Agent-as-a-Service: A New Business Model for AI Agents Companies
Introduction As artificial intelligence continues to evolve, so too do the business models that power its widespread adoption. One of the most promising developments is the emergence of Agent-as-a-Service (AaaS)—a model where AI agents are deployed on demand to complete tasks, automate workflows, and provide intelligent assistance in real time. Much like Software-as-a-Service revolutionized enterprise software delivery, Agent-as-a-Service is reshaping how businesses access, integrate, and benefit from AI. AI agents are intelligent software entities capable of perceiving their environment, reasoning through data, and taking actions autonomously. The Agent-as-a-Service model takes these capabilities and packages them into scalable, subscription-based services that companies can use without building their own complex systems. This model has opened the door to a wide range of applications, from customer service and research automation to operations, logistics, and enterprise decision-making. This article explores how Agent-as-a-Service works, what makes it attractive to businesses, how AI agents companies are capitalizing on this trend, and what the future of this business model looks like. The Rise of Agent-as-a-Service The traditional deployment of AI agents required heavy upfront investment in infrastructure, custom development, and ongoing maintenance. While powerful, these systems were often out of reach for smaller companies or teams without deep technical expertise. Agent-as-a-Service simplifies that process. In this model, companies can subscribe to or call upon specialized AI agents hosted in the cloud. These agents can be tailored to specific roles—like research assistant, marketing strategist, data analyst, or customer support rep—and used as needed, without the burden of building and maintaining them in-house. This model is made possible by recent advances in cloud computing, API-first architecture, and large language models, which allow AI agents to be modular, adaptable, and accessible over standard interfaces. For companies looking to streamline operations or scale quickly, AaaS offers a low-risk, high-reward Deep learning entry point into intelligent automation. How Agent-as-a-Service Works Agent-as-a-Service providers typically offer AI agents through cloud-based platforms or APIs. Businesses can integrate these agents into their existing systems or use them via web interfaces. The agents are designed to autonomously perform a wide range of tasks, often with minimal human input. A typical AaaS setup includes: Agent Catalogs: A library of pre-configured AI agents, each designed for a specific use case or vertical. Customizable Workflows: Tools that allow businesses to customize how agents behave, what goals they pursue, and how they interact with internal data. Inter-agent Collaboration: The ability for multiple AI agents to work together, delegate tasks, and share information in real time. Monitoring and Analytics: Dashboards that track agent performance, task completion rates, and cost efficiency. Security and Compliance: Role-based access, audit trails, and data handling policies to ensure that agents operate safely within enterprise environments. These systems may be built using multi-agent frameworks or orchestration layers that enable dynamic planning, reasoning, and communication between AI agents. Use Cases Driving the AaaS Model Agent-as-a-Service is gaining popularity across a wide range of industries. Some prominent use cases include: Customer Support Automation AI agents can serve as intelligent virtual assistants, handling common customer inquiries, resolving issues, and even escalating cases when necessary. Unlike chatbots, these agents use reasoning and adaptive learning to respond with context and accuracy. Enterprise Research AaaS platforms can deploy research agents that scan databases, extract insights, summarize findings, and deliver reports to decision-makers. This helps organizations reduce research time and uncover insights that would otherwise be missed. Sales Enablement Sales agents can automate lead qualification, schedule meetings, write follow-up emails, and personalize outreach at scale. These agents increase productivity and allow sales teams to focus on closing deals. Operations and Logistics AI agents can optimize supply chains, manage inventories, predict demand, and assist in planning logistics. When agents collaborate across departments, they offer a holistic view that drives better operational decisions. Creative Assistance Marketing teams can use content generation agents to create social media posts, ad copy, and blog content. These agents are trained in brand tone, SEO practices, and campaign goals to produce relevant, high-quality output. Why Businesses Are Adopting Agent-as-a-Service There are several reasons why businesses are rapidly embracing this model: Cost Efficiency Building AI agents from scratch requires significant investment in talent,
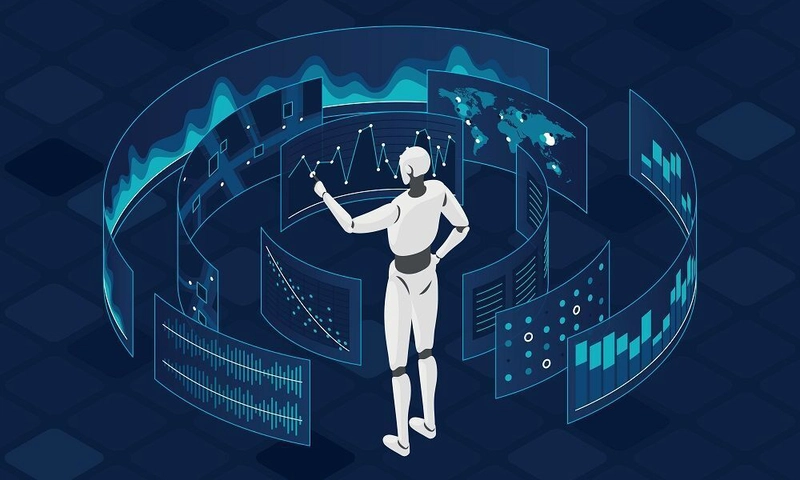
Introduction
As artificial intelligence continues to evolve, so too do the business models that power its widespread adoption. One of the most promising developments is the emergence of Agent-as-a-Service (AaaS)—a model where AI agents are deployed on demand to complete tasks, automate workflows, and provide intelligent assistance in real time. Much like Software-as-a-Service revolutionized enterprise software delivery, Agent-as-a-Service is reshaping how businesses access, integrate, and benefit from AI.
AI agents are intelligent software entities capable of perceiving their environment, reasoning through data, and taking actions autonomously. The Agent-as-a-Service model takes these capabilities and packages them into scalable, subscription-based services that companies can use without building their own complex systems. This model has opened the door to a wide range of applications, from customer service and research automation to operations, logistics, and enterprise decision-making.
This article explores how Agent-as-a-Service works, what makes it attractive to businesses, how AI agents companies are capitalizing on this trend, and what the future of this business model looks like.
The Rise of Agent-as-a-Service
The traditional deployment of AI agents required heavy upfront investment in infrastructure, custom development, and ongoing maintenance. While powerful, these systems were often out of reach for smaller companies or teams without deep technical expertise.
Agent-as-a-Service simplifies that process. In this model, companies can subscribe to or call upon specialized AI agents hosted in the cloud. These agents can be tailored to specific roles—like research assistant, marketing strategist, data analyst, or customer support rep—and used as needed, without the burden of building and maintaining them in-house.
This model is made possible by recent advances in cloud computing, API-first architecture, and large language models, which allow AI agents to be modular, adaptable, and accessible over standard interfaces. For companies looking to streamline operations or scale quickly, AaaS offers a low-risk, high-reward Deep learning entry point into intelligent automation.
How Agent-as-a-Service Works
Agent-as-a-Service providers typically offer AI agents through cloud-based platforms or APIs. Businesses can integrate these agents into their existing systems or use them via web interfaces. The agents are designed to autonomously perform a wide range of tasks, often with minimal human input.
A typical AaaS setup includes:
Agent Catalogs: A library of pre-configured AI agents, each designed for a specific use case or vertical.
Customizable Workflows: Tools that allow businesses to customize how agents behave, what goals they pursue, and how they interact with internal data.
Inter-agent Collaboration: The ability for multiple AI agents to work together, delegate tasks, and share information in real time.
Monitoring and Analytics: Dashboards that track agent performance, task completion rates, and cost efficiency.
Security and Compliance: Role-based access, audit trails, and data handling policies to ensure that agents operate safely within enterprise environments.
These systems may be built using multi-agent frameworks or orchestration layers that enable dynamic planning, reasoning, and communication between AI agents.
Use Cases Driving the AaaS Model
Agent-as-a-Service is gaining popularity across a wide range of industries. Some prominent use cases include:
- Customer Support Automation AI agents can serve as intelligent virtual assistants, handling common customer inquiries, resolving issues, and even escalating cases when necessary. Unlike chatbots, these agents use reasoning and adaptive learning to respond with context and accuracy.
- Enterprise Research AaaS platforms can deploy research agents that scan databases, extract insights, summarize findings, and deliver reports to decision-makers. This helps organizations reduce research time and uncover insights that would otherwise be missed.
- Sales Enablement Sales agents can automate lead qualification, schedule meetings, write follow-up emails, and personalize outreach at scale. These agents increase productivity and allow sales teams to focus on closing deals.
- Operations and Logistics AI agents can optimize supply chains, manage inventories, predict demand, and assist in planning logistics. When agents collaborate across departments, they offer a holistic view that drives better operational decisions.
- Creative Assistance Marketing teams can use content generation agents to create social media posts, ad copy, and blog content. These agents are trained in brand tone, SEO practices, and campaign goals to produce relevant, high-quality output. Why Businesses Are Adopting Agent-as-a-Service There are several reasons why businesses are rapidly embracing this model:
- Cost Efficiency Building AI agents from scratch requires significant investment in talent, infrastructure, and R&D. With AaaS, companies pay for what they use—whether that’s by time, task, or volume—making it more affordable and scalable.
- Speed of Deployment Instead of months-long AI initiatives, businesses can deploy AI agents within days or even hours. This speed allows them to test ideas, run pilot programs, and iterate quickly based on performance.
- Customization Without Complexity Modern AaaS platforms offer customization through low-code or no-code interfaces, allowing non-technical users to fine-tune agents for their needs. This democratizes AI and empowers business users to take ownership.
- Flexibility and Scale As demand fluctuates, companies can scale their use of AI agents up or down instantly. This agility is especially valuable in dynamic industries like e-commerce, media, and finance.
- Continuous Improvement Because AI agents are hosted in the cloud, service providers can roll out updates, fine-tune models, and improve performance continuously without client-side disruptions. The Business Opportunity for AI Agents Companies For companies building and selling AI agents, the AaaS model offers a recurring revenue stream, deeper customer relationships, and opportunities for innovation. Successful AI agents companies are focusing on a few core strategies: Vertical Specialization: Developing AI agents tailored to specific industries like healthcare, legal, or finance where domain knowledge enhances performance.
Platform Ecosystems: Creating ecosystems where businesses can mix and match agents, plug into existing tools, and build workflows with minimal friction.
White Labeling and OEM Models: Allowing other companies to rebrand or integrate AaaS capabilities into their own products and platforms.
Enterprise Integration: Building connectors and APIs that make it easy for clients to embed agents within existing CRMs, ERPs, and internal systems.
Data Privacy and Trust: Prioritizing privacy, security, and compliance to reassure enterprise clients and win long-term contracts.
This model not only unlocks new markets but also incentivizes continuous innovation in AI agents capabilities and performance.
Challenges in the AaaS Landscape
Despite its promise, the Agent-as-a-Service model faces certain challenges:
Interoperability: Ensuring agents can work seamlessly across different systems, platforms, and data formats.
Transparency: Many businesses want explainability in AI decision-making, which remains a work in progress for complex agents.
Performance Guarantees: Service-level agreements (SLAs) for intelligent agents can be hard to define and enforce.
Vendor Lock-in: Some platforms make it difficult for businesses to switch providers or take their data elsewhere.
Data Security: Enterprises are cautious about handing sensitive tasks to third-party AI agents, especially in regulated industries.
Conclusion
Agent-as-a-Service represents a major shift in how businesses consume artificial intelligence. By packaging AI agents into scalable, easy-to-use services, this model lowers the barrier to entry and opens up new possibilities for automation, decision-making, and customer engagement.
For companies building AI agents, AaaS is both a growth opportunity and a design challenge. It demands flexibility, specialization, and a relentless focus on user experience. For businesses adopting these services, the rewards include reduced costs, faster innovation, and the ability to stay competitive in a fast-changing landscape.
As the ecosystem matures, Agent-as-a-Service may become as foundational to business operations as cloud computing is today—powering a new generation of intelligent, collaborative, and highly efficient organizations.