AI-Powered Test Data Generation: Ensuring Quality with Synthetic Data
In the modern era of software development, high-quality test data is crucial for ensuring the robustness and reliability of applications. However, acquiring real-world data often comes with challenges such as privacy concerns, regulatory compliance, and data inconsistencies. AI-powered test data generation has emerged as a transformative solution, enabling businesses to create synthetic datasets that mimic real-world scenarios without exposing sensitive information. The Role of AI in Test Data Generation Traditional methods of generating test data often involve manual entry, data masking, or using outdated datasets, all of which have limitations in scalability and accuracy. AI-driven solutions, on the other hand, leverage machine learning and statistical modeling to generate realistic, diverse, and high-quality synthetic data. These models analyze existing data patterns and create variations that maintain data integrity while eliminating security risks. Key advantages of AI-powered test data generation include: Data Privacy and Security: Since synthetic data is not derived from actual user records, it mitigates compliance risks associated with regulations like GDPR and CCPA. Scalability: AI can generate large volumes of diverse test cases, ensuring comprehensive testing for different scenarios. Data Quality and Consistency: AI-driven tools can eliminate inconsistencies and anomalies, leading to more reliable test data. Cost Efficiency: Automating test data generation reduces the time and effort spent on manual processes. Use Cases for Synthetic Test Data Software Testing & QA — AI-generated test data ensures better coverage for edge cases and real-world scenarios. Machine Learning Model Training — Synthetic data can supplement real datasets, improving model accuracy and reducing bias. Data Migration & Integration — When transferring data across systems, AI-generated test data helps validate the process without exposing sensitive information. Performance Testing — Large-scale synthetic datasets can simulate real-world loads, helping teams optimize application performance. Genqe.ai and the Future of AI-Powered Test Data As AI-powered test data generation continues to evolve, companies like Genqe.ai are at the forefront of this innovation, offering cutting-edge solutions to help businesses generate high-quality synthetic datasets. By leveraging advanced algorithms, these platforms enable organizations to enhance software testing, ensure compliance, and streamline development processes. Conclusion AI-driven test data generation is revolutionizing the way businesses approach software testing and data privacy. With the ability to create realistic yet secure datasets, organizations can improve application quality while minimizing compliance risks. As the demand for reliable test data grows, AI-powered solutions will play an increasingly vital role in shaping the future of software development and data management.
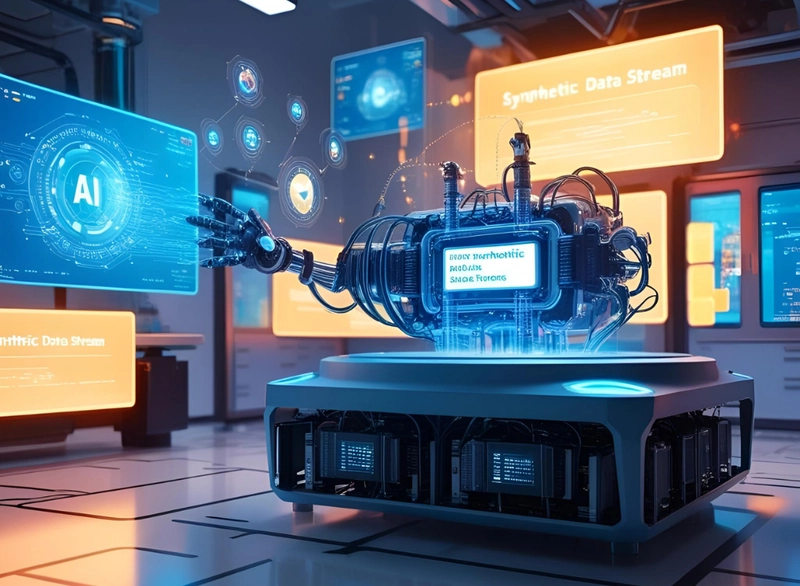
In the modern era of software development, high-quality test data is crucial for ensuring the robustness and reliability of applications. However, acquiring real-world data often comes with challenges such as privacy concerns, regulatory compliance, and data inconsistencies. AI-powered test data generation has emerged as a transformative solution, enabling businesses to create synthetic datasets that mimic real-world scenarios without exposing sensitive information.
The Role of AI in Test Data Generation
Traditional methods of generating test data often involve manual entry, data masking, or using outdated datasets, all of which have limitations in scalability and accuracy. AI-driven solutions, on the other hand, leverage machine learning and statistical modeling to generate realistic, diverse, and high-quality synthetic data. These models analyze existing data patterns and create variations that maintain data integrity while eliminating security risks.
Key advantages of AI-powered test data generation include:
Data Privacy and Security: Since synthetic data is not derived from actual user records, it mitigates compliance risks associated with regulations like GDPR and CCPA.
Scalability: AI can generate large volumes of diverse test cases, ensuring comprehensive testing for different scenarios.
Data Quality and Consistency: AI-driven tools can eliminate inconsistencies and anomalies, leading to more reliable test data.
Cost Efficiency: Automating test data generation reduces the time and effort spent on manual processes.
Use Cases for Synthetic Test Data
Software Testing & QA — AI-generated test data ensures better coverage for edge cases and real-world scenarios.
Machine Learning Model Training — Synthetic data can supplement real datasets, improving model accuracy and reducing bias.
Data Migration & Integration — When transferring data across systems, AI-generated test data helps validate the process without exposing sensitive information.
Performance Testing — Large-scale synthetic datasets can simulate real-world loads, helping teams optimize application performance.
Genqe.ai and the Future of AI-Powered Test Data
As AI-powered test data generation continues to evolve, companies like Genqe.ai are at the forefront of this innovation, offering cutting-edge solutions to help businesses generate high-quality synthetic datasets. By leveraging advanced algorithms, these platforms enable organizations to enhance software testing, ensure compliance, and streamline development processes.
Conclusion
AI-driven test data generation is revolutionizing the way businesses approach software testing and data privacy. With the ability to create realistic yet secure datasets, organizations can improve application quality while minimizing compliance risks. As the demand for reliable test data grows, AI-powered solutions will play an increasingly vital role in shaping the future of software development and data management.