Create High-Quality AI Videos with Wan2.1 FLF2V 14B: A Step-By-Step Guide
Imagine creating stunning videos from simple text prompts, editing existing videos seamlessly, or even generating realistic sounds directly from videos all on your own computer. That’s exactly what Wan2.1 FLF2V 14B makes possible. Wan2.1 is an open source suite of video generative models that’s redefining the possibilities of AI video creation. It doesn't just generate short clips, it supports Text-to-Video, Image-to-Video, Video Editing, Text-to-Image, and even Video-to-Audio tasks, all with top-notch quality. On GPUs like H100 or H200, Wan2.1 runs smoothly and delivers performance that outperforms top-tier commercial solutions. For video creators, developers, marketers, Wan2.1 gives the power to bring creative ideas to life, with faster and better results than ever before. In this guide, we'll walk you through a simple step-by-step process to install and run Wan2.1 FLF2V 14B locally or with on cloud GPUs, so you can start creating realistic and magical videos in just a few steps. Prerequisites The minimum system requirements for this use case are: GPUs: 2x H100 or 1x H200 Disk Space: 100 GB RAM: At least 80 GB. Anaconda set up Note: The prerequisites for this are highly variable across use cases. A high-end configuration could be used for a large-scale deployment. Step-by-step process to install and run Wan2.1 FLF2V Locally For the purpose of this tutorial, we’ll use a GPU-powered Virtual Machine by NodeShift since it provides high compute Virtual Machines at a very affordable cost on a scale that meets GDPR, SOC2, and ISO27001 requirements. Also, it offers an intuitive and user-friendly interface, making it easier for beginners to get started with Cloud deployments. However, feel free to use any cloud provider of your choice and follow the same steps for the rest of the tutorial. Step 1: Setting up a NodeShift Account Visit app.nodeshift.com and create an account by filling in basic details, or continue signing up with your Google/GitHub account. If you already have an account, login straight to your dashboard. Step 2: Create a GPU Node After accessing your account, you should see a dashboard (see image), now: 1) Navigate to the menu on the left side. 2) Click on the GPU Nodes option. 3) Click on Start to start creating your very first GPU node. These GPU nodes are GPU-powered virtual machines by NodeShift. These nodes are highly customizable and let you control different environmental configurations for GPUs ranging from H100s to A100s, CPUs, RAM, and storage, according to your needs. Step 3: Selecting configuration for GPU (model, region, storage) 1) For this tutorial, we’ll be using the H200 GPU; however, you can choose any GPU of your choice based on your needs. 2) Similarly, we’ll opt for 100GB storage by sliding the bar. You can also select the region where you want your GPU to reside from the available ones. Step 4: Choose GPU Configuration and Authentication method 1) After selecting your required configuration options, you'll see the available GPU nodes in your region and according to (or very close to) your configuration. In our case, we'll choose a 1x H200 140GB GPU node with 192vCPUs/252GB RAM/100GB SSD. 2) Next, you'll need to select an authentication method. Two methods are available: Password and SSH Key. We recommend using SSH keys, as they are a more secure option. To create one, head over to our official documentation. Step 5: Choose an Image The final step would be to choose an image for the VM, which in our case is Nvidia Cuda, where we’ll deploy and run the inference of our model. That's it! You are now ready to deploy the node. Finalize the configuration summary, and if it looks good, click Create to deploy the node. Step 6: Connect to active Compute Node using SSH 1) As soon as you create the node, it will be deployed in a few seconds or a minute. Once deployed, you will see a status Running in green, meaning that our Compute node is ready to use! 2) Once your GPU shows this status, navigate to the three dots on the right and click on Connect with SSH. This will open a pop-up box with the Host details. Copy and paste that in your local terminal to connect to the remote server via SSH. As you copy the details, follow the below steps to connect to the running GPU VM via SSH: 1) Open your terminal, paste the SSH command, and run it. 2) In some cases, your terminal may take your consent before connecting. Enter ‘yes’. 3) A prompt will request a password. Type the SSH password, and you should be connected. Output: Next, If you want to check the GPU details, run the following command in the terminal: !nvidia-smi Step 7: Set up the project environment with dependencies 1) Create a virtual environment using Anaconda. conda create -n wan python=3.11 && conda activate wan Output: 2) Clone the official repository of Orpheus
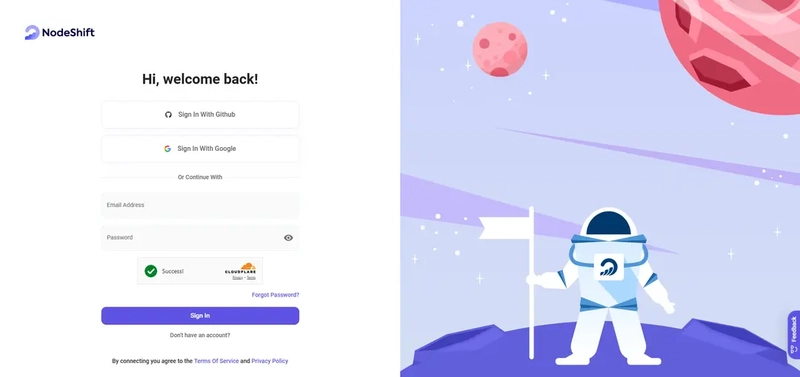
Imagine creating stunning videos from simple text prompts, editing existing videos seamlessly, or even generating realistic sounds directly from videos all on your own computer. That’s exactly what Wan2.1 FLF2V 14B makes possible. Wan2.1 is an open source suite of video generative models that’s redefining the possibilities of AI video creation. It doesn't just generate short clips, it supports Text-to-Video, Image-to-Video, Video Editing, Text-to-Image, and even Video-to-Audio tasks, all with top-notch quality. On GPUs like H100 or H200, Wan2.1 runs smoothly and delivers performance that outperforms top-tier commercial solutions. For video creators, developers, marketers, Wan2.1 gives the power to bring creative ideas to life, with faster and better results than ever before.
In this guide, we'll walk you through a simple step-by-step process to install and run Wan2.1 FLF2V 14B locally or with on cloud GPUs, so you can start creating realistic and magical videos in just a few steps.
Prerequisites
The minimum system requirements for this use case are:
GPUs: 2x H100 or 1x H200
Disk Space: 100 GB
RAM: At least 80 GB.
Note: The prerequisites for this are highly variable across use cases. A high-end configuration could be used for a large-scale deployment.
Step-by-step process to install and run Wan2.1 FLF2V Locally
For the purpose of this tutorial, we’ll use a GPU-powered Virtual Machine by NodeShift since it provides high compute Virtual Machines at a very affordable cost on a scale that meets GDPR, SOC2, and ISO27001 requirements. Also, it offers an intuitive and user-friendly interface, making it easier for beginners to get started with Cloud deployments. However, feel free to use any cloud provider of your choice and follow the same steps for the rest of the tutorial.
Step 1: Setting up a NodeShift Account
Visit app.nodeshift.com and create an account by filling in basic details, or continue signing up with your Google/GitHub account.
If you already have an account, login straight to your dashboard.
Step 2: Create a GPU Node
After accessing your account, you should see a dashboard (see image), now:
1) Navigate to the menu on the left side.
2) Click on the GPU Nodes option.
3) Click on Start to start creating your very first GPU node.
These GPU nodes are GPU-powered virtual machines by NodeShift. These nodes are highly customizable and let you control different environmental configurations for GPUs ranging from H100s to A100s, CPUs, RAM, and storage, according to your needs.
Step 3: Selecting configuration for GPU (model, region, storage)
1) For this tutorial, we’ll be using the H200 GPU; however, you can choose any GPU of your choice based on your needs.
2) Similarly, we’ll opt for 100GB storage by sliding the bar. You can also select the region where you want your GPU to reside from the available ones.
Step 4: Choose GPU Configuration and Authentication method
1) After selecting your required configuration options, you'll see the available GPU nodes in your region and according to (or very close to) your configuration. In our case, we'll choose a 1x H200 140GB GPU node with 192vCPUs/252GB RAM/100GB SSD.
2) Next, you'll need to select an authentication method. Two methods are available: Password and SSH Key. We recommend using SSH keys, as they are a more secure option. To create one, head over to our official documentation.
Step 5: Choose an Image
The final step would be to choose an image for the VM, which in our case is Nvidia Cuda, where we’ll deploy and run the inference of our model.
That's it! You are now ready to deploy the node. Finalize the configuration summary, and if it looks good, click Create to deploy the node.
Step 6: Connect to active Compute Node using SSH
1) As soon as you create the node, it will be deployed in a few seconds or a minute. Once deployed, you will see a status Running in green, meaning that our Compute node is ready to use!
2) Once your GPU shows this status, navigate to the three dots on the right and click on Connect with SSH. This will open a pop-up box with the Host details. Copy and paste that in your local terminal to connect to the remote server via SSH.
As you copy the details, follow the below steps to connect to the running GPU VM via SSH:
1) Open your terminal, paste the SSH command, and run it.
2) In some cases, your terminal may take your consent before connecting. Enter ‘yes’.
3) A prompt will request a password. Type the SSH password, and you should be connected.
Output:
Next, If you want to check the GPU details, run the following command in the terminal:
!nvidia-smi
Step 7: Set up the project environment with dependencies
1) Create a virtual environment using Anaconda.
conda create -n wan python=3.11 && conda activate wan
Output:
2) Clone the official repository of Orpheus TTS and move inside the project directory.
git clone https://github.com/Wan-Video/Wan2.1.git && cd Wan2.1
Output:
3) Once you're inside the project directory, install project dependencies as mentioned in below.
pip install torch torchvision torchaudio --index-url https://download.pytorch.org/whl/cu121
pip install --no-cache-dir flash-attn --no-build-isolation
Output:
4) Install dependencies mentioned in requirements.txt.
pip install -r requirements.txt
Output:
Step 8: Download and Run the model
1) Since this is a gated mode, we'll need to first login to huggingface-cli
with our access token.
(Enter your HF READ
Token when prompted)
huggingface-cli login
Output:
2) Download the model checkpoints.
huggingface-cli download Wan-AI/Wan2.1-T2V-14B --local-dir ./Wan2.1-T2V-14B
Output:
3) Run the model with prompt for inference.
python generate.py --task t2v-14B --size 1280*720 --ckpt_dir ./Wan2.1-T2V-14B --prompt "Two anthropomorphic cats in comfy boxing gear and bright gloves fight intensely on a spotlighted stage."
Output:
After the model has successfully completed generating the video, you'll be able to see and download the output video from project directory in Visual Studio Code Editor. For this, if you're using a remote server (e.g. NodeShift GPU), you'll first need to connect your local VS Code editor to your remote server via SSH with the following steps:
a) Install the “Remote-SSH” Extension by Microsoft on VS Code.
b) Type “Remote-SSH: Connect to Host” on the Command Palette.
c) Enter the host details, such as username and SSH password, and you should be connected.
Here's the downloaded output video:
https://drive.google.com/drive/folders/1IsmukyaG8iaftLHkEDMug3xx1SHrCCr_?usp=sharing
Conclusion
By following this guide, you’ve learned how to install and run Wan2.1 FLF2V 14B locally, unlocking powerful capabilities like Text-to-Video, Image-to-Video, and Video-to-Audio generation right at your fingertips. Whether you’re experimenting on your personal machine or scaling up projects, NodeShift makes it even easier by providing instant access to high-performance GPUs like H100 and H200, optimized specifically for demanding models like Wan2.1 but without breaking the bank.
For more information about NodeShift: