Best Cloud Platforms for Data Engineering in 2025
As data engineering continues to evolve, cloud platforms play a crucial role in enabling scalable, efficient, and cost-effective data pipelines. With advancements in AI, real-time processing, and serverless computing, choosing the right cloud platform is more important than ever. In 2025, these are the top cloud platforms for data engineering, based on performance, flexibility, and innovation. 1. Google Cloud Platform (GCP) Best for: BigQuery, AI/ML Integration, and Real-Time Analytics Google Cloud remains a leader in data engineering, thanks to its powerful managed services: BigQuery: A serverless, highly scalable data warehouse with built-in ML capabilities. Dataflow: Fully managed Apache Beam-based data processing for batch and streaming. Dataproc: Managed Spark and Hadoop clusters with autoscaling. Vertex AI: Simplifies MLOps and generative AI integrations. Why GCP in 2025? BigQuery ML allows SQL-based machine learning, reducing the need for complex pipelines. Real-time analytics with Pub/Sub and Dataflow is unmatched. Sustainability focus: Google’s carbon-neutral cloud aligns with green data engineering trends. 2. Amazon Web Services (AWS) Best for: Scalability, Enterprise Adoption, and Serverless Data Pipelines AWS Database services continues to dominate with its vast ecosystem: Amazon Redshift: Improved performance with AQUA (Advanced Query Accelerator). AWS Glue: Serverless ETL with enhanced AI-driven data cataloging. Kinesis: Real-time data streaming at massive scale. EMR (Elastic MapReduce): Now supports Spark, Flink, and Ray for AI workloads. Why AWS in 2025? Largest market share with deep enterprise integrations. Bedrock & SageMaker streamline generative AI and ML workflows. Cost optimization with AWS Graviton processors reducing compute expenses. 3. Microsoft Azure Best for: Hybrid Cloud, Synapse Analytics, and Microsoft Ecosystem Azure is a top choice for enterprises using Microsoft products: Azure Synapse Analytics: Unified analytics with SQL, Spark, and data lake integration. Azure Data Factory: Low-code/no-code ETL with AI-powered mapping. Fabric: A new SaaS offering combining Power BI, Data Lake, and AI in one platform. HDInsight & Databricks: Optimized for big data and AI workloads. Why Azure in 2025? Deep Microsoft integration (Office 365, Power BI, Dynamics). Fabric reduces data silos by combining BI, analytics, and data engineering. Hybrid cloud support for enterprises with on-premises dependencies. 4. Snowflake Best for: Multi-Cloud Data Warehousing and Zero-Copy Cloning Though not a traditional cloud provider, Snowflake’s Data Cloud is a game-changer: Near-unlimited scalability with separation of storage and compute. Snowpark: Allows Python, Java, and Scala for advanced data engineering. Native integrations with AWS, Azure, and GCP. Why Snowflake in 2025? Multi-cloud flexibility without vendor lock-in. AI Data Cloud partnerships with NVIDIA and OpenAI. Cost-efficient with per-second billing and auto-suspend. 5. Databricks Lakehouse Platform Best for: Unified Data & AI, Delta Lake, and Open-Source Flexibility Databricks combines data lakes and warehouses with: Delta Lake: ACID transactions for big data. Unity Catalog: Unified governance across clouds. MLflow & AutoML: End-to-end MLOps integration. Why Databricks in 2025? Best for AI-driven data engineering with seamless PySpark and LLM support. Open architecture (Apache Spark, Python, SQL). Growing adoption in generative AI and real-time analytics. Final Thoughts: Which One to Choose? For AI & real-time analytics → GCP For enterprise scale & serverless → AWS For Microsoft-centric companies → Azure For multi-cloud data warehousing → Snowflake For AI/ML & open-source flexibility → Databricks In 2025, hybrid and multi-cloud strategies will dominate, so many organizations will leverage multiple platforms. The key is to align your choice with your data engineering needs, cost constraints, and AI roadmap. You can check more info about: Cloud Security in DevOps. DevOps Company. Platform Engineering Services. data integration challenges.
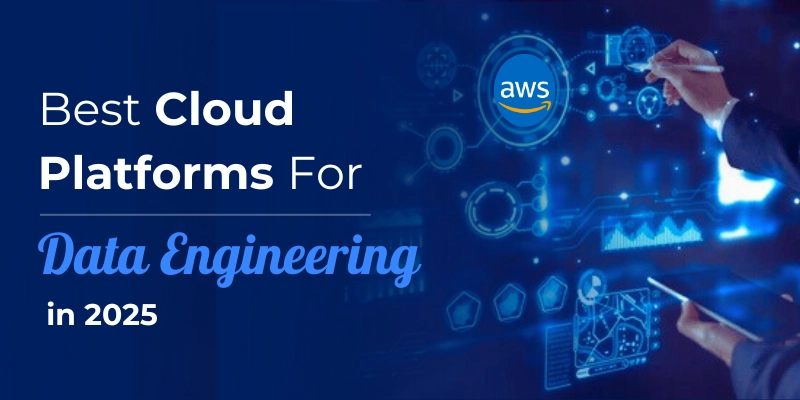
As data engineering continues to evolve, cloud platforms play a crucial role in enabling scalable, efficient, and cost-effective data pipelines. With advancements in AI, real-time processing, and serverless computing, choosing the right cloud platform is more important than ever.
In 2025, these are the top cloud platforms for data engineering, based on performance, flexibility, and innovation.
1. Google Cloud Platform (GCP)
Best for: BigQuery, AI/ML Integration, and Real-Time Analytics
Google Cloud remains a leader in data engineering, thanks to its powerful managed services:
BigQuery: A serverless, highly scalable data warehouse with built-in ML capabilities.
Dataflow: Fully managed Apache Beam-based data processing for batch and streaming.
Dataproc: Managed Spark and Hadoop clusters with autoscaling.
Vertex AI: Simplifies MLOps and generative AI integrations.
Why GCP in 2025?
BigQuery ML allows SQL-based machine learning, reducing the need for complex pipelines.
Real-time analytics with Pub/Sub and Dataflow is unmatched.
Sustainability focus: Google’s carbon-neutral cloud aligns with green data engineering trends.
2. Amazon Web Services (AWS)
Best for: Scalability, Enterprise Adoption, and Serverless Data Pipelines
AWS Database services continues to dominate with its vast ecosystem:
Amazon Redshift: Improved performance with AQUA (Advanced Query Accelerator).
AWS Glue: Serverless ETL with enhanced AI-driven data cataloging.
Kinesis: Real-time data streaming at massive scale.
EMR (Elastic MapReduce): Now supports Spark, Flink, and Ray for AI workloads.
Why AWS in 2025?
Largest market share with deep enterprise integrations.
Bedrock & SageMaker streamline generative AI and ML workflows.
Cost optimization with AWS Graviton processors reducing compute expenses.
3. Microsoft Azure
Best for: Hybrid Cloud, Synapse Analytics, and Microsoft Ecosystem
Azure is a top choice for enterprises using Microsoft products:
Azure Synapse Analytics: Unified analytics with SQL, Spark, and data lake integration.
Azure Data Factory: Low-code/no-code ETL with AI-powered mapping.
Fabric: A new SaaS offering combining Power BI, Data Lake, and AI in one platform.
HDInsight & Databricks: Optimized for big data and AI workloads.
Why Azure in 2025?
Deep Microsoft integration (Office 365, Power BI, Dynamics).
Fabric reduces data silos by combining BI, analytics, and data engineering.
Hybrid cloud support for enterprises with on-premises dependencies.
4. Snowflake
Best for: Multi-Cloud Data Warehousing and Zero-Copy Cloning
Though not a traditional cloud provider, Snowflake’s Data Cloud is a game-changer:
Near-unlimited scalability with separation of storage and compute.
Snowpark: Allows Python, Java, and Scala for advanced data engineering.
Native integrations with AWS, Azure, and GCP.
Why Snowflake in 2025?
Multi-cloud flexibility without vendor lock-in.
AI Data Cloud partnerships with NVIDIA and OpenAI.
Cost-efficient with per-second billing and auto-suspend.
5. Databricks Lakehouse Platform
Best for: Unified Data & AI, Delta Lake, and Open-Source Flexibility
Databricks combines data lakes and warehouses with:
Delta Lake: ACID transactions for big data.
Unity Catalog: Unified governance across clouds.
MLflow & AutoML: End-to-end MLOps integration.
Why Databricks in 2025?
Best for AI-driven data engineering with seamless PySpark and LLM support.
Open architecture (Apache Spark, Python, SQL).
Growing adoption in generative AI and real-time analytics.
Final Thoughts: Which One to Choose?
For AI & real-time analytics → GCP
For enterprise scale & serverless → AWS
For Microsoft-centric companies → Azure
For multi-cloud data warehousing → Snowflake
For AI/ML & open-source flexibility → Databricks
In 2025, hybrid and multi-cloud strategies will dominate, so many organizations will leverage multiple platforms. The key is to align your choice with your data engineering needs, cost constraints, and AI roadmap.
You can check more info about: Cloud Security in DevOps.