InsightFlow Part 7: Data Quality Implementation & Best Practices for InsightFlow
InsightFlow GitHub Repo In this post, we’ll explore how data quality was implemented in the InsightFlow project and share best practices for ensuring reliable and accurate data pipelines. Data quality is a critical aspect of any data engineering project, as it ensures that the insights derived from the data are trustworthy and actionable. Why Data Quality Matters Data quality directly impacts the reliability of analytics and decision-making. Poor data quality can lead to: Inaccurate Insights: Misleading trends and correlations. Operational Inefficiencies: Wasted time debugging and fixing issues downstream. Loss of Trust: Stakeholders losing confidence in the data. For InsightFlow, ensuring data quality was essential to accurately analyze retail sales trends and their correlation with fuel prices. Data Quality Framework for InsightFlow The data quality framework for InsightFlow was implemented at multiple stages of the pipeline, from ingestion to transformation and analysis. Below are the key components: 1. Data Validation During Ingestion The ingestion layer, implemented using AWS Batch, includes basic validation checks to ensure the raw data meets expected formats and structures. Validation Steps File Format Validation: Ensures that ingested files are in the expected format (e.g., Parquet or CSV). Schema Validation: Confirms that the files contain the required columns with the correct data types. Null Checks: Flags missing or null values in critical columns. Example: Python Validation Script def validate_data(df, required_columns): missing_columns = [col for col in required_columns if col not in df.columns] if missing_columns: raise ValueError(f"Missing required columns: {missing_columns}") if df.isnull().any().any(): raise ValueError("Null values detected in the dataset.") 2. Data Quality in Transformation (dbt) The transformation layer, implemented using dbt, includes robust data quality checks through schema tests and custom tests. Schema Tests Schema tests ensure that the data adheres to predefined rules. For example: Not Null: Ensures critical columns (e.g., sales_value_rm_mil) are not null. Unique: Ensures unique values in primary key columns (e.g., date_key). Relationships: Validates foreign key relationships between fact and dimension tables. Example: Schema Test for fct_retail_sales_monthly models: - name: fct_retail_sales_monthly description: "Monthly fact table combining retail sales data and average fuel prices." columns: - name: date_key description: "Foreign key to the date dimension." tests: - not_null - relationships: to: ref('dim_date') field: date_key - name: sales_value_rm_mil description: "Monthly sales value in RM millions." tests: - not_null - dbt_utils.expression_is_true: expression: "sales_value_rm_mil >= 0" Custom Tests Custom tests were implemented using the dbt-utils package to validate business-specific rules. For example: Price Range Validation: Ensures fuel prices are within a reasonable range. Volume Index Validation: Ensures volume indices are non-negative. 3. Monitoring and Alerts To ensure ongoing data quality, monitoring and alerting mechanisms were implemented using CloudWatch and Kestra. CloudWatch Metrics Glue Crawler Logs: Monitors schema changes and ingestion errors. Athena Query Logs: Tracks query performance and errors. Kestra Workflow Alerts Kestra workflows include error handling and notifications for failed tasks. For example: If a Glue Crawler fails, an alert is sent to the team via email or Slack. If a dbt test fails, the pipeline halts, and the issue is logged for debugging. Best Practices for Data Quality 1. Define Clear Data Quality Rules Collaborate with stakeholders to define rules for each dataset (e.g., required columns, valid ranges). Document these rules in a central repository for easy reference. 2. Automate Data Quality Checks Use tools like dbt to automate schema and custom tests. Integrate validation scripts into the ingestion pipeline. 3. Monitor Data Quality Continuously Set up dashboards to monitor key metrics (e.g., null values, schema changes). Use alerts to notify the team of issues in real time. 4. Handle Data Quality Issues Proactively Implement retry mechanisms for transient errors (e.g., network issues during ingestion). Log all data quality issues for auditing and debugging. 5. Test Data Quality Regularly Schedule regular tests to ensure data quality rules are enforced. Use historical data to validate new rules and identify anomalies. Challenges and Lessons Learned Schema Evo
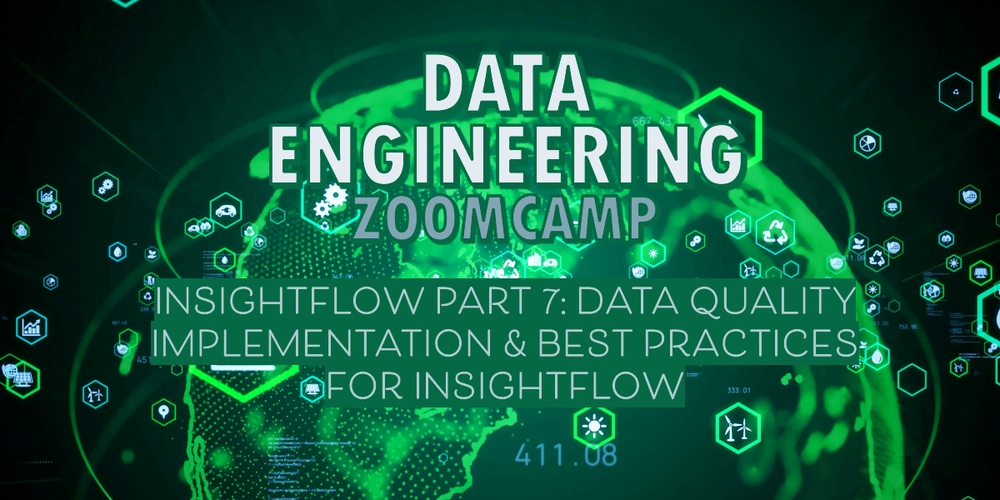
In this post, we’ll explore how data quality was implemented in the InsightFlow project and share best practices for ensuring reliable and accurate data pipelines. Data quality is a critical aspect of any data engineering project, as it ensures that the insights derived from the data are trustworthy and actionable.
Why Data Quality Matters
Data quality directly impacts the reliability of analytics and decision-making. Poor data quality can lead to:
- Inaccurate Insights: Misleading trends and correlations.
- Operational Inefficiencies: Wasted time debugging and fixing issues downstream.
- Loss of Trust: Stakeholders losing confidence in the data.
For InsightFlow, ensuring data quality was essential to accurately analyze retail sales trends and their correlation with fuel prices.
Data Quality Framework for InsightFlow
The data quality framework for InsightFlow was implemented at multiple stages of the pipeline, from ingestion to transformation and analysis. Below are the key components:
1. Data Validation During Ingestion
The ingestion layer, implemented using AWS Batch, includes basic validation checks to ensure the raw data meets expected formats and structures.
Validation Steps
- File Format Validation: Ensures that ingested files are in the expected format (e.g., Parquet or CSV).
- Schema Validation: Confirms that the files contain the required columns with the correct data types.
- Null Checks: Flags missing or null values in critical columns.
Example: Python Validation Script
def validate_data(df, required_columns):
missing_columns = [col for col in required_columns if col not in df.columns]
if missing_columns:
raise ValueError(f"Missing required columns: {missing_columns}")
if df.isnull().any().any():
raise ValueError("Null values detected in the dataset.")
2. Data Quality in Transformation (dbt)
The transformation layer, implemented using dbt, includes robust data quality checks through schema tests and custom tests.
Schema Tests
Schema tests ensure that the data adheres to predefined rules. For example:
-
Not Null: Ensures critical columns (e.g.,
sales_value_rm_mil
) are not null. -
Unique: Ensures unique values in primary key columns (e.g.,
date_key
). - Relationships: Validates foreign key relationships between fact and dimension tables.
Example: Schema Test for fct_retail_sales_monthly
models:
- name: fct_retail_sales_monthly
description: "Monthly fact table combining retail sales data and average fuel prices."
columns:
- name: date_key
description: "Foreign key to the date dimension."
tests:
- not_null
- relationships:
to: ref('dim_date')
field: date_key
- name: sales_value_rm_mil
description: "Monthly sales value in RM millions."
tests:
- not_null
- dbt_utils.expression_is_true:
expression: "sales_value_rm_mil >= 0"
Custom Tests
Custom tests were implemented using the dbt-utils
package to validate business-specific rules. For example:
- Price Range Validation: Ensures fuel prices are within a reasonable range.
- Volume Index Validation: Ensures volume indices are non-negative.
3. Monitoring and Alerts
To ensure ongoing data quality, monitoring and alerting mechanisms were implemented using CloudWatch and Kestra.
CloudWatch Metrics
- Glue Crawler Logs: Monitors schema changes and ingestion errors.
- Athena Query Logs: Tracks query performance and errors.
Kestra Workflow Alerts
Kestra workflows include error handling and notifications for failed tasks. For example:
- If a Glue Crawler fails, an alert is sent to the team via email or Slack.
- If a dbt test fails, the pipeline halts, and the issue is logged for debugging.
Best Practices for Data Quality
1. Define Clear Data Quality Rules
- Collaborate with stakeholders to define rules for each dataset (e.g., required columns, valid ranges).
- Document these rules in a central repository for easy reference.
2. Automate Data Quality Checks
- Use tools like dbt to automate schema and custom tests.
- Integrate validation scripts into the ingestion pipeline.
3. Monitor Data Quality Continuously
- Set up dashboards to monitor key metrics (e.g., null values, schema changes).
- Use alerts to notify the team of issues in real time.
4. Handle Data Quality Issues Proactively
- Implement retry mechanisms for transient errors (e.g., network issues during ingestion).
- Log all data quality issues for auditing and debugging.
5. Test Data Quality Regularly
- Schedule regular tests to ensure data quality rules are enforced.
- Use historical data to validate new rules and identify anomalies.
Challenges and Lessons Learned
-
Schema Evolution: Managing schema changes in Glue required careful configuration of the
schema_change_policy
. - Custom Tests: Writing custom tests for business-specific rules required collaboration with domain experts.
- Alert Fatigue: Fine-tuning alerts was necessary to avoid overwhelming the team with non-critical notifications.
Conclusion
Implementing robust data quality practices is essential for building reliable data pipelines. By integrating validation checks, schema tests, and monitoring mechanisms, InsightFlow ensures that its data is accurate, consistent, and trustworthy. These practices not only improve the quality of insights but also build confidence among stakeholders.