When algorithms decide who gets a loan: The fraught fight to purge bias from AI
Artificial Intelligence (AI) has transformed numerous industries, including finance. One of its most significant applications in banking and credit services is in the decision-making process for loan approvals. AI-driven computers examine large amounts of financial data to assess a borrower’s creditworthiness, presumably making the process more efficient, accurate, and impartial. However, concerns regarding prejudice and discrimination in these algorithms generated extensive controversy. The dependence on machine learning (ML) models trained on historical data has, in numerous instances, maintained existing inequalities, disproportionately disadvantaging vulnerable communities.** *The Role of AI in Loan Decisions * Traditionally, banks and credit institutions depended on human underwriters to assess an applicant’s creditworthiness based on financial history, income, job position, and other characteristics. This approach was typically time-consuming and prone to human biases. AI has substantially expedited this procedure, evaluating complicated patterns in financial data within seconds to provide loan eligibility scores. Machine learning algorithms assess risk using enormous datasets, discovering tendencies that might not be immediately obvious to human analysts. By evaluating significant historical data, AI algorithms predict the possibility of a borrower defaulting on a loan. This automation reduces human involvement and is supposed to promote efficiency, minimise errors, and improve risk assessment. However, AI-based lending systems are not immune to bias despite these advantages. These algorithms inherit prejudices buried in past data, potentially leading to discriminatory lending practices that contribute to economic and social disparity. *A Problem with AI Loan Algorithms and Bias * To predict how the financial markets will behave in the future, AI algorithms examine historical data. The issue is that AI models can learn and perpetuate biases if the training data reflects past forms of discrimination, such as redlining, which systematically denied home loans to racial minorities. Numerous studies have shown that minority borrowers are disproportionately affected by the algorithms used by large financial institutions, leading to higher rejection rates and less favourable loan terms for these groups. Artificial intelligence lending models often include factors that can introduce bias. Indirect indications, such as ZIP codes, educational background, or employment history, might substitute for race, socioeconomic status, or gender even when algorithms don’t include explicit racial or gender data. Therefore, due to historical inequalities, AI models may unintentionally harm some groups. For instance, even after accounting for creditworthiness, a National Bureau of Economic Research study indicated that mortgage algorithms still charge Black and Hispanic borrowers greater interest rates than White borrowers. This provides more evidence that AI-driven lending algorithms are fundamentally biased. *The Effects of AI Discrimination on Access to Credit * Artificial intelligence (AI) bias in lending processes worsens already-existing income disparities. When AI models reject credit applications from marginalised groups, they compound their economic disadvantage. This has significant effects on access to credit, upward mobility, and wealth creation. Economic growth depends on financial inclusion, which means that people and companies can easily and affordably access a variety of financial products and services. When AI-driven loan algorithms unfairly reject borrowers from low-income areas, a divide opens between affluent and impoverished neighbourhoods. For example, minority business owners may face obstacles while trying to get a business loan, which might restrict their capacity to launch or grow their companies and contribute to economic inequality. If biased lending algorithms are used, affected people may be discouraged from seeking loans completely. Some may turn to fraudulent lenders or payday loan companies for higher-interest alternatives if they have a history of bad experiences with traditional lenders, such as high rejection rates or unfavourable loan terms. Preventing Artificial Intelligence Lending Bias Financial organisations, regulators, and researchers have been actively seeking ways to address the issue of biased AI models and encourage fair lending practices. There are a number of ways that AI-driven loan decisions could be made less biased: Enhancing the Accuracy and Transparency of Data Enhancing data and other similar strategies can also aid in achieving a more balanced training sample. This requires creating data points artificially so that minority groups are better represented and the algorithm is not biased against them. Testing for Bias and Fairness in Algorithms Additionally, banks and other financial organizations should limit t
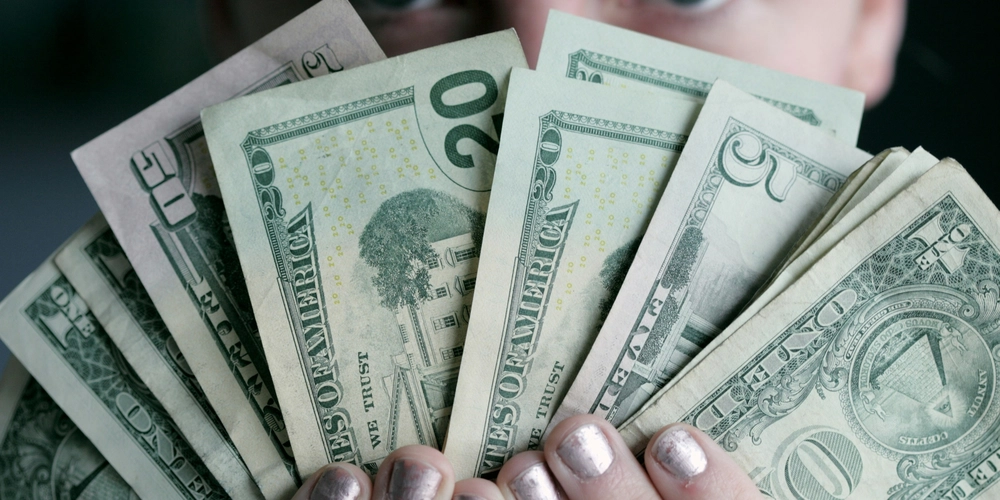
Artificial Intelligence (AI) has transformed numerous industries, including finance. One of its most significant applications in banking and credit services is in the decision-making process for loan approvals. AI-driven computers examine large amounts of financial data to assess a borrower’s creditworthiness, presumably making the process more efficient, accurate, and impartial.
However, concerns regarding prejudice and discrimination in these algorithms generated extensive controversy. The dependence on machine learning (ML) models trained on historical data has, in numerous instances, maintained existing inequalities, disproportionately disadvantaging vulnerable communities.**
*The Role of AI in Loan Decisions *
Traditionally, banks and credit institutions depended on human underwriters to assess an applicant’s creditworthiness based on financial history, income, job position, and other characteristics. This approach was typically time-consuming and prone to human biases. AI has substantially expedited this procedure, evaluating complicated patterns in financial data within seconds to provide loan eligibility scores.
Machine learning algorithms assess risk using enormous datasets, discovering tendencies that might not be immediately obvious to human analysts. By evaluating significant historical data, AI algorithms predict the possibility of a borrower defaulting on a loan. This automation reduces human involvement and is supposed to promote efficiency, minimise errors, and improve risk assessment.
However, AI-based lending systems are not immune to bias despite these advantages. These algorithms inherit prejudices buried in past data, potentially leading to discriminatory lending practices that contribute to economic and social disparity.
*A Problem with AI Loan Algorithms and Bias *
To predict how the financial markets will behave in the future, AI algorithms examine historical data. The issue is that AI models can learn and perpetuate biases if the training data reflects past forms of discrimination, such as redlining, which systematically denied home loans to racial minorities. Numerous studies have shown that minority borrowers are disproportionately affected by the algorithms used by large financial institutions, leading to higher rejection rates and less favourable loan terms for these groups.
Artificial intelligence lending models often include factors that can introduce bias. Indirect indications, such as ZIP codes, educational background, or employment history, might substitute for race, socioeconomic status, or gender even when algorithms don’t include explicit racial or gender data. Therefore, due to historical inequalities, AI models may unintentionally harm some groups.
For instance, even after accounting for creditworthiness, a National Bureau of Economic Research study indicated that mortgage algorithms still charge Black and Hispanic borrowers greater interest rates than White borrowers. This provides more evidence that AI-driven lending algorithms are fundamentally biased.
*The Effects of AI Discrimination on Access to Credit *
Artificial intelligence (AI) bias in lending processes worsens already-existing income disparities. When AI models reject credit applications from marginalised groups, they compound their economic disadvantage. This has significant effects on access to credit, upward mobility, and wealth creation.
Economic growth depends on financial inclusion, which means that people and companies can easily and affordably access a variety of financial products and services. When AI-driven loan algorithms unfairly reject borrowers from low-income areas, a divide opens between affluent and impoverished neighbourhoods. For example, minority business owners may face obstacles while trying to get a business loan, which might restrict their capacity to launch or grow their companies and contribute to economic inequality.
If biased lending algorithms are used, affected people may be discouraged from seeking loans completely. Some may turn to fraudulent lenders or payday loan companies for higher-interest alternatives if they have a history of bad experiences with traditional lenders, such as high rejection rates or unfavourable loan terms.
Preventing Artificial Intelligence Lending Bias
Financial organisations, regulators, and researchers have been actively seeking ways to address the issue of biased AI models and encourage fair lending practices. There are a number of ways that AI-driven loan decisions could be made less biased:
Enhancing the Accuracy and Transparency of Data
Enhancing data and other similar strategies can also aid in achieving a more balanced training sample. This requires creating data points artificially so that minority groups are better represented and the algorithm is not biased against them.
Testing for Bias and Fairness in Algorithms
Additionally, banks and other financial organizations should limit their AI models to be fair. These limitations, which may compromise the accuracy of the model, are necessary to prevent the algorithm from unfairly harming any particular group.
Artificial Intelligence Decisions: Openness and Clarity
Since machine learning models are “black box” in nature, this poses a big problem for AI-driven lending. It is challenging to comprehend the decision-making process of many AI algorithms since they function like complex systems. Recognising and addressing biases becomes more difficult due to this lack of openness.
*Frameworks for Regulation and Law *
Artificial intelligence lending models should be made more open by lawmakers who should make financial institutions publish the decision-making processes of their algorithms and require them to do bias evaluations. In addition, accusations about biased AI choices can be addressed by establishing independent monitoring groups that check compliance.
The Evolution of Moral AI
Financial institutions should prioritise ethics when creating and implementing AI lending models. They must ensure that AI systems adhere to the ideals of honesty, openness, and responsibility. To this end, financial institutions should form internal ethical committees to monitor artificial intelligence research and evaluate how lending algorithms affect various demographics.
To further create AI systems that promote financial inclusion while reducing harm, collaborative efforts among data scientists, ethicists, legislators, and community activists are necessary to change the financial sector for the better. It must be used wisely. By actively attempting to eradicate bias from AI lending models, we can create a more inclusive financial system in which credit is granted based on fair and unbiased criteria rather than previous preconceptions.