How Pandas Simplifies ETL Data Cleaning
When discussing the "T" in the ETL (Extract, Transform, Load) process, a huge part of the work involves cleaning the data and ensuring it is ready for analysis. If you're a data engineer, you've probably stared down the barrel of messy spreadsheets more times than you can count, columns riddled with null values, dates being formatted every which way, inconsistent text, and those delightful outliers that make no sense. Our job is to wrangle this chaos into clean, reliable data pipelines. In this article, we will see how Pandas, a data manipulation library written in Python, can help address these challenges and simplify the data cleaning process in the context of ETL pipelines. We will be using a real-world example of banking data stored in a CSV file to see exactly how Pandas deals with common data issues and converts messy, raw information into a format that works perfectly for analysis. What is Pandas? Pandas is a Python library for data manipulation and analysis. It acts like a Swiss Army Knife for data wrangling providing versatile tools to handle messy data of any size and suitable for both quick analyses and complex data pipelines. The library's foundation rests on two primary data structures: DataFrame: A 2D, table-like structure (akin to a spreadsheet) for holding labeled, structured data. Series: A 1D labeled array, effectively a single column from a DataFrame. This ability to efficiently and intuitively address real-world data challenges is what makes Pandas so powerful. Common Hurdles in Data Quality Data entering the ETL processes is often dirty and failure to identify and correct common issues can seriously undermine analytics and machine learning models, leading to flawed insights. Before exploring Pandas' solutions, let's recognize these typical problems: Missing Data: Records may be incomplete (null or blank values) due to collection errors or omissions. Inconsistent Formatting: Data representation can vary widely (e.g., different date styles, mixed types in one column, inconsistent labels). Duplication: Redundant entries of the same information can bloat datasets and skew analysis. Outliers: Atypical, extreme values may distort statistical summaries and model performance. Incorrect Data Types: Some Columns might contain unexpected data types. A good example is a text data type appearing where numbers are expected. Addressing these challenges is a critical step, highlighting the need for robust data cleaning tools like Pandas. Data Cleaning with Pandas Pandas equips data engineers with a robust toolkit, making the often tedious task of data cleaning significantly easier. Its functions streamline handling missing values, standardizing formats, integrating data sources, and more. Key capabilities include: Addressing Missing Values: Use functions like isna() to detect nulls, fillna() to impute them (with mean, median, mode, etc.), or dropna() to remove them. Transforming and Standardizing Data: Easily rename columns, apply custom functions (apply(), map()), perform string manipulations, and ensure consistent formatting across datasets. Combining Data Sources: Integrate data from various tables or files using high-performance merging (merge(), join()) and concatenation (concat()) operations. Eliminating Redundancy: Identify and remove duplicate rows efficiently with drop_duplicates() to maintain data uniqueness. Correcting Data Types: Convert columns to their appropriate types (numeric, string, boolean, datetime) using astype() or specialized converters like to_datetime(). These features allow for systematic and efficient data preparation within ETL pipelines Real-World Example: Cleaning Banking Marketing Data Let’s take a look at a real-world example of how Pandas can help clean data. Imagine you’re working with data from a recent marketing campaign for a bank, where the goal is to identify potential clients for personal loans. The raw data likely contains common issues: missing values, inconsistent text formats (like 'yes'/'no' variations), incorrect data types, and maybe some outliers. These need fixing before the data can be reliably used for analysis or loaded into a data lake or a data warehouse Here’s a breakdown of the types of data involved and typical cleaning tasks: Client Data: Contains details like job type, marital status, education level, and mortgage status. Cleaning might involve: Replacing inconsistent text (e.g., standardizing job or education entries containing '.') with consistent values. Handling missing values (e.g., replacing 'unknown' education levels with NaN - Not a Number). Converting 'yes'/'no' fields (like credit_default or mortgage) into Boolean (True/False) types. Campaign Data: Contains information on contact history, such as the number of contacts, duration, outcomes of previous campaigns, and last contact date. Cleaning often requires: Converting outcome descriptions (e.g., 'succ
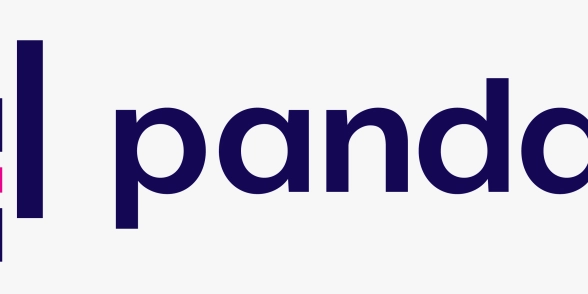
When discussing the "T" in the ETL (Extract, Transform, Load) process, a huge part of the work involves cleaning the data and ensuring it is ready for analysis.
If you're a data engineer, you've probably stared down the barrel of messy spreadsheets more times than you can count, columns riddled with null values, dates being formatted every which way, inconsistent text, and those delightful outliers that make no sense. Our job is to wrangle this chaos into clean, reliable data pipelines.
In this article, we will see how Pandas, a data manipulation library written in Python, can help address these challenges and simplify the data cleaning process in the context of ETL pipelines.
We will be using a real-world example of banking data stored in a CSV file to see exactly how Pandas deals with common data issues and converts messy, raw information into a format that works perfectly for analysis.
What is Pandas?
Pandas is a Python library for data manipulation and analysis. It acts like a Swiss Army Knife for data wrangling providing versatile tools to handle messy data of any size and suitable for both quick analyses and complex data pipelines. The library's foundation rests on two primary data structures:
DataFrame: A 2D, table-like structure (akin to a spreadsheet) for holding labeled, structured data.
Series: A 1D labeled array, effectively a single column from a DataFrame.
This ability to efficiently and intuitively address real-world data challenges is what makes Pandas so powerful.
Common Hurdles in Data Quality
Data entering the ETL processes is often dirty and failure to identify and correct common issues can seriously undermine analytics and machine learning models, leading to flawed insights. Before exploring Pandas' solutions, let's recognize these typical problems:
Missing Data: Records may be incomplete (null or blank values) due to collection errors or omissions.
Inconsistent Formatting: Data representation can vary widely (e.g., different date styles, mixed types in one column, inconsistent labels).
Duplication: Redundant entries of the same information can bloat datasets and skew analysis.
Outliers: Atypical, extreme values may distort statistical summaries and model performance.
Incorrect Data Types: Some Columns might contain unexpected data types. A good example is a text data type appearing where numbers are expected.
Addressing these challenges is a critical step, highlighting the need for robust data cleaning tools like Pandas.
Data Cleaning with Pandas
Pandas equips data engineers with a robust toolkit, making the often tedious task of data cleaning significantly easier. Its functions streamline handling missing values, standardizing formats, integrating data sources, and more. Key capabilities include:
Addressing Missing Values: Use functions like
isna()
to detect nulls,fillna()
to impute them (with mean, median, mode, etc.), ordropna()
to remove them.Transforming and Standardizing Data: Easily rename columns, apply custom functions (
apply()
,map()
), perform string manipulations, and ensure consistent formatting across datasets.Combining Data Sources: Integrate data from various tables or files using high-performance merging (
merge()
,join()
) and concatenation (concat()
) operations.Eliminating Redundancy: Identify and remove duplicate rows efficiently with
drop_duplicates()
to maintain data uniqueness.Correcting Data Types: Convert columns to their appropriate types (numeric, string, boolean, datetime) using
astype()
or specialized converters liketo_datetime()
.
These features allow for systematic and efficient data preparation within ETL pipelines
Real-World Example: Cleaning Banking Marketing Data
Let’s take a look at a real-world example of how Pandas can help clean data. Imagine you’re working with data from a recent marketing campaign for a bank, where the goal is to identify potential clients for personal loans.
The raw data likely contains common issues: missing values, inconsistent text formats (like 'yes'/'no'
variations), incorrect data types, and maybe some outliers. These need fixing before the data can be reliably used for analysis or loaded into a data lake or a data warehouse
Here’s a breakdown of the types of data involved and typical cleaning tasks:
-
Client Data: Contains details like job type, marital status, education level, and mortgage status. Cleaning might involve:
- Replacing inconsistent text (e.g., standardizing job or education entries containing '.') with consistent values.
- Handling missing values (e.g., replacing 'unknown' education levels with
NaN
- Not a Number). - Converting
'yes'/'no'
fields (likecredit_default
ormortgage
) into Boolean (True/False
) types.
-
Campaign Data: Contains information on contact history, such as the number of contacts, duration, outcomes of previous campaigns, and last contact date. Cleaning often requires:
- Converting outcome descriptions (e.g., 'success', 'failure', 'nonexistent') into Boolean or numerical flags.
- Parsing date components (like day and month) into a standard datetime format.
-
Economic Indicators: Contextual data like consumer price index or interest rates (
EURIBOR
). Cleaning typically focuses on:- Ensuring these columns have the correct numerical data type.
- Addressing any missing numerical values appropriately (e.g., filling or removing).
Here’s a code snippet illustrating how Pandas tackles some of these cleaning steps:
import pandas as pd
import numpy as np # Often needed for handling NaN
# Let's pretend we loaded the messy data
# df = pd.read_csv('bank_marketing_messy.csv') # Placeholder for loading
# --- Client Data Cleaning ---
client = df[['client_id', 'age', 'job', 'marital', 'education', 'credit_default', 'mortgage']].copy() # Use .copy() to avoid SettingWithCopyWarning
# Clean up text inconsistencies (e.g., '.' shouldn't be in job/education)
client['job'] = client['job'].str.replace('.', '_', regex=False)
client['education'] = client['education'].str.replace('.', '_', regex=False)
# Handle 'unknown' consistently - replace with NaN for better handling later
client['education'] = client['education'].replace('unknown', np.NaN)
# Convert yes/no/unknown to more usable types (e.g., boolean or numerical)
# Assuming 'unknown' in credit_default means 'no' for this example
client['credit_default'] = client['credit_default'].map({'yes': True, 'no': False, 'unknown': False}).astype(bool)
client['mortgage'] = client['mortgage'].map({'yes': True, 'no': False, 'unknown': False}).astype(bool) # Assuming 'unknown' means False here too
# --- Campaign Data Cleaning ---
campaign = df[['client_id', 'number_contacts', 'contact_duration', 'previous_campaign_contacts', 'previous_outcome', 'campaign_outcome', 'day', 'month']].copy()
# Map outcomes to boolean (or 1/0)
campaign['previous_outcome'] = campaign['previous_outcome'].map({'success': True, 'failure': False, 'nonexistent': False}).astype(bool)
campaign['campaign_outcome'] = campaign['campaign_outcome'].map({'yes': True, 'no': False}).astype(bool)
# Create a proper datetime column from day/month (assuming year 2022 for context)
# Note: This requires careful handling of potential errors/formats
try:
# Build a date string: '5 May 2022'
date_str = df['day'].astype(str) + ' ' + df['month'].str.capitalize() + ' ' + '2022'
campaign['last_contact_date'] = pd.to_datetime(date_str, format='%d %b %Y', errors='coerce') # errors='coerce' turns bad dates into NaT (Not a Time)
except Exception as e:
print(f"Date conversion error: {e}")
# Handle error - maybe create an empty date column or log issues
campaign['last_contact_date'] = pd.NaT
# Drop original day/month columns if no longer needed
campaign = campaign.drop(columns=['day', 'month'])
# --- Economics Data Cleaning ---
economics = df[['client_id', 'cons_price_idx', 'euribor_three_months']].copy()
# Main task here is often ensuring correct numeric type and handling NaNs
# Example: fill missing economic indicators with the mean/median if appropriate
economics['euribor_three_months'] = economics['euribor_three_months'].fillna(economics['euribor_three_months'].median())
# Ensure data types are correct (they often load as object/string if NaNs were present)
economics['cons_price_idx'] = pd.to_numeric(economics['cons_price_idx'], errors='coerce')
economics['euribor_three_months'] = pd.to_numeric(economics['euribor_three_months'], errors='coerce')
# Now you'd save these cleaned dataframes
client.to_csv('client_cleaned.csv', index=False)
campaign.to_csv('campaign_cleaned.csv', index=False)
economics.to_csv('economics_cleaned.csv', index=False)
print("Data cleaning steps applied (example).")
Why Pandas is a Valuable Tool for Data Engineers
For data engineers, tools that offer efficiency and maintainability are crucial. Pandas provides several advantages:
Efficient Data Handling: It's designed for good performance on in-memory datasets, allowing for rapid cleaning and manipulation often faster than iterating manually or using overly complex SQL for transformations.
Readable and Reusable Code: The straightforward syntax makes writing, understanding, and maintaining data cleaning scripts easier.
These scripts can often be adapted for different projects or integrated into automated data pipelines.
- Strong Integration: Pandas works well with other core Python libraries (like NumPy, Scikit-learn) and data sources/sinks (databases, CSV, Parquet, JSON), facilitating its use in comprehensive data processing workflows.
Conclusion
Ultimately, Pandas is a fundamental part of a data engineer's toolkit. Whether dealing with marketing data like the example above or preparing datasets for other purposes, Pandas helps simplify and standardize the transformation ('T') stage of ETL.
Using (replaced "Leveraging") its powerful features saves time, reduces potential errors, and ensures data is correctly structured for loading and subsequent analysis."
Acknowledgements
The real-world banking marketing data cleaning example discussed in this article draws inspiration from the practical exercises and datasets provided in DataCamp's Data Engineering track.