AI Math vs. Reality: Category Theory Exposes the Gap
This is a Plain English Papers summary of a research paper called AI Math vs. Reality: Category Theory Exposes the Gap. If you like these kinds of analysis, you should join AImodels.fyi or follow us on Twitter. The Gap Between AI Math Claims and Reality Recent claims by AI companies tout impressive mathematical capabilities. OpenAI's o3-mini reportedly solved 80% of problems on the American Invitational Mathematics Examination 2024, while xAI's Grok-3 claims similar excellence in math and physics. These assertions clash dramatically with academic research suggesting AI systems can solve only 2% of mathematical research problems. This stark disparity motivated mathematician Răzvan Diaconescu to conduct a focused experiment testing how leading AI systems perform on category theory problems. The goal was twofold: understand how AI might assist working mathematicians and identify improvement opportunities for AI developers. The experiment, detailed in this research paper, reveals the significant gap between marketing claims and actual mathematical capabilities of current AI systems. This gap connects to broader discussions about AI's inherent mathematical difficulties that limit even the most advanced models. Methodology: Selecting a Suitable Mathematical Problem The researcher designed a methodical approach with three components: Choice of mathematical area: Category theory was selected as ideal because it's well-recognized with standardized concepts and extensive literature that AI systems should easily access. Selection of an appropriate problem: The researcher sought a problem that was straightforward yet not trivial, clear to formulate, but not commonly found in textbooks. This led to selecting a problem involving inclusion systems - a concept with sufficient literature presence but not mainstream enough to be a standard exercise. Analysis framework: The evaluation examined three aspects of AI performance: Data gathering capabilities Mathematical language quality Reasoning quality Some might argue that selecting a less mainstream topic deliberately hinders AI systems. However, this reflects the reality of mathematical research, where professionals tackle problems that haven't been extensively studied rather than standard exercises. This experimental design connects to broader research on bridging mathematics and AI creativity, exploring the limits of machine capabilities in mathematical domains. The Problem and Its Solution: Inclusion Systems and Pullbacks Understanding Inclusion Systems Inclusion systems were introduced in theoretical computer science but have since found applications in both computer science and logic, particularly model theory. The concept provides an abstract axiomatic approach to substructures and quotient structures across various categories. Definition: A pair of categories (ℐ,ℰ) is an inclusion system for a category
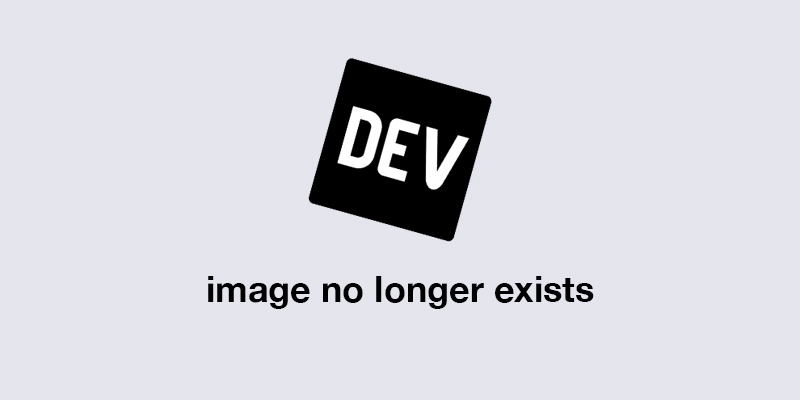
This is a Plain English Papers summary of a research paper called AI Math vs. Reality: Category Theory Exposes the Gap. If you like these kinds of analysis, you should join AImodels.fyi or follow us on Twitter.
The Gap Between AI Math Claims and Reality
Recent claims by AI companies tout impressive mathematical capabilities. OpenAI's o3-mini reportedly solved 80% of problems on the American Invitational Mathematics Examination 2024, while xAI's Grok-3 claims similar excellence in math and physics. These assertions clash dramatically with academic research suggesting AI systems can solve only 2% of mathematical research problems.
This stark disparity motivated mathematician Răzvan Diaconescu to conduct a focused experiment testing how leading AI systems perform on category theory problems. The goal was twofold: understand how AI might assist working mathematicians and identify improvement opportunities for AI developers.
The experiment, detailed in this research paper, reveals the significant gap between marketing claims and actual mathematical capabilities of current AI systems. This gap connects to broader discussions about AI's inherent mathematical difficulties that limit even the most advanced models.
Methodology: Selecting a Suitable Mathematical Problem
The researcher designed a methodical approach with three components:
Choice of mathematical area: Category theory was selected as ideal because it's well-recognized with standardized concepts and extensive literature that AI systems should easily access.
Selection of an appropriate problem: The researcher sought a problem that was straightforward yet not trivial, clear to formulate, but not commonly found in textbooks. This led to selecting a problem involving inclusion systems - a concept with sufficient literature presence but not mainstream enough to be a standard exercise.
-
Analysis framework: The evaluation examined three aspects of AI performance:
- Data gathering capabilities
- Mathematical language quality
- Reasoning quality
Some might argue that selecting a less mainstream topic deliberately hinders AI systems. However, this reflects the reality of mathematical research, where professionals tackle problems that haven't been extensively studied rather than standard exercises.
This experimental design connects to broader research on bridging mathematics and AI creativity, exploring the limits of machine capabilities in mathematical domains.
The Problem and Its Solution: Inclusion Systems and Pullbacks
Understanding Inclusion Systems
Inclusion systems were introduced in theoretical computer science but have since found applications in both computer science and logic, particularly model theory. The concept provides an abstract axiomatic approach to substructures and quotient structures across various categories.
Definition: A pair of categories (ℐ,ℰ) is an inclusion system for a category