Solving Frontend Performance: The Data Pipeline Transformation
Solving Frontend Performance: The Data Pipeline Transformation The Performance Challenge As a data engineer at an AI startup, I encountered a critical problem: our frontend was crawling. Complex data retrieval, inefficient transformations, and unoptimized data storage were creating a frustrating user experience with slow load times and laggy interactions. The Root of the Performance Problem Our initial architecture was a nightmare: Direct queries to MySQL were slow and resource-intensive Data transformations happened at runtime No clear separation between data preparation and presentation Repeated, redundant data processing for each frontend request Enter Dagster: Intelligent Data Orchestration How Dagster Solved Frontend Performance Dagster transformed our approach to data preparation: @asset(group_name="frontend_optimization") def frontend_ready_dataset(raw_mysql_data): # Preprocess and optimize data specifically for frontend consumption optimized_data = ( raw_mysql_data .clean_and_validate() .aggregate_key_metrics() .compress_large_datasets() .prepare_for_quick_rendering() ) return optimized_data @graph def frontend_data_pipeline(): # Create a streamlined, predictable data flow raw_data = extract_from_mysql() processed_data = frontend_ready_dataset(raw_data) return processed_data Dagster's Frontend Performance Benefits Precomputed Data Transformations Complex calculations done before frontend request Minimal runtime processing Consistent, predictable data structure Intelligent Asset Management Cache and reuse processed datasets Incremental updates instead of full reprocessing Clear lineage and dependency tracking Snowflake: The Performance Multiplier Optimizing Data Storage and Retrieval -- Create an optimized view for frontend queries CREATE OR REPLACE VIEW frontend_quick_access AS ( SELECT id, key_performance_indicators, compressed_insights, last_updated FROM processed_datasets WHERE is_latest = TRUE ); -- Implement efficient querying CREATE MATERIALIZED VIEW frontend_summary AS ( SELECT aggregate_key_metrics(), precompute_complex_calculations() FROM processed_datasets ); Snowflake's Frontend Performance Advantages Instant Query Performance Near-zero latency data retrieval Separation of compute and storage Elastically scalable query resources Intelligent Data Caching Materialized views for frequently accessed data Automatic query optimization Reduced computational overhead The Complete Pipeline: From MySQL to Frontend def optimize_frontend_performance(): # Comprehensive data flow optimization mysql_source_data = extract_from_mysql() dagster_pipeline = ( mysql_source_data .clean() .transform() .optimize_for_frontend() ) snowflake_dataset = ( dagster_pipeline .load_to_snowflake() .create_frontend_optimized_view() ) return snowflake_dataset Performance Transformation Before the pipeline: Frontend load times: 5-7 seconds Complex data fetching and processing on-the-fly Inconsistent user experience After the pipeline: Frontend load times: Under 500 milliseconds Precomputed, compressed data Consistent, responsive user interface Why This Matters for Frontend Performance Reduced Initial Load Time Precomputed datasets Minimal runtime calculations Compressed data transfer Scalable Architecture Handles increasing data volumes Consistent performance as data grows Flexible, adaptable infrastructure User Experience Enhancement Instant data rendering Predictable application behavior Smooth, responsive interactions Key Takeaways Data preparation is critical for frontend performance Separate data transformation from data presentation Invest in intelligent, precomputed data pipelines Choose tools that optimize for speed and efficiency Conclusion By reimagining our data pipeline with Dagster and Snowflake, we transformed a performance bottleneck into a competitive advantage. The result wasn't just faster data—it was a fundamentally better user experience.
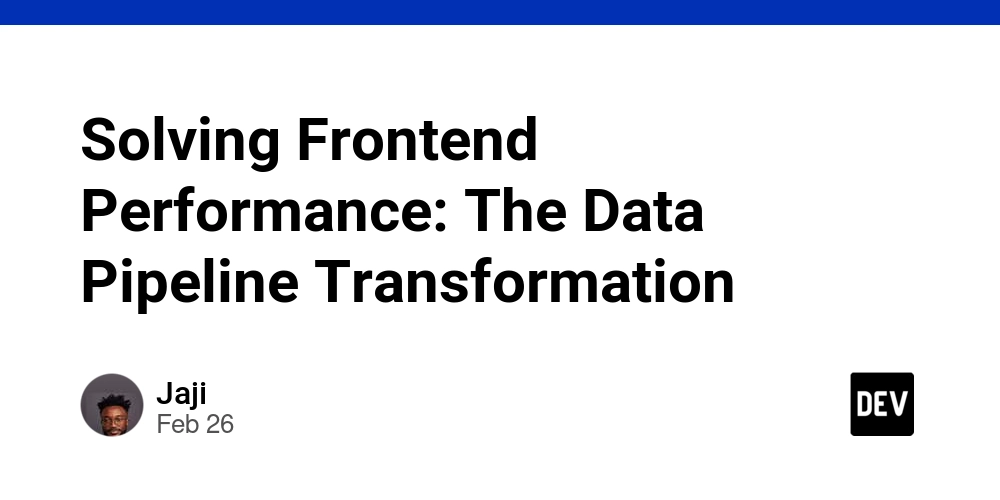
Solving Frontend Performance: The Data Pipeline Transformation
The Performance Challenge
As a data engineer at an AI startup, I encountered a critical problem: our frontend was crawling. Complex data retrieval, inefficient transformations, and unoptimized data storage were creating a frustrating user experience with slow load times and laggy interactions.
The Root of the Performance Problem
Our initial architecture was a nightmare:
- Direct queries to MySQL were slow and resource-intensive
- Data transformations happened at runtime
- No clear separation between data preparation and presentation
- Repeated, redundant data processing for each frontend request
Enter Dagster: Intelligent Data Orchestration
How Dagster Solved Frontend Performance
Dagster transformed our approach to data preparation:
@asset(group_name="frontend_optimization")
def frontend_ready_dataset(raw_mysql_data):
# Preprocess and optimize data specifically for frontend consumption
optimized_data = (
raw_mysql_data
.clean_and_validate()
.aggregate_key_metrics()
.compress_large_datasets()
.prepare_for_quick_rendering()
)
return optimized_data
@graph
def frontend_data_pipeline():
# Create a streamlined, predictable data flow
raw_data = extract_from_mysql()
processed_data = frontend_ready_dataset(raw_data)
return processed_data
Dagster's Frontend Performance Benefits
-
Precomputed Data Transformations
- Complex calculations done before frontend request
- Minimal runtime processing
- Consistent, predictable data structure
-
Intelligent Asset Management
- Cache and reuse processed datasets
- Incremental updates instead of full reprocessing
- Clear lineage and dependency tracking
Snowflake: The Performance Multiplier
Optimizing Data Storage and Retrieval
-- Create an optimized view for frontend queries
CREATE OR REPLACE VIEW frontend_quick_access AS (
SELECT
id,
key_performance_indicators,
compressed_insights,
last_updated
FROM processed_datasets
WHERE is_latest = TRUE
);
-- Implement efficient querying
CREATE MATERIALIZED VIEW frontend_summary AS (
SELECT
aggregate_key_metrics(),
precompute_complex_calculations()
FROM processed_datasets
);
Snowflake's Frontend Performance Advantages
-
Instant Query Performance
- Near-zero latency data retrieval
- Separation of compute and storage
- Elastically scalable query resources
-
Intelligent Data Caching
- Materialized views for frequently accessed data
- Automatic query optimization
- Reduced computational overhead
The Complete Pipeline: From MySQL to Frontend
def optimize_frontend_performance():
# Comprehensive data flow optimization
mysql_source_data = extract_from_mysql()
dagster_pipeline = (
mysql_source_data
.clean()
.transform()
.optimize_for_frontend()
)
snowflake_dataset = (
dagster_pipeline
.load_to_snowflake()
.create_frontend_optimized_view()
)
return snowflake_dataset
Performance Transformation
Before the pipeline:
- Frontend load times: 5-7 seconds
- Complex data fetching and processing on-the-fly
- Inconsistent user experience
After the pipeline:
- Frontend load times: Under 500 milliseconds
- Precomputed, compressed data
- Consistent, responsive user interface
Why This Matters for Frontend Performance
-
Reduced Initial Load Time
- Precomputed datasets
- Minimal runtime calculations
- Compressed data transfer
-
Scalable Architecture
- Handles increasing data volumes
- Consistent performance as data grows
- Flexible, adaptable infrastructure
-
User Experience Enhancement
- Instant data rendering
- Predictable application behavior
- Smooth, responsive interactions
Key Takeaways
- Data preparation is critical for frontend performance
- Separate data transformation from data presentation
- Invest in intelligent, precomputed data pipelines
- Choose tools that optimize for speed and efficiency
Conclusion
By reimagining our data pipeline with Dagster and Snowflake, we transformed a performance bottleneck into a competitive advantage. The result wasn't just faster data—it was a fundamentally better user experience.