Rethinking AI in Security
Ambiguity, Confidence, and What Trust Actually Looks Like “AI should augment analysts, not replace them.” That phrase shows up everywhere. It sounds right. It feels responsible. But if we’ve all agreed on that, why are so many systems still nudging humans into quiet compliance? The problem isn’t just in implementation. It’s in our assumptions. AI in security is still being built around outdated ideas of intelligence and risk. Systems are optimized for output, not dialogue. Precision, not process. If augmentation is the goal, we have to rethink how systems support the actual work of being uncertain. Support means leaving room for ambiguity Security analysts don’t just follow playbooks. They work in gray areas. They ask hard questions. The tools we give them should make that easier, not harder. But most AI systems are trained on statistical regularities. They compress nuance into confidence. Even when the evidence is murky, they present clean answers. That makes them brittle. It makes them misleading. A better system wouldn’t flatten ambiguity. It would surface it. This is where concepts like epistemic uncertainty and aleatoric uncertainty matter. A system should know the difference between what it hasn’t seen enough of and what is simply noisy by nature. That’s the difference between “I don’t know yet” and “it’s hard to know.” Bayesian deep learning, Monte Carlo dropout, and evidential deep learning all offer paths toward calibrated uncertainty. These methods help AI say something human: "I’m not sure." That matters. Because when humans aren’t sure, we dig deeper. We escalate. We talk. But when AI doesn’t show doubt, analysts often assume it’s right. Even when it isn’t. What makes data meaningful? Luciano Floridi argues that meaning can’t be reduced to raw correlation. We don’t just need data to match. We need it to matter. We need AI systems to help analysts build meaning, not just spot patterns. Here’s one way to check your tooling: If an analyst can’t ask, “How did you get there?” and get an intelligible answer, your AI isn’t a partner. It’s a guess engine. In real life One system flagged a developer’s login as suspicious due to “unusual access timing.” That might sound solid. But it didn’t know the dev was traveling internationally. The analyst did. No escalation. No breach. But the system stayed confident. That’s flattened ambiguity. And if the analyst had trusted it blindly, it might’ve escalated a false positive... or worse, missed something that actually mattered. What Trust Actually Looks Like in AI-Augmented Security If we want AI to truly support analysts, we need systems that don’t just spit out conclusions but know how to ask better questions. That starts with something most AI still struggles with: uncertainty. High confidence doesn’t always mean accuracy. Models trained on limited historical data can sound sure but miss the mark because they’re working with a narrow slice of reality. This is where Bayesian deep learning and Monte Carlo dropout come in. They help models express uncertainty, not just results. That doesn’t make AI weaker. It makes it more honest. Security work is full of ambiguity. Sometimes even a human wouldn’t call something cleanly malicious or benign. When an AI forces a yes-or-no without room for doubt, it’s not helping. It’s brittle. We need systems that can say “I’m not sure” or flag uncertainty. That doesn’t make them less valuable. It makes them safer. Explainability Isn’t a Bonus. It’s the Job. When analysts can’t see how a decision was made, trust breaks down. This is where explainability techniques like SHAP and LIME matter. They help make model behavior more transparent by showing which inputs mattered most. Attention heatmaps can reveal what the model focused on when it made a call. That kind of visibility gives analysts a way to validate or challenge the logic. Augmentation should be about clarity, not black boxes. What Privacy Looks Like When Context Matters Privacy isn’t a checklist. It’s contextual. Helen Nissenbaum, a philosopher who writes about data ethics, introduced the idea of contextual integrity. Privacy isn’t just about who has access. It’s about whether that access makes sense in the moment, based on the expectations of the environment. Most AI systems treat data as open once collected. But if a detection system pulls from HR data to flag an insider threat, should that info be used later to prioritize a support ticket? Maybe not. That’s a privacy failure wrapped in a security feature. Better Conversations, Not Just Better Predictions At the end of the day, a good AI system doesn’t just tell you something. It invites collaboration. That’s what reinforcement learning with human feedback (RLHF) is built to support. Systems that improve by being questioned. But even that goes wrong if the model starts optimizing for the wrong signals (reward hacking) or
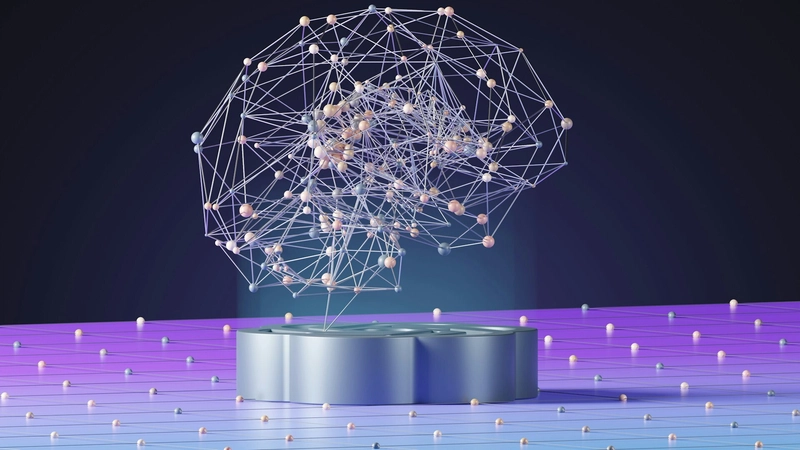
Ambiguity, Confidence, and What Trust Actually Looks Like
“AI should augment analysts, not replace them.”
That phrase shows up everywhere. It sounds right. It feels responsible. But if we’ve all agreed on that, why are so many systems still nudging humans into quiet compliance?
The problem isn’t just in implementation. It’s in our assumptions. AI in security is still being built around outdated ideas of intelligence and risk. Systems are optimized for output, not dialogue. Precision, not process.
If augmentation is the goal, we have to rethink how systems support the actual work of being uncertain.
Support means leaving room for ambiguity
Security analysts don’t just follow playbooks. They work in gray areas. They ask hard questions. The tools we give them should make that easier, not harder.
But most AI systems are trained on statistical regularities. They compress nuance into confidence. Even when the evidence is murky, they present clean answers. That makes them brittle. It makes them misleading.
A better system wouldn’t flatten ambiguity. It would surface it.
This is where concepts like epistemic uncertainty and aleatoric uncertainty matter. A system should know the difference between what it hasn’t seen enough of and what is simply noisy by nature. That’s the difference between “I don’t know yet” and “it’s hard to know.”
Bayesian deep learning, Monte Carlo dropout, and evidential deep learning all offer paths toward calibrated uncertainty. These methods help AI say something human:
"I’m not sure."
That matters. Because when humans aren’t sure, we dig deeper. We escalate. We talk. But when AI doesn’t show doubt, analysts often assume it’s right. Even when it isn’t.
What makes data meaningful?
Luciano Floridi argues that meaning can’t be reduced to raw correlation. We don’t just need data to match. We need it to matter. We need AI systems to help analysts build meaning, not just spot patterns.
Here’s one way to check your tooling:
If an analyst can’t ask, “How did you get there?” and get an intelligible answer, your AI isn’t a partner. It’s a guess engine.
In real life
One system flagged a developer’s login as suspicious due to “unusual access timing.” That might sound solid. But it didn’t know the dev was traveling internationally. The analyst did.
No escalation. No breach.
But the system stayed confident.
That’s flattened ambiguity. And if the analyst had trusted it blindly, it might’ve escalated a false positive... or worse, missed something that actually mattered.
What Trust Actually Looks Like in AI-Augmented Security
If we want AI to truly support analysts, we need systems that don’t just spit out conclusions but know how to ask better questions. That starts with something most AI still struggles with: uncertainty.
High confidence doesn’t always mean accuracy. Models trained on limited historical data can sound sure but miss the mark because they’re working with a narrow slice of reality. This is where Bayesian deep learning and Monte Carlo dropout come in. They help models express uncertainty, not just results. That doesn’t make AI weaker. It makes it more honest.
Security work is full of ambiguity. Sometimes even a human wouldn’t call something cleanly malicious or benign. When an AI forces a yes-or-no without room for doubt, it’s not helping. It’s brittle.
We need systems that can say “I’m not sure” or flag uncertainty. That doesn’t make them less valuable. It makes them safer.
Explainability Isn’t a Bonus. It’s the Job.
When analysts can’t see how a decision was made, trust breaks down. This is where explainability techniques like SHAP and LIME matter. They help make model behavior more transparent by showing which inputs mattered most. Attention heatmaps can reveal what the model focused on when it made a call. That kind of visibility gives analysts a way to validate or challenge the logic.
Augmentation should be about clarity, not black boxes.
What Privacy Looks Like When Context Matters
Privacy isn’t a checklist. It’s contextual. Helen Nissenbaum, a philosopher who writes about data ethics, introduced the idea of contextual integrity. Privacy isn’t just about who has access. It’s about whether that access makes sense in the moment, based on the expectations of the environment.
Most AI systems treat data as open once collected. But if a detection system pulls from HR data to flag an insider threat, should that info be used later to prioritize a support ticket? Maybe not. That’s a privacy failure wrapped in a security feature.
Better Conversations, Not Just Better Predictions
At the end of the day, a good AI system doesn’t just tell you something. It invites collaboration. That’s what reinforcement learning with human feedback (RLHF) is built to support. Systems that improve by being questioned. But even that goes wrong if the model starts optimizing for the wrong signals (reward hacking) or drifts out of alignment with real-world conditions (concept drift).
None of this should be left on autopilot.
The best AI in security won’t just be accurate. It’ll be interactive. It’ll know when it’s guessing. It’ll explain itself clearly. And it will respect that human judgment isn’t a fallback. It’s the foundation of what makes security work.
Here you go! Here’s a clean Sources & Further Reading section formatted for your dev.to post:
⸻
Sources & Further Reading
- Gal, Y., & Ghahramani, Z. (2016). Dropout as a Bayesian Approximation: Representing Model Uncertainty in Deep Learning. arXiv:1506.02142
- Nissenbaum, H. (2004). Privacy as Contextual Integrity. Washington Law Review
- Nguyen, A., Yosinski, J., & Clune, J. (2015). Deep Neural Networks are Easily Fooled: High Confidence Predictions for Unrecognizable Images. CVPR 2015
- Ribeiro, M. T., Singh, S., & Guestrin, C. (2016). "Why Should I Trust You?": Explaining the Predictions of Any Classifier. arXiv:1602.04938
- Lundberg, S. M., & Lee, S. I. (2017). A Unified Approach to Interpreting Model Predictions (SHAP). NeurIPS 2017
- Amodei, D. et al. (2016). Concrete Problems in AI Safety. arXiv:1606.06565
- Bostrom, N., & Yudkowsky, E. (2014). The Ethics of Artificial Intelligence. Cambridge Handbook of Artificial Intelligence
- Floridi, L. (2010). Information: A Very Short Introduction. Oxford University Press
- CrowdStrike (2024). AI in Cybersecurity Survey Report. CrowdStrike Blog
- SecurityWeek (2024). AI in the SOC: Why Human Oversight Still Matters. SecurityWeek Article