How to simplify the Merge Operations Across Tables from Multiple Databases
Due to business requirements, data is stored by year in two structurally identical databases. To perform statistical analysis on this data, it will involve cross-database computation. Implementing this directly through databases or hardcoding is cumbersome. esProc simplifies such operations. Data Structure of orders table: Where order_id is the primary key. Sample data: 10001 16 2024-12-14 116 Product116 9 12.84 115.56 Credit Card 984 Example St, City 2 Pending 2025-04-23 01:21:08 2025-04-23 01:21:08 10002 11 2024-08-18 116 Product116 5 25.71 128.55 PayPal 841 Example St, City 1 Shipped 2025-04-23 01:21:08 2025-04-23 01:21:08 10003 20 2024-08-08 109 Product109 2 13.23 26.46 PayPal 676 Example St, City 4 Pending 2025-04-23 01:21:08 2025-04-23 01:21:08 10127 7 2024-10-12 113 Product113 4 20.64 82.56 Cash 145 Example St, City 4 Delivered 2025-04-23 01:21:08 2025-04-23 01:21:08 10190 19 2024-06-02 110 Product110 7 88.55 619.85 PayPal 289 Example St, City 2 Pending 2025-04-23 01:21:08 2025-04-23 01:21:08 10001 16 2024-12-14 116 Product116 9 12.84 115.56 Credit Card 984 Example St, City 2 Pending 2025-04-23 01:21:08 2025-04-23 01:21:08 10002 11 2024-08-18 116 Product116 5 25.71 128.55 PayPal 841 Example St, City 1 Shipped 2025-04-23 01:21:08 2025-04-23 01:21:08 10003 20 2024-08-08 109 Product109 2 13.23 26.46 PayPal 676 Example St, City 4 Pending 2025-04-23 01:21:08 2025-04-23 01:21:08 10127 7 2024-10-12 113 Product113 4 20.64 82.56 Cash 145 Example St, City 4 Delivered 2025-04-23 01:21:08 2025-04-23 01:21:08 10190 19 2024-06-02 110 Product110 7 88.55 619.85 PayPal 289 Example St, City 2 Pending 2025-04-23 01:21:08 2025-04-23 01:21:08 Now, we want to merge and calculate data from the two databases. How can we do this using esProc? Installing esProc First, download esProc Standard Edition ,esProc is free. After installation, configure a connection to the MySQL database. Begin by placing the MySQL JDBC driver package in the directory [esProc installation directory]\common\jdbc (similar setup for other databases). Then, start the esProc IDE. From the menu bar, select Tool > Connect to Data Source and configure a standard MySQL JDBC connection. Configure the data source dbb of the bytedbb database in the same way. Test the connection by clicking Connect. The connection is successful if the two data sources just configured turn pink. Test the configuration by executing the script with Ctrl+F9. If the data is queried successfully, the configuration is correct. Cross-database computations Let’s now merge and calculate the data from the two tables. A2 and A4 query the orders data from the two databases, respectively. The @x option indicates that the connection will be closed after the query. A5 merges the data from A2 and A4 using the | symbol – it’s that simple! A6 then performs subsequent calculations on the merged data (specifically, grouping and aggregation). Clicking on a cell (such as A5, which contains the merged data) displays the calculation result for that step. However, we find that the two databases contain duplicate data. We need to deduplicate before calculating. A6 uses group@1 to group by order_id and retain only the first record from each group, thus removing duplicates. To retain records based on a specific condition (e.g., the most recent record), sort first and then use group@1 – it’s very flexible. Checking the result of A6 reveals that the duplicate data with IDs 10001, 10002, etc., previously present in both tables, has been removed. A7 then performs grouping and aggregation. Subsequent calculations remain the same as for a single table. To perform other computations, simply change the calculation expression. Being able to perform cross-database computations also makes it easy to handle data comparison tasks. For example, search for orders present in both databases, or orders present in only one database. Here, A5 performs a full join using join. A6 filters for duplicate orders (intersection), while A7 and A8 filter for unique orders (set difference), respectively. A9 filters for records with duplicate order_id values but differing values in other columns. To simplify the code, macros are used. The expression ${A2.fname().("~(1)."/~/"!=~(2)."/~).concat("||"), when expanded, becomes: ~(1).quantity != ~(2).quantity || ~(1).unit_price != ~(2).unit_price || ~(1).total_amount != ~(2).total_amount || ~(1).order_status != ~(2).order_status. The comparison results can be observed after execution: Big data scenarios If the data volume is too large to be loaded entirely into memory, we need to use esProc’s cursor mechanism to perform cross-database computations. If duplicates are not a concern, simply merge the two cursors and compute: A2 and A4 use the cursor function to query data. A5 merges the two cursors, and A6 performs the calcul
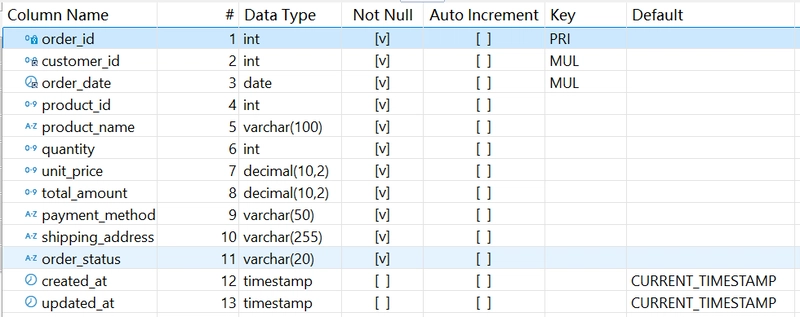
Due to business requirements, data is stored by year in two structurally identical databases. To perform statistical analysis on this data, it will involve cross-database computation. Implementing this directly through databases or hardcoding is cumbersome. esProc simplifies such operations.
Data
Structure of orders table:
Where order_id is the primary key.
Sample data:
10001 16 2024-12-14 116 Product116 9 12.84 115.56 Credit Card 984 Example St, City 2 Pending 2025-04-23 01:21:08 2025-04-23 01:21:08
10002 11 2024-08-18 116 Product116 5 25.71 128.55 PayPal 841 Example St, City 1 Shipped 2025-04-23 01:21:08 2025-04-23 01:21:08
10003 20 2024-08-08 109 Product109 2 13.23 26.46 PayPal 676 Example St, City 4 Pending 2025-04-23 01:21:08 2025-04-23 01:21:08
10127 7 2024-10-12 113 Product113 4 20.64 82.56 Cash 145 Example St, City 4 Delivered 2025-04-23 01:21:08 2025-04-23 01:21:08
10190 19 2024-06-02 110 Product110 7 88.55 619.85 PayPal 289 Example St, City 2 Pending 2025-04-23 01:21:08 2025-04-23 01:21:08
10001 16 2024-12-14 116 Product116 9 12.84 115.56 Credit Card 984 Example St, City 2 Pending 2025-04-23 01:21:08 2025-04-23 01:21:08
10002 11 2024-08-18 116 Product116 5 25.71 128.55 PayPal 841 Example St, City 1 Shipped 2025-04-23 01:21:08 2025-04-23 01:21:08
10003 20 2024-08-08 109 Product109 2 13.23 26.46 PayPal 676 Example St, City 4 Pending 2025-04-23 01:21:08 2025-04-23 01:21:08
10127 7 2024-10-12 113 Product113 4 20.64 82.56 Cash 145 Example St, City 4 Delivered 2025-04-23 01:21:08 2025-04-23 01:21:08
10190 19 2024-06-02 110 Product110 7 88.55 619.85 PayPal 289 Example St, City 2 Pending 2025-04-23 01:21:08 2025-04-23 01:21:08
Now, we want to merge and calculate data from the two databases. How can we do this using esProc?
Installing esProc
First, download esProc Standard Edition ,esProc is free.
After installation, configure a connection to the MySQL database.
Begin by placing the MySQL JDBC driver package in the directory [esProc installation directory]\common\jdbc (similar setup for other databases).
Then, start the esProc IDE. From the menu bar, select Tool > Connect to Data Source and configure a standard MySQL JDBC connection.
Configure the data source dbb of the bytedbb database in the same way. Test the connection by clicking Connect. The connection is successful if the two data sources just configured turn pink.
Test the configuration by executing the script with Ctrl+F9. If the data is queried successfully, the configuration is correct.
Cross-database computations
Let’s now merge and calculate the data from the two tables.
A2 and A4 query the orders data from the two databases, respectively. The @x option indicates that the connection will be closed after the query. A5 merges the data from A2 and A4 using the | symbol – it’s that simple! A6 then performs subsequent calculations on the merged data (specifically, grouping and aggregation).
Clicking on a cell (such as A5, which contains the merged data) displays the calculation result for that step.
However, we find that the two databases contain duplicate data. We need to deduplicate before calculating.
A6 uses group@1 to group by order_id and retain only the first record from each group, thus removing duplicates. To retain records based on a specific condition (e.g., the most recent record), sort first and then use group@1 – it’s very flexible.
Checking the result of A6 reveals that the duplicate data with IDs 10001, 10002, etc., previously present in both tables, has been removed. A7 then performs grouping and aggregation.
Subsequent calculations remain the same as for a single table. To perform other computations, simply change the calculation expression.
Being able to perform cross-database computations also makes it easy to handle data comparison tasks. For example, search for orders present in both databases, or orders present in only one database.
Here, A5 performs a full join using join. A6 filters for duplicate orders (intersection), while A7 and A8 filter for unique orders (set difference), respectively. A9 filters for records with duplicate order_id values but differing values in other columns. To simplify the code, macros are used. The expression ${A2.fname().("~(1)."/~/"!=~(2)."/~).concat("||"), when expanded, becomes: ~(1).quantity != ~(2).quantity || ~(1).unit_price != ~(2).unit_price || ~(1).total_amount != ~(2).total_amount || ~(1).order_status != ~(2).order_status.
The comparison results can be observed after execution:
Big data scenarios
If the data volume is too large to be loaded entirely into memory, we need to use esProc’s cursor mechanism to perform cross-database computations.
If duplicates are not a concern, simply merge the two cursors and compute:
A2 and A4 use the cursor function to query data. A5 merges the two cursors, and A6 performs the calculations. Overall, the process is similar to that of in-memory calculations.
Execute the script. A5 returns a cursor object. To view the contents, click ‘Load data’:
If deduplication is required, the cursors must be ordered to facilitate comparison of adjacent data. Therefore, sorting by order_id in the SQL query is necessary.
Merging ordered cursors requires using the CS.merge() function. merge provides many options. @u indicates a union operation, so it directly deduplicates. There are also @i for intersection and @d for difference. Subsequent calculations are all the same.
The merge operation still returns a cursor (no actual calculation is performed at this stage):
The calculation and result retrieval only begin at the final grouping and aggregation stage.
Overall, the calculation process for big data is fundamentally the same as in-memory processing, effectively lowering the barrier to entry.
Big data comparison is also supported:
Because A6-A10 return cursors, result set functions need to be added to B7-B10 to execute the calculations and retrieve the results.
However, strangely, only B7 has results, while B8-B10 are empty.
This is because cursors are single-use: once fully traversed, they are consumed, and further calculations become impossible. To address this, we utilize esProc’s cursor reuse (channel) mechanism, enabling multiple calculations to be completed in a single traversal for big data scenarios. Let’s modify the code:
A7-A10 create channels based on the cursor in A5 (A8-A10 use abbreviated notation). The remaining operations in B7-C10 are identical to those described above. After execution, the computed results will be displayed in cells A7-A10 (Important: the results are in A7-A10, not C7-C10).
Should the computed results also be too large to be loaded entirely into memory, the results can be output to files. Let’s modify the code again:
Add the output filenames to the fetch functions in C7-C10.
After execution, these result files will be generated.
With esProc, cross-database calculations are handled with ease.
esProc scripts can be easily embedded in Java applications. For details on deployment and integration procedures, please consult the documentation on the official website.