Running Wild with LLMs: 10 Open-Source Models You Can Tame on Your Local Machine
The AI Revolution in Your Pajamas Hey there, fellow code wranglers and AI enthusiasts! Remember when running a language model meant renting out half of AWS and selling a kidney? Well, grab your favorite caffeinated beverage because those days are gone. We're diving into the wonderful world of open-source LLMs you can run on your trusty local machine. No cloud required, pants optional. Why Local LLMs? Because Sometimes, You Just Want to be Alone with Your AI Before we jump into our list, let's talk about why you'd want to run an LLM locally: Privacy: Your conversations stay between you and your computer. No eavesdropping clouds here! Offline access: Internet down? No problem. Your AI buddy is always there for you. Customization: Tweak and train to your heart's content. Make an LLM that truly gets your obscure Star Trek references. Cost-effective: Save those cloud computing dollars for something more important. Like coffee. Or more RAM. Alright, let's meet our contestants! 1. GPT-J-6B: The Lightweight Champ First up, we have GPT-J-6B, the plucky underdog of the LLM world. Don't let its relatively small size fool you – this 6 billion parameter model packs a punch. from transformers import GPTJForCausalLM, AutoTokenizer model = GPTJForCausalLM.from_pretrained("EleutherAI/gpt-j-6B") tokenizer = AutoTokenizer.from_pretrained("EleutherAI/gpt-j-6B") # Now you're ready to generate some text! Pro tip: GPT-J-6B is perfect for those "I just want to dip my toes in the LLM waters" moments. It's like the gateway drug of local LLMs. 2. BLOOM: The Multilingual Marvel BLOOM is the polyglot of our bunch. It speaks 46 languages and can code in 13 programming languages. It's like that annoying friend who always shows off their language skills at parties, except BLOOM is actually useful. from transformers import BloomForCausalLM, AutoTokenizer model = BloomForCausalLM.from_pretrained("bigscience/bloom") tokenizer = AutoTokenizer.from_pretrained("bigscience/bloom") # Bonjour! Hola! Kon'nichiwa! BLOOM's got you covered. 3. OPT: Meta's Gift to the Masses OPT, or Open Pretrained Transformer, is Meta's way of saying "We're not just about collecting your data, we can give some back too!" It comes in various sizes, from the cute 125M to the beastly 175B version. from transformers import AutoModelForCausalLM, AutoTokenizer model = AutoModelForCausalLM.from_pretrained("facebook/opt-1.3b") tokenizer = AutoTokenizer.from_pretrained("facebook/opt-1.3b") # Now you're ready to... poke? 4. FLAN-T5: Google's Swiss Army Knife FLAN-T5 is like that overachiever in class who's good at everything. It can translate, summarize, answer questions, and probably do your taxes if you ask nicely. from transformers import AutoModelForSeq2SeqLM, AutoTokenizer model = AutoModelForSeq2SeqLM.from_pretrained("google/flan-t5-base") tokenizer = AutoTokenizer.from_pretrained("google/flan-t5-base") # Prepare for some serious multitasking 5. BERT: The OG Transformer No list would be complete without BERT. It's the grandfather of modern NLP, the one that started it all. Running BERT locally is like keeping a piece of AI history on your machine. from transformers import BertForMaskedLM, AutoTokenizer model = BertForMaskedLM.from_pretrained("bert-base-uncased") tokenizer = AutoTokenizer.from_pretrained("bert-base-uncased") # Time to mask some words and watch BERT work its magic 6. RoBERTa: BERT's Overachieving Sibling If BERT is the reliable family sedan, RoBERTa is the souped-up sports car version. Same basic structure, but with more training and optimizations. from transformers import RobertaForMaskedLM, AutoTokenizer model = RobertaForMaskedLM.from_pretrained("roberta-base") tokenizer = AutoTokenizer.from_pretrained("roberta-base") # Vroom vroom! RoBERTa's ready to race 7. DistilBERT: The Diet Coke of BERTs DistilBERT is for when you want BERT's capabilities but your poor laptop is already sweating. It's 40% smaller and 60% faster, but still retains 97% of BERT's language understanding capabilities. from transformers import DistilBertForMaskedLM, AutoTokenizer model = DistilBertForMaskedLM.from_pretrained("distilbert-base-uncased") tokenizer = AutoTokenizer.from_pretrained("distilbert-base-uncased") # Light, refreshing, and still packs a punch 8. XLNet: The Attention Seeker XLNet is all about that permutation-based training. It's like if BERT went to a yoga retreat and came back all flexible and zen. from transformers import XLNetLMHeadModel, AutoTokenizer model = XLNetLMHeadModel.from_pretrained("xlnet-base-cased") tokenizer = AutoTokenizer.from_pretrained("xlnet-base-cased") # Prepare for some serious permutation action 9. ALBERT: The Efficient Einstein ALBERT stands for "A Lite BERT," and it lives up to its name. It's a slimmed-down version of BERT
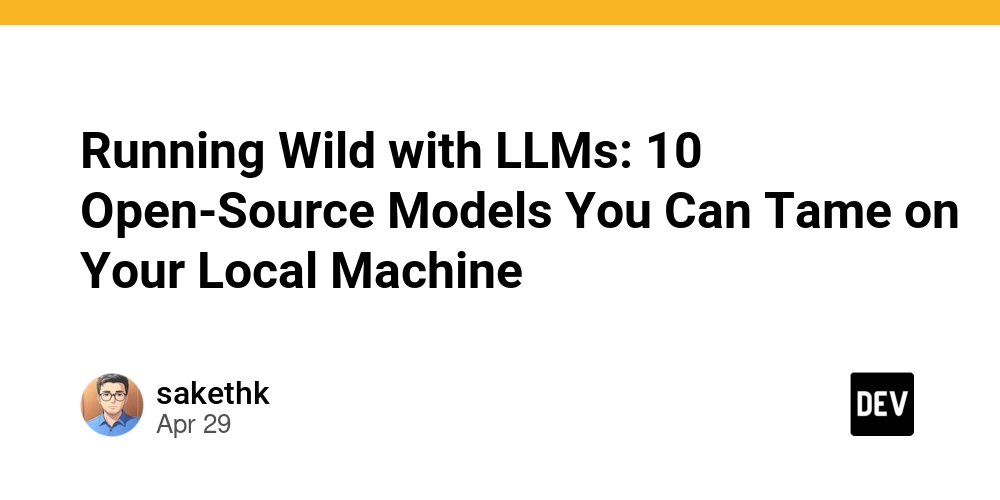
The AI Revolution in Your Pajamas
Hey there, fellow code wranglers and AI enthusiasts! Remember when running a language model meant renting out half of AWS and selling a kidney? Well, grab your favorite caffeinated beverage because those days are gone. We're diving into the wonderful world of open-source LLMs you can run on your trusty local machine. No cloud required, pants optional.
Why Local LLMs? Because Sometimes, You Just Want to be Alone with Your AI
Before we jump into our list, let's talk about why you'd want to run an LLM locally:
- Privacy: Your conversations stay between you and your computer. No eavesdropping clouds here!
- Offline access: Internet down? No problem. Your AI buddy is always there for you.
- Customization: Tweak and train to your heart's content. Make an LLM that truly gets your obscure Star Trek references.
- Cost-effective: Save those cloud computing dollars for something more important. Like coffee. Or more RAM.
Alright, let's meet our contestants!
1. GPT-J-6B: The Lightweight Champ
First up, we have GPT-J-6B, the plucky underdog of the LLM world. Don't let its relatively small size fool you – this 6 billion parameter model packs a punch.
from transformers import GPTJForCausalLM, AutoTokenizer
model = GPTJForCausalLM.from_pretrained("EleutherAI/gpt-j-6B")
tokenizer = AutoTokenizer.from_pretrained("EleutherAI/gpt-j-6B")
# Now you're ready to generate some text!
Pro tip: GPT-J-6B is perfect for those "I just want to dip my toes in the LLM waters" moments. It's like the gateway drug of local LLMs.
2. BLOOM: The Multilingual Marvel
BLOOM is the polyglot of our bunch. It speaks 46 languages and can code in 13 programming languages. It's like that annoying friend who always shows off their language skills at parties, except BLOOM is actually useful.
from transformers import BloomForCausalLM, AutoTokenizer
model = BloomForCausalLM.from_pretrained("bigscience/bloom")
tokenizer = AutoTokenizer.from_pretrained("bigscience/bloom")
# Bonjour! Hola! Kon'nichiwa! BLOOM's got you covered.
3. OPT: Meta's Gift to the Masses
OPT, or Open Pretrained Transformer, is Meta's way of saying "We're not just about collecting your data, we can give some back too!" It comes in various sizes, from the cute 125M to the beastly 175B version.
from transformers import AutoModelForCausalLM, AutoTokenizer
model = AutoModelForCausalLM.from_pretrained("facebook/opt-1.3b")
tokenizer = AutoTokenizer.from_pretrained("facebook/opt-1.3b")
# Now you're ready to... poke?
4. FLAN-T5: Google's Swiss Army Knife
FLAN-T5 is like that overachiever in class who's good at everything. It can translate, summarize, answer questions, and probably do your taxes if you ask nicely.
from transformers import AutoModelForSeq2SeqLM, AutoTokenizer
model = AutoModelForSeq2SeqLM.from_pretrained("google/flan-t5-base")
tokenizer = AutoTokenizer.from_pretrained("google/flan-t5-base")
# Prepare for some serious multitasking
5. BERT: The OG Transformer
No list would be complete without BERT. It's the grandfather of modern NLP, the one that started it all. Running BERT locally is like keeping a piece of AI history on your machine.
from transformers import BertForMaskedLM, AutoTokenizer
model = BertForMaskedLM.from_pretrained("bert-base-uncased")
tokenizer = AutoTokenizer.from_pretrained("bert-base-uncased")
# Time to mask some words and watch BERT work its magic
6. RoBERTa: BERT's Overachieving Sibling
If BERT is the reliable family sedan, RoBERTa is the souped-up sports car version. Same basic structure, but with more training and optimizations.
from transformers import RobertaForMaskedLM, AutoTokenizer
model = RobertaForMaskedLM.from_pretrained("roberta-base")
tokenizer = AutoTokenizer.from_pretrained("roberta-base")
# Vroom vroom! RoBERTa's ready to race
7. DistilBERT: The Diet Coke of BERTs
DistilBERT is for when you want BERT's capabilities but your poor laptop is already sweating. It's 40% smaller and 60% faster, but still retains 97% of BERT's language understanding capabilities.
from transformers import DistilBertForMaskedLM, AutoTokenizer
model = DistilBertForMaskedLM.from_pretrained("distilbert-base-uncased")
tokenizer = AutoTokenizer.from_pretrained("distilbert-base-uncased")
# Light, refreshing, and still packs a punch
8. XLNet: The Attention Seeker
XLNet is all about that permutation-based training. It's like if BERT went to a yoga retreat and came back all flexible and zen.
from transformers import XLNetLMHeadModel, AutoTokenizer
model = XLNetLMHeadModel.from_pretrained("xlnet-base-cased")
tokenizer = AutoTokenizer.from_pretrained("xlnet-base-cased")
# Prepare for some serious permutation action
9. ALBERT: The Efficient Einstein
ALBERT stands for "A Lite BERT," and it lives up to its name. It's a slimmed-down version of BERT that still performs incredibly well on language understanding tasks.
from transformers import AlbertForMaskedLM, AutoTokenizer
model = AlbertForMaskedLM.from_pretrained("albert-base-v2")
tokenizer = AutoTokenizer.from_pretrained("albert-base-v2")
# E = mc² (Efficiency = model compression²)
10. GPT-Neo: The Open-Source GPT
Last but not least, we have GPT-Neo, the open-source alternative to GPT-3. It's like the home-brewed craft beer of the LLM world – artisanal, open-source, and with a hint of digital hoppiness.
from transformers import GPTNeoForCausalLM, AutoTokenizer
model = GPTNeoForCausalLM.from_pretrained("EleutherAI/gpt-neo-1.3B")
tokenizer = AutoTokenizer.from_pretrained("EleutherAI/gpt-neo-1.3B")
# Time to generate some neo-classical text
Wrapping Up: Your Local AI Adventure Awaits
There you have it, folks – ten open-source LLMs you can run on your local machine faster than you can say "Hey, where's the cloud?" From the lightweight champs to the heavyweight contenders, there's an LLM for every need and every machine.
Remember, running these models locally is not just about having AI at your fingertips. It's about learning, experimenting, and maybe impressing your cat with your machine's newfound eloquence. So go ahead, download a model, fire up your Python environment, and let the AI shenanigans begin!
Just one last piece of advice: if your computer starts making strange noises or emitting smoke while running these models, it's probably not achieving sentience. It's just telling you it's time for an upgrade. Or a fire extinguisher. Possibly both.
Happy local LLM-ing, and may your VRAM be ever in your favor!
If you enjoyed this dive into the local LLM pool, follow me for more AI adventures and bad puns. I promise my next post will have 50% more references to obscure 80s movies and 100% fewer mentions of selling kidneys. Unless you're into that sort of thing. No judgment here!