Real-Time AI Image Captioning with Node.js and servbay
Real-Time AI Image Captioning with Node.js and servbay In the rapidly advancing world of artificial intelligence, one of the most fascinating capabilities is image captioning—the ability for machines to interpret and describe images in natural language. When combined with real-time processing, this technology becomes incredibly powerful, unlocking new possibilities in accessibility, surveillance, content management, and more. In this blog, we will dive deep into building a real-time AI image captioning system using Node.js and servbay. What is Real-Time AI Image Captioning? Image captioning is the process of generating a textual description for an image using deep learning models. Real-time captioning means this process happens on-the-fly, immediately after an image is received, with minimal delay. This involves: Extracting features from the image using a pre-trained Convolutional Neural Network (CNN) like ResNet or Inception. Passing the features to a Recurrent Neural Network (RNN) or Transformer-based model (like GPT or BERT derivatives) to generate a coherent caption. In real-time scenarios, we need to handle: Fast image uploads or stream processing Efficient model inference Response delivery without noticeable latency Why Node.js and servbay? Node.js Node.js is a non-blocking, event-driven JavaScript runtime ideal for building scalable, I/O-heavy applications like real-time systems. It supports a wide range of packages that make it easy to integrate AI services. servbay @servbay is a modern server environment that allows you to run backend services quickly and efficiently. It provides a simplified, container-like local development environment with features such as: Hot reload Easy configuration for multiple services Simplified deployment and isolation System Architecture Overview Here’s a high-level architecture of our image captioning system: Frontend: A web app where users upload images. Backend (Node.js): Handles file uploads Sends images to the AI model (hosted locally or via an API) Returns captions to the client in real-time AI Model Service: Loads a pre-trained model (like BLIP, CLIP+GPT2, or a Hugging Face pipeline) Accepts images and returns captions servbay Configuration: Manages local services and dependencies Step-by-Step Implementation 1. Setup the Project Start by creating a new Node.js project and initialize your dependencies. mkdir realtime-captioning cd realtime-captioning npm init -y npm install express multer axios @servbay/cli 2. Setup @servbay Install and initialize @servbay: npm install -g @servbay/cli servbay init Create a servbay.yml configuration: services: ai-model: image: huggingface/transformers-pytorch-cpu ports: - "5000:5000" volumes: - ./model:/app Start your services: servbay up 3. Backend: Express Server with File Uploads Create a simple server with Express and multer to handle file uploads. const express = require('express'); const multer = require('multer'); const axios = require('axios'); const fs = require('fs'); const path = require('path'); const app = express(); const upload = multer({ dest: 'uploads/' }); app.post('/upload', upload.single('image'), async (req, res) => { const filePath = path.join(__dirname, req.file.path); const image = fs.readFileSync(filePath); try { const response = await axios.post('http://localhost:5000/caption', image, { headers: { 'Content-Type': 'application/octet-stream' } }); res.json({ caption: response.data.caption }); } catch (err) { console.error(err); res.status(500).send('Error generating caption'); } finally { fs.unlinkSync(filePath); } }); app.listen(3000, () => console.log('Server started on http://localhost:3000')); 4. AI Captioning Service (Python Example) Create a separate Python service using Flask and a Hugging Face model: from flask import Flask, request, jsonify from transformers import BlipProcessor, BlipForConditionalGeneration from PIL import Image import io app = Flask(__name__) processor = BlipProcessor.from_pretrained("Salesforce/blip-image-captioning-base") model = BlipForConditionalGeneration.from_pretrained("Salesforce/blip-image-captioning-base") @app.route('/caption', methods=['POST']) def caption(): image = Image.open(io.BytesIO(request.data)).convert('RGB') inputs = processor(image, return_tensors="pt") out = model.generate(**inputs) caption = processor.decode(out[0], skip_special_tokens=True) return jsonify({ 'caption': caption }) if __name__ == '__main__': app.run(host='0.0.0.0', port=5000) Add this to the model folder and use @servbay to run it in an isolated service. Frontend: Upload Interface You can use a simple HTML page or React frontend: Upload document.getE
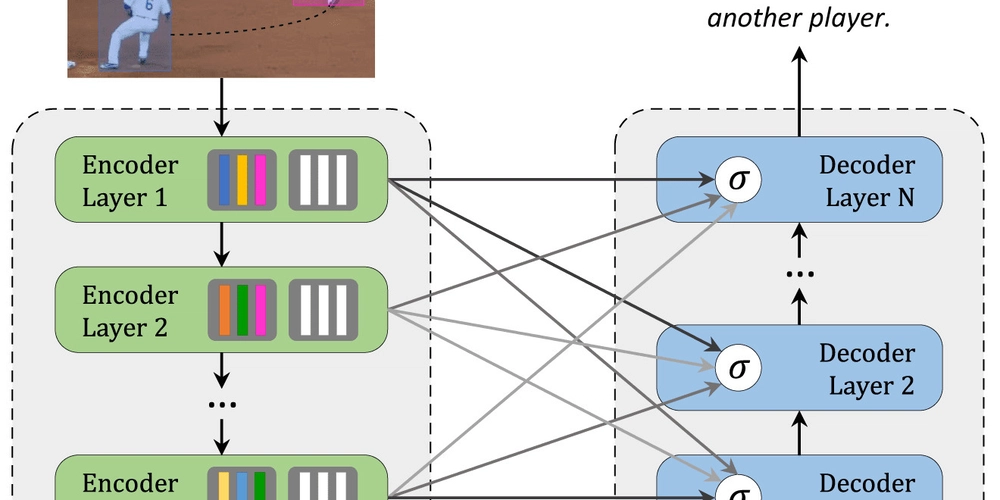
Real-Time AI Image Captioning with Node.js and servbay
In the rapidly advancing world of artificial intelligence, one of the most fascinating capabilities is image captioning—the ability for machines to interpret and describe images in natural language. When combined with real-time processing, this technology becomes incredibly powerful, unlocking new possibilities in accessibility, surveillance, content management, and more. In this blog, we will dive deep into building a real-time AI image captioning system using Node.js and servbay.
What is Real-Time AI Image Captioning?
Image captioning is the process of generating a textual description for an image using deep learning models. Real-time captioning means this process happens on-the-fly, immediately after an image is received, with minimal delay.
This involves:
- Extracting features from the image using a pre-trained Convolutional Neural Network (CNN) like ResNet or Inception.
- Passing the features to a Recurrent Neural Network (RNN) or Transformer-based model (like GPT or BERT derivatives) to generate a coherent caption.
In real-time scenarios, we need to handle:
- Fast image uploads or stream processing
- Efficient model inference
- Response delivery without noticeable latency
Why Node.js and servbay?
Node.js
Node.js is a non-blocking, event-driven JavaScript runtime ideal for building scalable, I/O-heavy applications like real-time systems. It supports a wide range of packages that make it easy to integrate AI services.
servbay
@servbay is a modern server environment that allows you to run backend services quickly and efficiently. It provides a simplified, container-like local development environment with features such as:
- Hot reload
- Easy configuration for multiple services
- Simplified deployment and isolation
System Architecture Overview
Here’s a high-level architecture of our image captioning system:
- Frontend: A web app where users upload images.
-
Backend (Node.js):
- Handles file uploads
- Sends images to the AI model (hosted locally or via an API)
- Returns captions to the client in real-time
-
AI Model Service:
- Loads a pre-trained model (like BLIP, CLIP+GPT2, or a Hugging Face pipeline)
- Accepts images and returns captions
- servbay Configuration: Manages local services and dependencies
Step-by-Step Implementation
1. Setup the Project
Start by creating a new Node.js project and initialize your dependencies.
mkdir realtime-captioning
cd realtime-captioning
npm init -y
npm install express multer axios @servbay/cli
2. Setup @servbay
Install and initialize @servbay:
npm install -g @servbay/cli
servbay init
Create a servbay.yml
configuration:
services:
ai-model:
image: huggingface/transformers-pytorch-cpu
ports:
- "5000:5000"
volumes:
- ./model:/app
Start your services:
servbay up
3. Backend: Express Server with File Uploads
Create a simple server with Express and multer
to handle file uploads.
const express = require('express');
const multer = require('multer');
const axios = require('axios');
const fs = require('fs');
const path = require('path');
const app = express();
const upload = multer({ dest: 'uploads/' });
app.post('/upload', upload.single('image'), async (req, res) => {
const filePath = path.join(__dirname, req.file.path);
const image = fs.readFileSync(filePath);
try {
const response = await axios.post('http://localhost:5000/caption', image, {
headers: {
'Content-Type': 'application/octet-stream'
}
});
res.json({ caption: response.data.caption });
} catch (err) {
console.error(err);
res.status(500).send('Error generating caption');
} finally {
fs.unlinkSync(filePath);
}
});
app.listen(3000, () => console.log('Server started on http://localhost:3000'));
4. AI Captioning Service (Python Example)
Create a separate Python service using Flask and a Hugging Face model:
from flask import Flask, request, jsonify
from transformers import BlipProcessor, BlipForConditionalGeneration
from PIL import Image
import io
app = Flask(__name__)
processor = BlipProcessor.from_pretrained("Salesforce/blip-image-captioning-base")
model = BlipForConditionalGeneration.from_pretrained("Salesforce/blip-image-captioning-base")
@app.route('/caption', methods=['POST'])
def caption():
image = Image.open(io.BytesIO(request.data)).convert('RGB')
inputs = processor(image, return_tensors="pt")
out = model.generate(**inputs)
caption = processor.decode(out[0], skip_special_tokens=True)
return jsonify({ 'caption': caption })
if __name__ == '__main__':
app.run(host='0.0.0.0', port=5000)
Add this to the model folder and use @servbay to run it in an isolated service.
Frontend: Upload Interface
You can use a simple HTML page or React frontend:
id="caption">
document.getElementById('upload-form').onsubmit = async (e) => {
e.preventDefault();
const formData = new FormData();
formData.append('image', document.getElementById('image').files[0]);
const response = await fetch('/upload', {
method: 'POST',
body: formData
});
const data = await response.json();
document.getElementById('caption').innerText = data.caption;
};
Performance Tips
- Use GPU-enabled containers if performance is critical
- Use streaming APIs for large files
- Batch requests where possible
- Optimize models using ONNX or TensorRT
Conclusion
Real-time AI image captioning opens up powerful use cases, from enhancing accessibility to managing digital content. With the combination of Node.js for handling asynchronous logic and @servbay for managing services, developers can rapidly prototype and deploy scalable solutions. By following this guide, you’ve taken a deep dive into building a real-time AI image captioning system that combines modern tools and technologies for efficient development and deployment.
AI isn’t just about data science anymore—it’s about smart, accessible engineering. Let your code speak as smartly as your machines do!