RL for Multimodal Label Ranking: A Novel Approach
This is a Plain English Papers summary of a research paper called RL for Multimodal Label Ranking: A Novel Approach. If you like these kinds of analysis, you should join AImodels.fyi or follow us on Twitter. Overview This paper explores a novel approach to multimodal label relevance ranking by employing reinforcement learning. The research addresses the challenge of effectively ranking labels based on their relevance to multimodal inputs, such as images and text. The authors propose a reinforcement learning framework to learn an optimal ranking policy for labels, considering the complex interplay between different modalities. The method aims to improve the accuracy and efficiency of label ranking in multimodal contexts, potentially enhancing applications like image retrieval and content recommendation. This work contributes to the growing field of multimodal learning and offers a new perspective on label ranking using advanced machine learning techniques. Plain English Explanation Imagine you have a system that looks at pictures and reads text, and you want it to pick the most relevant labels or tags for what it's seeing and reading. This is multimodal label relevance ranking. For example, if you show it a picture of a cat playing with yarn and the ... Click here to read the full summary of this paper
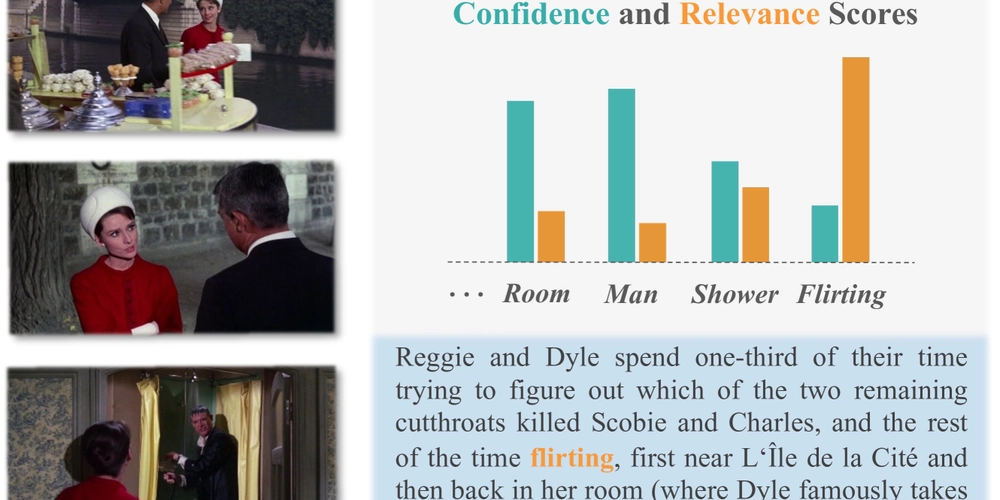
This is a Plain English Papers summary of a research paper called RL for Multimodal Label Ranking: A Novel Approach. If you like these kinds of analysis, you should join AImodels.fyi or follow us on Twitter.
Overview
- This paper explores a novel approach to multimodal label relevance ranking by employing reinforcement learning.
- The research addresses the challenge of effectively ranking labels based on their relevance to multimodal inputs, such as images and text.
- The authors propose a reinforcement learning framework to learn an optimal ranking policy for labels, considering the complex interplay between different modalities.
- The method aims to improve the accuracy and efficiency of label ranking in multimodal contexts, potentially enhancing applications like image retrieval and content recommendation.
- This work contributes to the growing field of multimodal learning and offers a new perspective on label ranking using advanced machine learning techniques.
Plain English Explanation
Imagine you have a system that looks at pictures and reads text, and you want it to pick the most relevant labels or tags for what it's seeing and reading. This is multimodal label relevance ranking. For example, if you show it a picture of a cat playing with yarn and the ...