Document Q&A with RAG: Google Gen AI Capstone Project
As part of the Google Generative AI Intensive Course, the final task was to experiment with Gen AI using the tools and techniques presented throughout the program. For my project, I chose a Document Q&A system using Retrieval-Augmented Generation (RAG) and themed it around one of my hobbies: the Call of Cthulhu RPG. The notebook answers the question about the game rules as described in the Call of Cthulhu 7th Edition Quick-Start Rules guide available on Chaosium's website. Some example queries the system handles: Is there magic in the game? What do I need to bring for the game What is STR? While large language models (LLMs) are very powerful, they have two key limitations: They can’t “know” anything beyond their training data — so they don’t have access to private, recent, or domain-specific documents unless you explicitly give them that information. They have limited context windows — meaning they can only handle a certain amount of text at once, which limits how much you can “feed” them per query. Retrieval-Augmented Generation (RAG) RAG is a technique that addresses both of these limitations. Instead of trying to cram all the necessary information into a single prompt or hoping the model already knows the answer, RAG combines the power of retrieval systems with language generation. Here’s how it works, broken into three stages: 1. Indexing (Preprocessing) Before anything can be retrieved, your documents need to be processed and stored in a way that enables fast, intelligent searching. This involves: Splitting documents into smaller chunks (like paragraphs or sections), Creating vector embeddings for each chunk—numerical representations that capture the meaning of the text, Storing these embeddings in a vector database for quick lookup. 2. Retrieval (Query-Time Search) When a user asks a question, the system: Converts the question into an embedding, Searches the vector database to find the most semantically similar chunks, Returns these top matches as the most relevant context. 3. Generation (LLM Response) The retrieved document chunks, the user’s original question, and any specific instructions are passed to an LLM, which then generates a natural language response grounded in the retrieved context. This allows the system to provide answers even about topics the LLM has never seen during training. Vector Databases One of the most important parts of a RAG system is the vector database, such as ChromaDB, an open-source embedding store designed for fast, scalable retrieval. Vector databases store embeddings, which allow you to search for semantic meaning, not just keyword matches. This makes them a great match for document Q&A applications. Links You can explore the full project notebook on Kaggle here. Source of the material used for the article/notebook: Google Generative AI Intensive Course This article was created with the help of AI - for better readability.
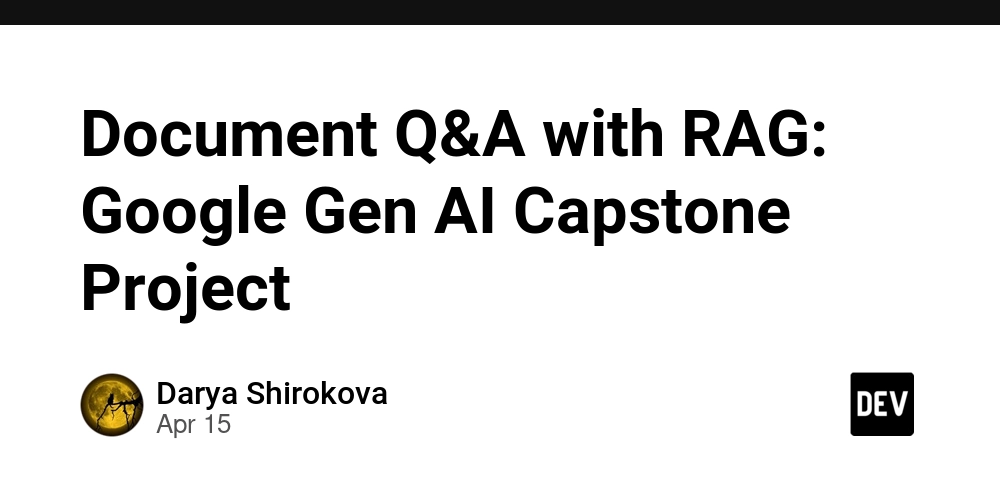
As part of the Google Generative AI Intensive Course, the final task was to experiment with Gen AI using the tools and techniques presented throughout the program.
For my project, I chose a Document Q&A system using Retrieval-Augmented Generation (RAG) and themed it around one of my hobbies: the Call of Cthulhu RPG. The notebook answers the question about the game rules as described in the Call of Cthulhu 7th Edition Quick-Start Rules guide available on Chaosium's website.
Some example queries the system handles:
- Is there magic in the game?
- What do I need to bring for the game
- What is STR?
While large language models (LLMs) are very powerful, they have two key limitations:
- They can’t “know” anything beyond their training data — so they don’t have access to private, recent, or domain-specific documents unless you explicitly give them that information.
- They have limited context windows — meaning they can only handle a certain amount of text at once, which limits how much you can “feed” them per query.
Retrieval-Augmented Generation (RAG)
RAG is a technique that addresses both of these limitations. Instead of trying to cram all the necessary information into a single prompt or hoping the model already knows the answer, RAG combines the power of retrieval systems with language generation.
Here’s how it works, broken into three stages:
1. Indexing (Preprocessing)
Before anything can be retrieved, your documents need to be processed and stored in a way that enables fast, intelligent searching. This involves:
- Splitting documents into smaller chunks (like paragraphs or sections),
- Creating vector embeddings for each chunk—numerical representations that capture the meaning of the text,
- Storing these embeddings in a vector database for quick lookup.
2. Retrieval (Query-Time Search)
When a user asks a question, the system:
- Converts the question into an embedding,
- Searches the vector database to find the most semantically similar chunks,
- Returns these top matches as the most relevant context.
3. Generation (LLM Response)
The retrieved document chunks, the user’s original question, and any specific instructions are passed to an LLM, which then generates a natural language response grounded in the retrieved context.
This allows the system to provide answers even about topics the LLM has never seen during training.
Vector Databases
One of the most important parts of a RAG system is the vector database, such as ChromaDB, an open-source embedding store designed for fast, scalable retrieval.
Vector databases store embeddings, which allow you to search for semantic meaning, not just keyword matches. This makes them a great match for document Q&A applications.
Links
You can explore the full project notebook on Kaggle here.
Source of the material used for the article/notebook: Google Generative AI Intensive Course
This article was created with the help of AI - for better readability.