Red Hat Expands AI Capabilities Across Hybrid Cloud and OpenShift
Originally published at ssojet Red Hat has recently announced upgrades to its AI portfolio, which are designed to enhance the development and deployment of AI solutions across hybrid cloud environments. This includes a focus on integrating AI into enterprise operations to support both predictive and generative AI models. Red Hat OpenShift AI is a central platform in these enhancements, providing tools for managing AI lifecycles across hybrid clouds. This platform supports machine learning operations (MLOps) and large language model operations (LLMOps), facilitating the building, tuning, and deployment of AI models. Key features include: Distributed Serving: Enabled through the vLLM inference server, this feature allows model serving across multiple GPUs, improving resource efficiency and training speed. For further details, visit Red Hat OpenShift AI. Model Evaluation: Utilizing the language model evaluation (lm-eval) component, data scientists can benchmark their models across various tasks, ensuring optimal AI performance. AI Guardrails: A technology preview feature that enhances the accuracy and performance of models by monitoring user interactions and outputs, helping to prevent unwanted content. The enhancements in Red Hat Enterprise Linux AI (RHEL AI) also include support for generative AI models, enabling users to develop, test, and run models tailored to specific business needs. More information can be found on the Red Hat RHEL AI page. Llama 4 Herd Integration with OpenShift AI On April 7, 2025, Meta released its latest Llama model family, Llama 4, which is now fully compatible with Red Hat OpenShift AI and vLLM. This collaboration between Red Hat and Meta allows users to leverage Llama 4 in their OpenShift AI environments seamlessly. The Llama 4 models, including Scout and Maverick, utilize a mixture of experts (MoE) architecture for improved computational efficiency. Key specifications include: Llama 4 Scout: 17 billion active parameters, capable of processing up to 10 million tokens, ideal for tasks like multi-document summarization. Llama 4 Maverick: 17 billion active parameters with 400 billion total parameters, suitable for sophisticated AI applications across various languages. To deploy Llama 4 in OpenShift AI, users need an OpenShift Cluster with OpenShift AI (Version 2.13 or above) and adequate system resources. Detailed instructions can be found in Red Hat's deployment guide. Expanding AI Capabilities with KServe and Other Integrations Red Hat has broadened its AI capabilities by introducing KServe, a Kubernetes custom resource definition that allows orchestration of multiple AI models. This expansion is part of Red Hat's strategy to streamline AI workload deployments on its OpenShift platform. Additional enhancements include: Support for deploying inference engines for large language models (LLMs) with vLLM. Integration with NVIDIA's NIM microservices framework for enhanced AI model management. New partnerships with cloud providers to simplify deployments across different environments. For more information on these developments, you can visit the Cloud Native Now article. No-Cost AI Foundations Training Red Hat is committed to supporting organizations in their AI journey by offering no-cost online training courses. These courses are designed for both experienced leaders and novices, providing valuable insights on how to leverage AI for business transformation. The training is available through the Red Hat AI Foundations page. Organizations looking to implement secure authentication solutions can explore SSOJet's API-first platform. SSOJet offers features such as directory sync, SAML, OIDC, and magic link authentication, enabling enterprises to manage single sign-on (SSO) and user management effectively. Visit SSOJet for more details.
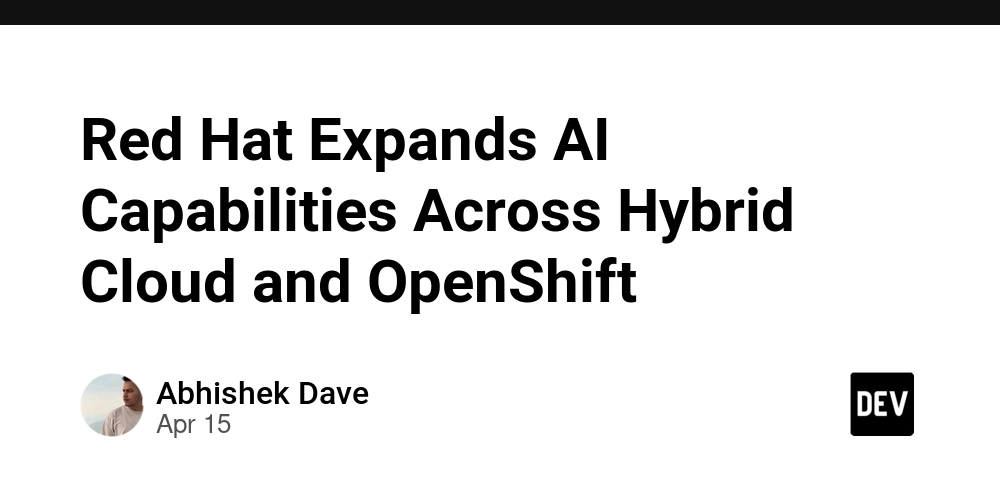
Originally published at ssojet
Red Hat has recently announced upgrades to its AI portfolio, which are designed to enhance the development and deployment of AI solutions across hybrid cloud environments. This includes a focus on integrating AI into enterprise operations to support both predictive and generative AI models. Red Hat OpenShift AI is a central platform in these enhancements, providing tools for managing AI lifecycles across hybrid clouds. This platform supports machine learning operations (MLOps) and large language model operations (LLMOps), facilitating the building, tuning, and deployment of AI models.
Key features include:
- Distributed Serving: Enabled through the vLLM inference server, this feature allows model serving across multiple GPUs, improving resource efficiency and training speed. For further details, visit Red Hat OpenShift AI.
- Model Evaluation: Utilizing the language model evaluation (lm-eval) component, data scientists can benchmark their models across various tasks, ensuring optimal AI performance.
- AI Guardrails: A technology preview feature that enhances the accuracy and performance of models by monitoring user interactions and outputs, helping to prevent unwanted content.
The enhancements in Red Hat Enterprise Linux AI (RHEL AI) also include support for generative AI models, enabling users to develop, test, and run models tailored to specific business needs. More information can be found on the Red Hat RHEL AI page.
Llama 4 Herd Integration with OpenShift AI
On April 7, 2025, Meta released its latest Llama model family, Llama 4, which is now fully compatible with Red Hat OpenShift AI and vLLM. This collaboration between Red Hat and Meta allows users to leverage Llama 4 in their OpenShift AI environments seamlessly.
The Llama 4 models, including Scout and Maverick, utilize a mixture of experts (MoE) architecture for improved computational efficiency. Key specifications include:
Llama 4 Scout: 17 billion active parameters, capable of processing up to 10 million tokens, ideal for tasks like multi-document summarization.
Llama 4 Maverick: 17 billion active parameters with 400 billion total parameters, suitable for sophisticated AI applications across various languages.
To deploy Llama 4 in OpenShift AI, users need an OpenShift Cluster with OpenShift AI (Version 2.13 or above) and adequate system resources. Detailed instructions can be found in Red Hat's deployment guide.
Expanding AI Capabilities with KServe and Other Integrations
Red Hat has broadened its AI capabilities by introducing KServe, a Kubernetes custom resource definition that allows orchestration of multiple AI models. This expansion is part of Red Hat's strategy to streamline AI workload deployments on its OpenShift platform.
Additional enhancements include:
- Support for deploying inference engines for large language models (LLMs) with vLLM.
- Integration with NVIDIA's NIM microservices framework for enhanced AI model management.
- New partnerships with cloud providers to simplify deployments across different environments.
For more information on these developments, you can visit the Cloud Native Now article.
No-Cost AI Foundations Training
Red Hat is committed to supporting organizations in their AI journey by offering no-cost online training courses. These courses are designed for both experienced leaders and novices, providing valuable insights on how to leverage AI for business transformation. The training is available through the Red Hat AI Foundations page.
Organizations looking to implement secure authentication solutions can explore SSOJet's API-first platform. SSOJet offers features such as directory sync, SAML, OIDC, and magic link authentication, enabling enterprises to manage single sign-on (SSO) and user management effectively. Visit SSOJet for more details.