelines may provision new RDS instances for integration tests or staging deployments, yet fail to include teardown logic on failure paths or timeouts. These instances don’t just persist—they accumulate silently across accounts and regions, especially when the automation lacks tagging or TTL enforcement.
Another overlooked source comes from internal training or onboarding exercises. Teams spin up realistic environments to teach new hires or simulate customer setups, but unless someone owns the cleanup step, those environments become permanent residents. These databases often look like legitimate workloads—especially if they mimic production naming conventions—and are rarely flagged until a cost spike triggers a deeper review.
Post-Migration Leftovers and Dormant Archives
Infrastructure migrations tend to leave echoes: clusters, read replicas, or legacy endpoints that were supposed to be temporary but got lost in the shuffle. Often, the switch to a new instance happens cleanly, but the old one remains available out of habit or as a fallback that no one wants to touch. These drift further into obscurity when they’re excluded from IaC or drift detection tooling, making them invisible to standard audits.
Then there are the archival workloads—datasets that serve compliance or audit use cases but are no longer part of day-to-day operations. These aren't abandoned, but they’re grossly overprovisioned. For example, an RDS instance storing quarterly financial logs might idle at under 1% CPU for months, yet still runs on a db.m5.2xlarge
tier. Without scheduled shutdowns or a shift to more storage-efficient models, they quietly burn through budget while doing almost nothing.
Where Do Idle Databases Usually Live?
Idle databases tend to hide in the edges of your infrastructure—the places no one checks unless something breaks. Non-production environments lead the pack: staging, QA, sandbox accounts. These environments exist to mirror production closely enough for testing, but they often evolve organically without consistent controls. Feature branches, parallel testing workflows, or hotfix validation setups leave behind full-stack environments that no longer map to active code. Over time, these “temporary” databases blend into the noise because no one knows if they’re still important—or who to ask.
Automated systems, ironically, are another source of debris. Infrastructure-as-code tools, scheduled builds, or ephemeral environments generated on the fly often lack teardown hooks that execute reliably in every failure path. Over time, you’ll find RDS or Redshift instances created for one-off data loads, dry runs, or schema validation tests that never completed—but the instance stuck around. These workloads are rarely tagged with consistent metadata, and even when they are, they’re often excluded from cleanup policies due to lack of ownership mapping.
Multi-region activity introduces another layer of complexity. Organizations experimenting with latency optimization, geo-redundancy, or cross-border compliance often deploy workloads in secondary or tertiary regions. These databases serve a purpose for a few days—but months later, they’re still online in regions like me-south-1
or ap-northeast-3
, quietly accruing storage and networking costs. Since most billing dashboards default to primary regions, and many teams work within isolated scopes, these idle workloads often escape detection until someone performs a CUR deep dive or a cross-region resource inventory.
Then there’s the forgotten legacy tier—databases linked to deprecated services or abandoned proof-of-concepts. These are rarely part of modern CI/CD or infrastructure automation. Nobody’s watching the metrics, no alerts are configured, and no one has logged in for months. They’re still online because they’ve become “too old to touch”—deleting them feels risky without documentation, and the original owner probably left the organization or moved on to a different team. So they sit, draining budget and widening your security perimeter.
How to Find and Safely Remediate Idle Databases on AWS
Identifying idle databases starts with surfacing underutilized infrastructure across your AWS footprint. Use the Cost and Usage Report (CUR) to isolate long-running database instances with consistent spend. From there, pull runtime metrics like CPUUtilization
, FreeableMemory
, and NetworkThroughput
via CloudWatch to detect usage anomalies or consistent inactivity patterns over time. These aren't just raw stats—they form the behavioral fingerprint of whether an instance is doing real work or just existing.
To accelerate this process, use CLI queries to capture a snapshot of current state:
aws rds describe-db-instances \
--query "DBInstances[*].{DB:DBInstanceIdentifier,Class:DBInstanceClass,State:DBInstanceStatus,Created:InstanceCreateTime}" \
--output table
Then, compare that against CloudWatch data collected over a 14–31 day window:
aws cloudwatch get-metric-statistics \
--metric-name CPUUtilization \
--start-time 2024-05-01T00:00:00Z \
--end-time 2024-05-31T23:59:59Z \
--period 86400 \
--namespace AWS/RDS \
--statistics Average \
--dimensions Name=DBInstanceIdentifier,Value=my-db
Instances with low CPU and negligible network activity—especially those with stable or declining memory usage—are strong idle candidates.
Validate Business Context Before Acting
Before selecting a remediation path, establish the current relevance of the instance. Use existing tags (Environment
, Project
, Owner
, etc.) to identify responsible teams. If tags are missing or stale, use IAM access patterns, CloudTrail events, or recent login history to trace back recent activity. You can also leverage AWS Resource Groups to list assets tied to specific teams or projects.
When ownership is unclear, append a review tag to the instance:
aws rds add-tags-to-resource \
--resource-name arn:aws:rds:us-east-1:123456789012:db:my-db \
--tags Key=ReviewStatus,Value=NeedsVerification
This creates an audit trail and signals to others that the instance is under evaluation. Once ownership is confirmed or dismissed, update the tag accordingly.
Choose the Right Remediation Strategy
Each idle instance should follow a remediation path based on cost, risk, and future accessibility.
- Delete with Backup: When the instance is confirmed unused and not tied to compliance retention, create a snapshot and remove it entirely:
aws rds create-db-snapshot \
--db-instance-identifier my-db \
--db-snapshot-identifier my-db-final-snapshot
Then:
aws rds delete-db-instance \
--db-instance-identifier my-db \
--final-db-snapshot-identifier my-db-final-snapshot
-
Retain with Adjustments: For rarely accessed but necessary databases, reduce instance size or switch to burstable classes like
db.t4g.micro
to minimize costs:
aws rds modify-db-instance \
--db-instance-identifier my-db \
--db-instance-class db.t4g.micro \
--apply-immediately
- Suspend or Pause: For Aurora Serverless clusters, configure auto-pause to suspend compute when idle:
aws rds modify-db-cluster \
--db-cluster-identifier audit-logs-cluster \
--scaling-configuration MinCapacity=0,AutoPause=true,SecondsUntilAutoPause=3600
These options give you flexibility—whether you need to decommission, minimize, or preserve the database with reduced cost exposure.
Lock Down What You Keep
Idle databases left online must be isolated from unnecessary access. Start by limiting network exposure:
aws rds modify-db-instance \
--db-instance-identifier my-db \
--vpc-security-group-ids sg-00000000 \
--apply-immediately
Attach a security group that denies all inbound connections except from trusted internal ranges or jump boxes. Then remove any IAM roles, Lambda triggers, or SNS topics previously tied to the database. If the database must remain online for archival or reference, add a Quarantine
tag and restrict its visibility in dashboards and monitoring tools.
Automate Detection and Review
For ongoing idle detection, build a Lambda function that runs daily and queries metrics across all RDS instances. Use thresholds like < 1% CPU
and < 5 connections
over 14 days to flag candidates. When flagged, tag them with IdleCandidate=true
and send alerts via SNS or Slack for manual review.
You can also integrate with EventBridge to trigger evaluations on instance creation, ensuring new databases follow lifecycle policies from day one. Pair this with a runbook that outlines remediation protocols, backup policies, and escalation paths. That way, cleanup isn’t a one-time effort—it becomes part of the infrastructure lifecycle.
1. Inventory Your Databases
Start with coverage. Not partial, not “just the prod account”—full-region, full-account visibility. The point isn’t just to surface what’s online, it’s to expose what’s quietly burning budget with no clear owner. Begin with your AWS Organizations account structure. Use list-accounts
with sts assume-role
to pull RDS inventory from every child account. This way, you’re not blind to idle resources running in sandbox or legacy projects someone forgot to shut down three quarters ago.
Here’s how to list RDS instances across multiple accounts and regions using a loop:
for account in $(aws organizations list-accounts --query 'Accounts[*].Id' --output text); do
creds=$(aws sts assume-role --role-arn arn:aws:iam::$account:role/OrgAuditAccess --role-session-name audit-session)
export AWS_ACCESS_KEY_ID=$(echo $creds | jq -r .Credentials.AccessKeyId)
export AWS_SECRET_ACCESS_KEY=$(echo $creds | jq -r .Credentials.SecretAccessKey)
export AWS_SESSION_TOKEN=$(echo $creds | jq -r .Credentials.SessionToken)
for region in $(aws ec2 describe-regions --query 'Regions[*].RegionName' --output text); do
echo "Account: $account | Region: $region"
aws rds describe-db-instances --region $region \
--query "DBInstances[*].{DB:DBInstanceIdentifier,Type:Engine,Class:DBInstanceClass,Status:DBInstanceStatus}" \
--output table
done
done
This gives you a multi-account, multi-region view of your database landscape. Once you’ve got the raw list, push it into a central repository—DynamoDB, Athena, or even a tagged S3 CSV—so you can filter by engine type, region, or creation date later. Tracking it in a living system makes it easier to attach review states over time.
Cross-Reference Metrics Over Time
Once inventory is established, focus on behavior. You’re not just looking for what exists—you’re looking for databases that consistently do nothing. Use AWS Cost Explorer to flag RDS instances with flat spend trends over 30+ days. Then layer in CloudWatch metrics like DatabaseConnections
, ReadIOPS
, and WriteIOPS
to validate inactivity.
Instead of reviewing one instance at a time, automate the metric pull using get-metric-data
. This lets you batch-query multiple instances in a single request:
aws cloudwatch get-metric-data \
--metric-data-queries file://metric-queries.json \
--start-time $(date -u -d '30 days ago' +%Y-%m-%dT00:00:00Z) \
--end-time $(date -u +%Y-%m-%dT00:00:00Z) \
--region us-east-1
Where metric-queries.json
contains:
[
{
"Id": "readiops1",
"MetricStat": {
"Metric": {
"Namespace": "AWS/RDS",
"MetricName": "ReadIOPS",
"Dimensions": [
{
"Name": "DBInstanceIdentifier",
"Value": "my-db-instance"
}
]
},
"Period": 86400,
"Stat": "Sum"
},
"ReturnData": true
}
]
Batching metrics like this helps you identify low-activity patterns at scale, without drowning in one-off CLI calls per instance. You’ll start to see which ones never spike, never connect, and never move data—your top idle suspects.
Capture Fallbacks Before You Touch Anything
Before tagging or terminating anything, document the state. Store metadata like engine version, allocated storage, parameter groups, and security group associations. This makes rollback easier if someone flags the instance post-cleanup. For retention-sensitive environments, record your findings in a separate audit log and attach a flag like IdleCandidate=True
or RemediationStatus=PendingReview
.
If deletion is on the table, use a snapshot strategy that includes naming conventions and timestamping:
aws rds create-db-snapshot \
--db-instance-identifier my-db-instance \
--db-snapshot-identifier idle-snap-my-db-instance-$(date +%Y%m%d)
Don’t just rely on snapshots for backup—use them to prove due diligence. When you’re working across teams or regulated environments, a standardized naming scheme for snapshots and tags helps signal intent and avoids accidental overlaps with active workloads.
2. Evaluate Each Database’s Role
After surfacing idle candidates, the next step is confirming whether they still matter. Metrics show you what’s happening under the hood—but they don’t tell you whether the database still holds business value. That context lives in ownership, integrations, and historical decisions made outside the console.
When metadata is missing or unreliable, trace the origin using CloudTrail’s creation logs. This gives you a timestamp and the IAM identity that created the instance—often enough to connect the dots. Run this to surface the creator:
aws cloudtrail lookup-events \
--lookup-attributes AttributeKey=EventName,AttributeValue=CreateDBInstance \
--query 'Events[*].{Time:EventTime,User:Username,Resource:Resources[0].ResourceName}' \
--max-results 20
If the user is active, a short message asking whether the database supports anything critical can resolve ambiguity fast. If not, look for context in provisioning pipelines, resource policies, or existing role bindings. You’ll often find clues in IAM permissions, ECS task definitions, or Lambda environment variables that reference the database identifier.
Determine Usage Through Connected Systems
Rather than guessing at purpose, check whether the database appears in active configurations. You can scan Secrets Manager and Parameter Store for stored credentials that are still in use by apps or services:
aws secretsmanager list-secrets \
--query "SecretList[?contains(Name, 'my-db')].Name"
aws ssm describe-parameters \
--query "Parameters[?contains(Name, 'my-db')].Name"
Also look at task queues, data pipelines, or scheduled jobs tied to the database. For example, a Redshift cluster may not see daily queries but could be processing monthly reports via a Step Functions state machine or AWS Glue job. In those cases, you’re dealing with low-frequency usage—not true idleness.
Apply Clear, Future-Friendly Tagging
Once you’ve confirmed the role—or lack thereof—tag the instance to document its status and avoid reevaluating it later. Use clear labels like Role=HistoricalReporting
, RemediationStatus=IdleCandidate
, or ReviewDate=2024-06-01
to give future teams context at a glance:
aws rds add-tags-to-resource \
--resource-name arn:aws:rds:us-west-2:123456789012:db:legacy-db \
--tags Key=Role,Value=LegacyData \
Key=RemediationStatus,Value=NeedsApproval \
Key=ReviewDate,Value=2024-06-01
If the database is still under review or lacks a clear owner, tag it accordingly and schedule a follow-up. This creates a paper trail—and avoids false positives the next time someone runs a cleanup script.
The goal here isn’t just to clean up one instance. It’s to embed context into your infrastructure so that future reviews move quickly, decisions are traceable, and idle database management becomes a lightweight, continuous process.
3. Confirm Data Retention Requirements
Before any remediation action—termination, downsizing, or archiving—you need to understand the data’s contractual or regulatory weight. Usage metrics don’t reveal whether a database contains GDPR-affected records, financial logs tied to tax filings, or archive content required by HIPAA. Removing an idle database without validating retention obligations exposes you to compliance violations, not just operational risk.
Start by reviewing your internal data classification policy, if one exists. Many organizations mandate different retention periods for PII, logs, analytical models, or system telemetry. If the database falls under a formal data governance category—like “Regulatory Financials” or “Customer Interaction Logs”—you’ll want to escalate the review to a compliance lead before taking any action. When no classification is available, fall back to metadata like schema names (audit_logs
, transactions
, etc.) or table row counts to infer its historical value.
Export for Long-Term Retention Without Keeping Infrastructure
If you confirm the data must remain accessible beyond the life of the instance, exporting it is a more durable and cost-efficient approach than keeping the instance running or relying solely on RDS snapshots. For example, you can export a snapshot to S3 in Parquet format, which supports downstream querying via Athena without spinning up compute:
aws rds export-db-snapshot \
--db-snapshot-identifier audit-db-snap-20240601 \
--source-engine mysql \
--s3-bucket-name org-compliance-archive \
--iam-role-arn arn:aws:iam::123456789012:role/RDSExportToS3 \
--kms-key-id arn:aws:kms:us-east-1:123456789012:key/abc12345-6789-0123-4567-abcdef123456
This export strategy allows you to retain data in a queryable format, minimize storage costs, and avoid compute resources entirely. It’s especially useful for compliance teams that require access to historical data but don’t need a live database to retrieve it.
Put Lifecycle Controls Around Retained Data
Once exported, the data must follow structured lifecycle controls to avoid becoming the next generation of idle sprawl. Use S3 object tags and bucket lifecycle configurations to enforce expiration:
{
"Rules": [
{
"ID": "ExpireComplianceDataAfter7Years",
"Filter": {
"Tag": {
"Key": "RetentionPolicy",
"Value": "Finance"
}
},
"Status": "Enabled",
"Expiration": {
"Days": 2555
}
}
]
}
Couple this with versioning and access logging to create an auditable trail. If your organization uses centralized security tooling, integrate these exported records into your compliance vault or archival domain, and restrict access via resource-level IAM policies that reference specific tags like DataSensitivity=High
or LegalHold=True
.
Instead of just preserving data reactively, this approach embeds retention logic into the export process—ensuring archived databases follow the same rigor as live systems but without the operational overhead.
4. Apply the Best Remediation Strategy
Once you've finished auditing, tagging, and confirming the purpose of each idle database, the next step is choosing the right remediation path. Not every instance needs to be deleted—your decision should reflect the level of risk, the need for historical access, and the frequency of future use.
Full Termination with Lifecycle Controls
For databases that serve zero ongoing purpose and carry no retention requirements, complete removal is appropriate—but it still requires a structured approach. Before deletion, register the instance in your internal cleanup log and check for any downstream dependencies (e.g., secrets, parameter references, or IAM bindings that might still reference the database). Rather than relying on a single snapshot, consider exporting the database contents to S3 using the ExportDBSnapshot
feature, especially if the data may need to be queried later via Athena or Redshift Spectrum.
aws rds export-db-snapshot \
--db-snapshot-identifier legacy-db-snap-20240601 \
--source-engine postgres \
--s3-bucket-name longterm-archive-bucket \
--iam-role-arn arn:aws:iam::123456789012:role/RDSExportRole \
--kms-key-id arn:aws:kms:us-east-1:123456789012:key/abc123
After confirming the export, follow up with deletion and attach a record of the action to your central resource inventory or security audit trail.
Downsizing to Align with Intermittent Use
Some databases are technically idle but still serve low-frequency workloads—quarterly audits, monthly reports, or ad-hoc queries by internal teams. In those cases, full termination may be excessive. Instead, reduce the footprint to match actual usage. Choose instance classes with burstable capacity (t3
, t4g
) and evaluate whether storage throughput or IOPS provisioning can be scaled down. This tactic preserves availability while significantly reducing runtime costs.
For example, to update a database to a smaller, ARM-based instance class:
aws rds modify-db-instance \
--db-instance-identifier archive-db \
--db-instance-class db.t4g.micro \
--storage-type gp3 \
--apply-immediately
This method is particularly effective when paired with scheduled backups and a defined retention period, allowing the database to remain functional but cost-aligned.
Suspend or Quarantine for Low-Risk Retention
When deletion isn't an option and downsizing doesn’t go far enough—such as in compliance-heavy environments or when ownership is unclear—quarantine is a viable middle ground. This involves isolating the instance from the network, locking down IAM policies, and removing any triggers or downstream integrations. Instead of relying on auto-scaling or pause features alone, you enforce a state of intentional disconnection, reducing exposure without losing the data.
For Aurora Serverless v1 or v2 clusters, where pausing is supported, configure inactivity thresholds explicitly. This allows the cluster to suspend compute resources automatically while data remains intact.
aws rds modify-db-cluster \
--db-cluster-identifier dormant-reporting-cluster \
--scaling-configuration MinCapacity=1,MaxCapacity=2,AutoPause=true,SecondsUntilAutoPause=7200 \
--apply-immediately
For engines that don’t support pause, simulate the effect by disabling inbound access and scheduling instance stops. Use Systems Manager Automation documents or EventBridge rules to shut down the database outside known access windows, reducing runtime costs without removing the instance entirely. This gives you a predictable, low-risk fallback plan while buying time to confirm long-term decisions.
5. Update Network and Access Configurations
Idle databases that remain online often carry legacy access paths—open ports, broad CIDR blocks, or deprecated roles—that were never revisited once the database stopped serving live traffic. These misconfigurations don’t raise alarms on their own but present quiet, persistent risks. If a database is inactive, its exposure should reflect that status with strict, intentional controls.
Start by identifying which security groups are still attached to databases flagged as idle. Use describe-db-instances
to retrieve the associated security group IDs, then inspect the ingress rules:
aws ec2 describe-security-groups \
--group-ids sg-0123456789abcdef0 \
--query "SecurityGroups[*].IpPermissions"
Instead of just removing security groups, consolidate idle instances into a dedicated isolation group with no inbound rules defined—effectively dead-ending all network access:
aws ec2 create-security-group \
--group-name rds-isolation-zone \
--description "Blocked access for inactive RDS" \
--vpc-id vpc-0abc123def456ghij
Apply it to the instance:
aws rds modify-db-instance \
--db-instance-identifier dormant-db \
--vpc-security-group-ids sg-0abc123def456ghij \
--apply-immediately
This replaces any prior rules—public or private—with a locked-down profile. From a network perspective, the database becomes inert without requiring full deletion or snapshot restoration.
IAM roles and policies tied to idle databases often go unchecked. These roles may have been granted permissions like rds:StartDBInstance
or rds:RestoreDBInstanceFromSnapshot
months—or years—ago, and they persist long after their operational use ends. Use CloudTrail to pinpoint which IAM entities have interacted with the instance in the last 90 days:
aws cloudtrail lookup-events \
--lookup-attributes AttributeKey=ResourceName,AttributeValue=dormant-db \
--max-results 50
If no recent activity is found, simulate the role’s permissions to see whether it still has access to sensitive RDS actions:
aws iam simulate-principal-policy \
--policy-source-arn arn:aws:iam::123456789012:role/ObsoleteRDSRole \
--action-names rds:StartDBInstance rds:RestoreDBInstanceFromSnapshot
For roles still permitted to act on idle databases, either remove the policy or modify the trust relationship to prevent future assumptions. For example, to explicitly deny assumptions:
{
"Version": "2012-10-17",
"Statement": [
{
"Sid": "DenyObsoleteAccess",
"Effect": "Deny",
"Action": "sts:AssumeRole",
"Principal": "*",
"Condition": {
"ArnEquals": {
"aws:PrincipalArn": "arn:aws:iam::123456789012:role/ObsoleteRDSRole"
}
}
}
]
}
Outside of network and IAM, configuration bloat can also linger in parameter and option groups. These often accumulate enabled features—like audit logs, minor version preferences, or legacy extensions—that no longer serve their original purpose. Instead of defaulting to a full reset, duplicate the current group, strip out unused settings, and reassign it to the instance:
aws rds modify-db-instance \
--db-instance-identifier dormant-db \
--db-parameter-group-name minimal-config \
--apply-immediately
This approach reduces unnecessary complexity and aligns the database’s configuration with its current (or lack of) workload. It also makes future remediation simpler—leaner configurations mean fewer unknown variables when it’s time to archive, downsize, or delete.
6. Automate Ongoing Idle Detection
Manual reviews don’t scale—not across multiple teams, regions, or accounts. Automation ensures idle database detection becomes a continuous process, not a quarterly fire drill. By embedding scheduled metric evaluation into lightweight Lambda functions, you can catch drift before it becomes budget bloat, and remove guesswork from the equation entirely.
Start with a Lambda function that consumes instance IDs from a centralized inventory—stored in DynamoDB or fetched dynamically—and runs targeted metric queries via CloudWatch APIs. Instead of relying on fixed thresholds, implement configurable logic using environment variables or a lookup table to account for different environments and usage profiles (e.g., production vs. test). This flexibility allows you to tune for noise reduction without missing meaningful signals.
Here’s a basic implementation that uses a DynamoDB table to load instance configurations and supports adjustable thresholds per instance:
import boto3
import datetime
import os
cloudwatch = boto3.client('cloudwatch')
dynamodb = boto3.resource('dynamodb')
rds = boto3.client('rds')
def lambda_handler(event, context):
table = dynamodb.Table(os.environ['INSTANCE_CONFIG_TABLE'])
end = datetime.datetime.utcnow()
start = end - datetime.timedelta(days=7)
items = table.scan()['Items']
for item in items:
db_id = item['DBInstanceIdentifier']
threshold_cpu = float(item.get('CPUThreshold', 2))
threshold_conn = float(item.get('ConnThreshold', 1))
conn_stats = cloudwatch.get_metric_statistics(
Namespace='AWS/RDS',
MetricName='DatabaseConnections',
Dimensions=[{'Name': 'DBInstanceIdentifier', 'Value': db_id}],
StartTime=start,
EndTime=end,
Period=86400,
Statistics=['Average']
)
cpu_stats = cloudwatch.get_metric_statistics(
Namespace='AWS/RDS',
MetricName='CPUUtilization',
Dimensions=[{'Name': 'DBInstanceIdentifier', 'Value': db_id}],
StartTime=start,
EndTime=end,
Period=86400,
Statistics=['Average']
)
conn_avg = sum(dp['Average'] for dp in conn_stats['Datapoints']) / len(conn_stats['Datapoints']) if conn_stats['Datapoints'] else 0
cpu_avg = sum(dp['Average'] for dp in cpu_stats['Datapoints']) / len(cpu_stats['Datapoints']) if cpu_stats['Datapoints'] else 0
if conn_avg < threshold_conn and cpu_avg < threshold_cpu:
rds.add_tags_to_resource(
ResourceName=item['DBInstanceArn'],
Tags=[{'Key': 'IdleCandidate', 'Value': 'true'}]
)
print(f"Marked {db_id} as idle")
This version doesn’t assume one-size-fits-all logic. It adjusts based on the intended behavior of each instance and stores evidence in tagging, enabling downstream systems to take action—or escalate—without human intervention.
To extend this pipeline, integrate the function with an SNS topic or webhook that feeds into your internal ops tooling. Instead of plain alerts, push structured payloads that include instance metadata, thresholds breached, and time-series summaries. This gives reviewers the context they need to make decisions quickly without digging through metrics dashboards.
For long-term traceability, log all detection results and tagging actions to a dedicated S3 bucket or a time-partitioned Athena table. Include not just the instance ID and metric values but also the evaluation config that triggered the action. When teams review past decisions or investigate unexpected deletions, this audit log becomes the source of truth.
Capture your automation stack and detection logic in a shared runbook accessible to all teams involved in infrastructure lifecycle management. Include a decision tree for each remediation path, escalation contacts, and examples of valid idle patterns versus anomalies. When detection becomes embedded into your review cadence, idle cleanup turns from a one-off effort into a predictable, low-friction routine.
Reasons to Keep an Eye on AWS Idle Databases
Idle databases are easy to ignore—until they interfere with your ability to move fast. When your AWS inventory is bloated with unused resources, even simple tasks like running compliance scans or provisioning new environments take longer than they should. Visibility suffers, and so does the confidence that what you're deploying into is actually being used, secured, and maintained.
Unmonitored databases tend to operate outside of your standard guardrails. They might still be accessible from legacy IP ranges, tied to IAM roles no one remembers creating, or logging to buckets that are no longer monitored. One idle PostgreSQL instance left with unrestricted ingress for weeks can quietly sidestep your perimeter defenses—not because of negligence, but because no one knew it was still there.
Infrastructure That Doesn’t Fight Back
Clean infrastructure doesn’t just save money—it reduces resistance. When teams spin up new workloads or migrate existing ones, they aren’t forced to decipher what’s safe to reuse or what might break something. There’s no guessing if a database labeled "test-db-v2" is critical or disposable. By keeping idle databases out of the way, you prevent them from becoming blockers in future planning or sources of accidental regression.
As environments scale up, the risk of misclassifying resources grows. A database that supported a proof-of-concept last quarter might still sit on a large instance class, untouched but protected by deletion safeguards. Without a consistent way to surface and review these kinds of leftovers, capacity planning and cost forecasting get skewed—and so do your infrastructure decisions.
aws rds describe-db-instances \
--query "DBInstances[?contains(DBInstanceIdentifier, 'poc') && @.DBInstanceStatus=='available']" \
--output table
Use this to isolate candidate instances by naming convention and status—then evaluate whether they’ve had any active connections or IOPS in the last 30 days. It’s not just about finding what’s idle. It’s about knowing what’s safe to ignore—and what isn’t—before it affects your next deployment.
Tips on AWS Database Remediation
1. Tag Rigorously
Tagging is only useful if it’s opinionated and enforced. It's not enough to label something “dev” or “test”—your tags should declare intent. Tags like Owner
or Purpose
are just the start. Introduce tags that reflect lifecycle (TTL
), cost center (BillingCode
), and compliance scope (DataSensitivity
). These tags help automation scripts decide what to ignore, what to archive, and what to shut down without involving a human.
For example, when provisioning a database, inject a tag set that answers three questions: who owns it, when does it expire, and what kind of data lives inside?
aws rds create-db-instance \
--db-instance-identifier campaign-metrics \
--db-instance-class db.t3.small \
--engine postgres \
--tags Key=Owner,Value=team.analytics \
Key=TTL,Value=2024-09-30 \
Key=DataSensitivity,Value=PII \
Key=BillingCode,Value=MKT-Q3-2024
To enforce tagging at org level, use AWS Organizations tag policies to define allowed tag keys and value patterns. Combine that with Config rules that flag instances not matching policy. For high-sensitivity environments, pair tag-based access control with IAM condition keys to prevent untagged resources from being created altogether.
2. Embrace Snapshots
Snapshots are more than just a safety net—they’re a critical part of your idle database strategy. Instead of keeping dormant databases online “just in case,” convert them into snapshots that can be retained in low-cost storage tiers or exported to S3 in a queryable format. This gives teams access to historical data without incurring compute or high IOPS charges.
Use snapshot exports when the data needs to be queried periodically but doesn’t justify a running instance. For example, exporting a snapshot to S3 in Parquet format allows it to be queried directly with Athena:
aws rds export-db-snapshot \
--db-snapshot-identifier campaign-metrics-final-snap \
--source-engine postgres \
--s3-bucket-name analytics-historical-data \
--iam-role-arn arn:aws:iam::123456789012:role/RDSExportRole \
--kms-key-id arn:aws:kms:us-east-1:123456789012:key/abc123xyz
For retention tracking, tag your snapshots with RetentionWindow
and ArchiveStatus
. Then use a scheduled Lambda function to delete anything beyond your defined policy. This keeps storage lean and aligns with internal data governance requirements—without needing a human to remember what’s safe to delete.
Final Thoughts
Treat idle database management like documentation: not glamorous, but essential, and painful when neglected. The teams that stay ahead don’t rely on quarterly cleanups—they build systems that flag drift automatically and make deletion feel safe, not risky. It’s less about tooling and more about culture. Infrastructure that reflects the present, not the past, is easier to operate and trust.
Cleaning up unused databases reduces more than cost—it removes cognitive load. Every forgotten RDS instance introduces friction: in audits, in migration plans, in monitoring noise. When you've got fewer unknowns, you don’t waste cycles second-guessing what’s still in use. Instead, your team can focus on the infrastructure that actually powers the business.
The strongest environments aren’t the ones with the most controls—they’re the ones with the fewest surprises. Retiring what doesn’t serve a clear purpose clears the way for faster decisions, simpler security, and cleaner growth.
How to Find and Safely Remediate Idle Databases on AWS: Frequently Asked Questions
Q: What if I suspect a DB is idle but might be used quarterly or yearly?
Start by expanding the observation window. Instead of reviewing week-over-week usage, query CloudWatch for trends over the past 90 to 180 days. Look for recognizable usage spikes tied to business cycles—end-of-quarter reporting, annual audits, or seasonal traffic simulations. If the pattern holds, label the instance accordingly and avoid full remediation.
For these low-frequency workloads, consider shifting the database to a suspended or paused state if the engine supports it. Aurora Serverless, for example, can be configured to auto-pause after inactivity, which cuts compute costs while preserving availability. If pause isn’t supported, use Systems Manager or EventBridge to implement recurring stop/start routines aligned to expected activity windows.
Q: How can automation help me avoid future idle database sprawl?
The most effective automation happens before the database exists. Integrate tagging requirements into your provisioning pipelines so every new instance carries metadata like Owner
, Project
, and ExpectedTTL
. Then use AWS Config to continuously monitor for missing or misaligned tags and trigger remediation workflows.
For ongoing detection, implement a scheduled Lambda that pulls metrics like DatabaseConnections
and ReadIOPS
across your RDS estate. Cross-reference those with a DynamoDB table that tracks expected activity profiles. When a database falls outside its expected behavior, tag it for review or trigger a notification for the owning team. This creates a lightweight but persistent idle detection loop that evolves with your infrastructure.
Q: Are there any data compliance or regulatory concerns with deleting a DB?
Yes—and they’re often not immediately visible in the AWS resource itself. Compliance risks usually live in the data schema, not the instance metadata. Before any deletion, assess whether the database contains records tied to financial audits, customer interactions, medical logs, or legal holds. These datasets often fall under industry or jurisdictional mandates for long-term retention.
If data must be preserved, export it to an immutable medium. Use RDS snapshot exports to store the data in columnar formats like Parquet, which supports long-term archival and serverless querying. Apply S3 object tags like DataClassification=Regulated
and configure bucket policies that enforce encryption and access restrictions. This gives you retention without incurring compute costs or leaving services running.
Q: Is there a single best solution for all idle databases?
No, because each idle database represents a different context. Some are no-brainers to delete—test environments with zero connections for 30+ days. Others need to stick around for compliance, but not in their current form. The remediation strategy should reflect how often the data is accessed, what kind of data it holds, and the cost of keeping it online.
Think in terms of tiers. For databases that are completely dormant, snapshot and delete. For those with intermittent access or unknown status, isolate and shrink. For compliance-hardened workloads, export and archive. The key is to match remediation depth with operational risk—so that the cleanup effort doesn’t create more problems than it solves.
Managing idle databases isn’t just about cost—it's about clarity, control, and confidence in your infrastructure. When you know what's running and why, you can scale smarter and avoid painful surprises down the line.